Leveraging Kernelized Synergies on Shared Subspace for Precision Grasping and Dexterous Manipulation
IEEE TRANSACTIONS ON COGNITIVE AND DEVELOPMENTAL SYSTEMS(2023)
摘要
Manipulation in contrast to grasping is a trajectorial task that needs to use dexterous hands. Improving the dexterity of robot hands increases the controller complexity and thus requires to use the concept of postural synergies. Inspired from postural synergies, this research proposes a new framework called kernelized synergies that focuses on the reusability of the same subspace for precision grasping and dexterous manipulation. In this work, the computed subspace of postural synergies is parameterized by kernelized movement primitives (KMPs) to preserve its grasping and manipulation characteristics and allows its reuse for new objects. The grasp stability of the proposed framework is assessed with the force closure quality index, as a cost function. For performance evaluation, the proposed framework is initially tested on two different simulated robot hand models using the Syngrasp toolbox and experimentally, four complex grasping and manipulation tasks are performed and reported. Results confirm the hand agnostic approach of the proposed framework and its generalization to distinct objects irrespective of their dimensions.
更多查看译文
关键词
Anthropomorphic hands,dexterous manipulation,kernel trick,probabilistic learning,postural synergies
AI 理解论文
溯源树
样例
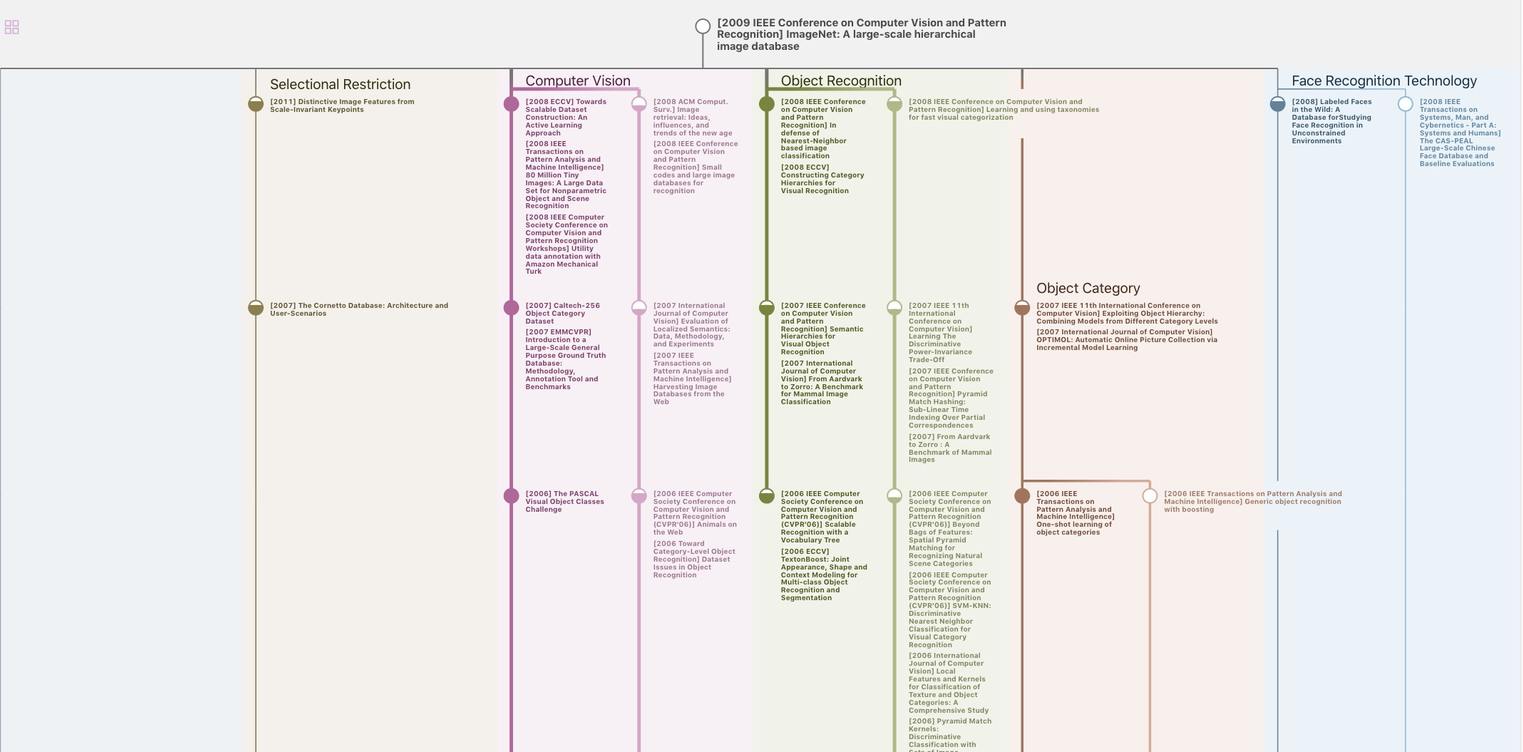
生成溯源树,研究论文发展脉络
Chat Paper
正在生成论文摘要