Multi-Scale Interaction for Real-Time LiDAR Data Segmentation on an Embedded Platform
IEEE Robotics and Automation Letters(2022)
摘要
Real-time semantic segmentation of LiDAR data is crucial for autonomously driving vehicles and robots, which are usually equipped with an embedded platform and have limited computational resources. Approaches that operate directly on the point cloud use complex spatial aggregation operations, which are very expensive and difficult to deploy on embedded platforms. As an alternative, projection-based methods are more efficient and can run on embedded hardware. However, current projection-based methods either have a low accuracy or require millions of parameters. In this letter, we therefore propose a projection-based method, called Multi-scale Interaction Network (MINet), which is very efficient and accurate. The network uses multiple paths with different scales and balances the computational resources between the scales. Additional dense interactions between the scales avoid redundant computations and make the network highly efficient. The proposed network outperforms point-based, image-based, and projection-based methods in terms of accuracy, number of parameters, and runtime. Moreover, the network processes more than 24 scans, captured by a high-resolution LiDAR sensor with 64 beams, per second on an embedded platform, which is higher than the framerate of the sensor. The network is therefore suitable for robotics applications.
更多查看译文
关键词
Autonomous vehicles,image segmentation,laser
AI 理解论文
溯源树
样例
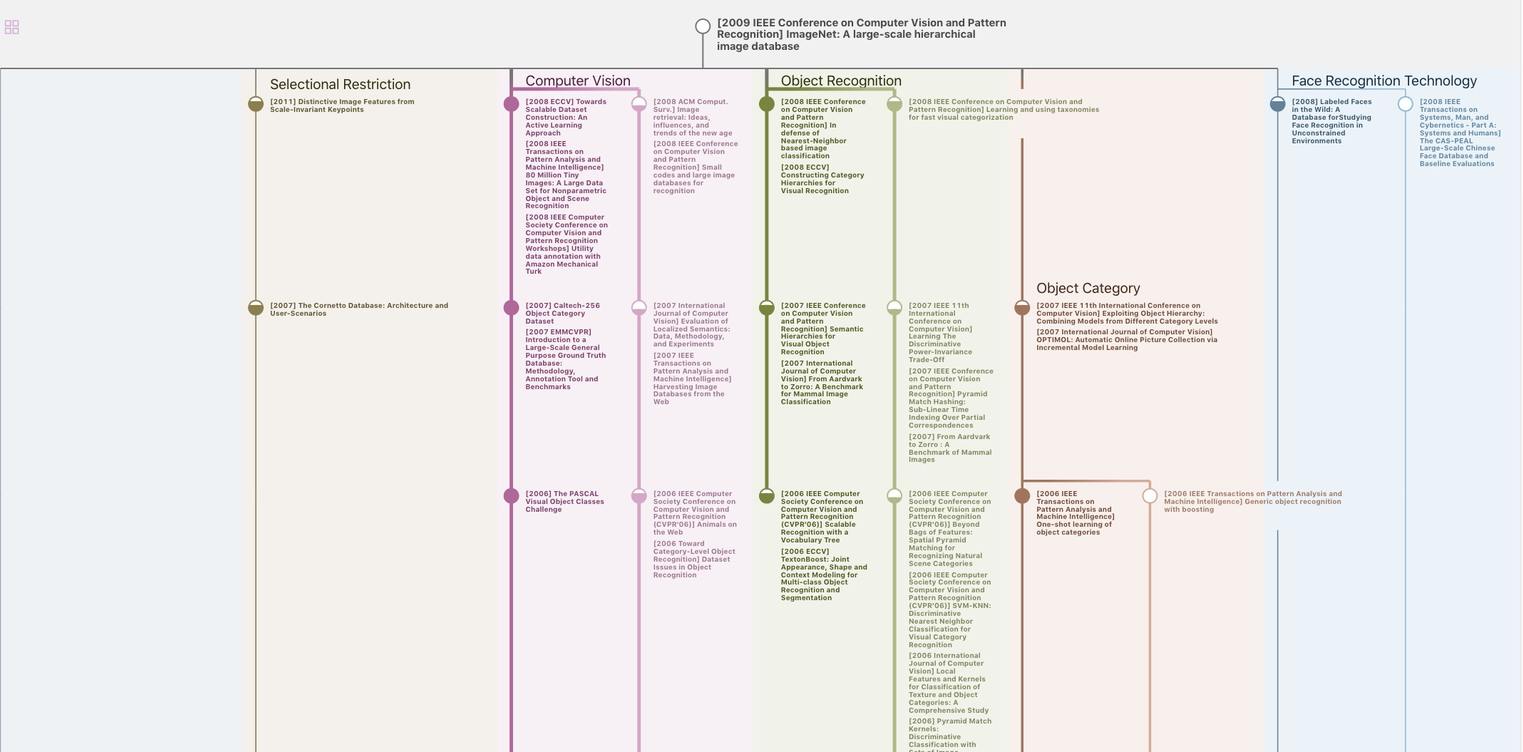
生成溯源树,研究论文发展脉络
Chat Paper
正在生成论文摘要