Causal Integration Of Multi-Omics Data With Prior Knowledge To Generate Mechanistic Hypotheses
bioRxiv(2021)
摘要
Multi-omics datasets can provide molecular insights beyond the sum of individual omics. Various tools have been recently developed to integrate such datasets, but there are limited strategies to systematically extract mechanistic hypotheses from them. Here, we present COSMOS (Causal Oriented Search of Multi-Omics Space), a method that integrates phosphoproteomics, transcriptomics, and metabolomics datasets. COSMOS combines extensive prior knowledge of signaling, metabolic, and gene regulatory networks with computational methods to estimate activities of transcription factors and kinases as well as network-level causal reasoning. COSMOS provides mechanistic hypotheses for experimental observations across multi-omics datasets. We applied COSMOS to a dataset comprising transcriptomics, phosphoproteomics, and metabolomics data from healthy and cancerous tissue from eleven clear cell renal cell carcinoma (ccRCC) patients. COSMOS was able to capture relevant crosstalks within and between multiple omics layers, such as known ccRCC drug targets. We expect that our freely available method will be broadly useful to extract mechanistic insights from multi-omics studies.
更多查看译文
关键词
causal reasoning, kidney cancer, metabolism, multi-omics, signaling
AI 理解论文
溯源树
样例
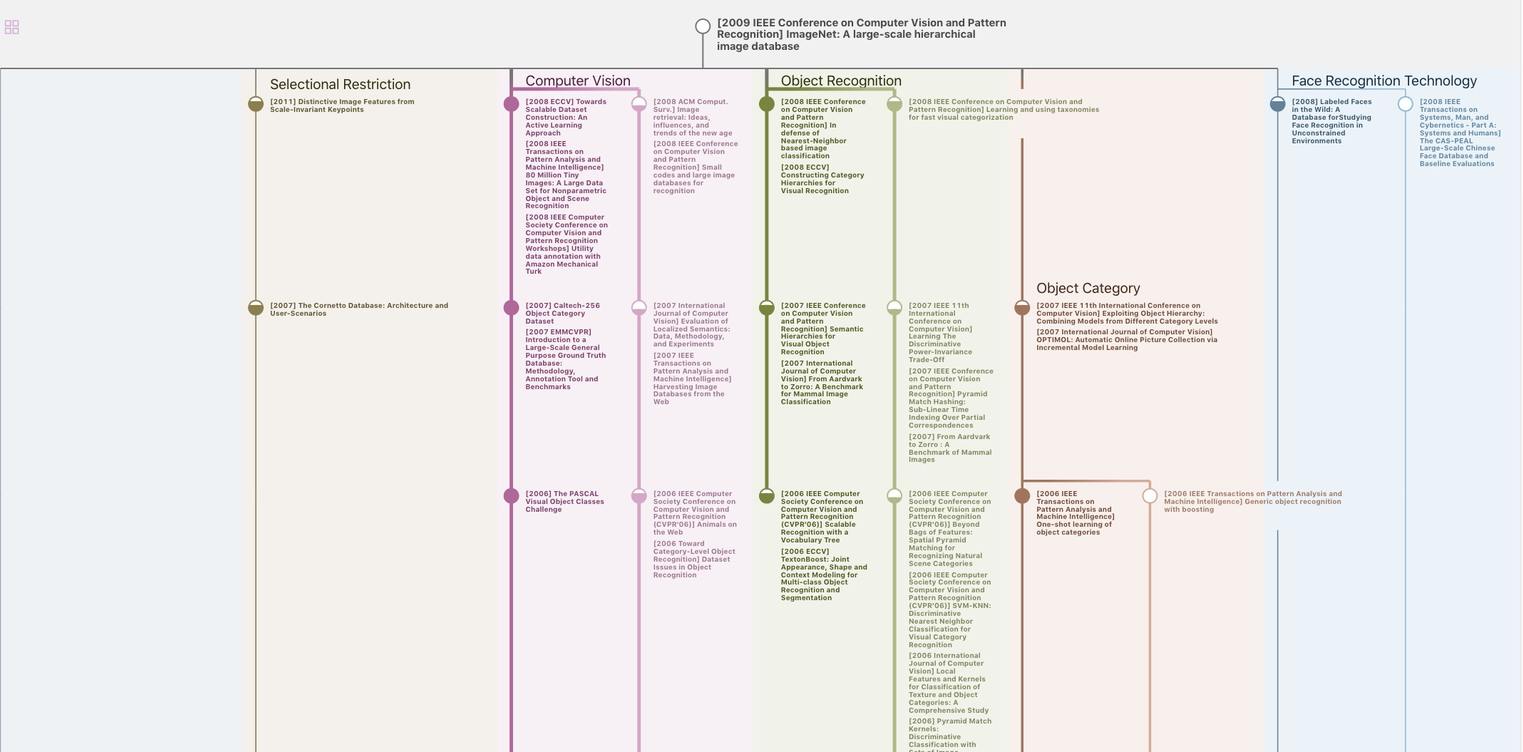
生成溯源树,研究论文发展脉络
Chat Paper
正在生成论文摘要