Self-supervised Learning for Deep Models in Recommendations
arxiv(2020)
摘要
Large scale neural recommender models play a critical role in modern search and recommendation systems. To model large-vocab sparse categorical features, typical recommender models learn a joint embedding space for both queries and items. With millions to billions of items to choose from, the quality of learned embedding representations is crucial to provide high quality recommendations to users with various interests. Inspired by the recent success in self-supervised representation learning research in both computer vision and natural language understanding, we propose a multi-task self-supervised learning (SSL) framework for sparse neural models in recommendations. Furthermore, we propose two highly generalizable self-supervised learning tasks: (i) Feature Masking (FM) and (ii) Feature Dropout (FD) within the proposed SSL framework. We evaluate our framework using two large-scale datasets with ~500M and 1B training examples respectively. Our results demonstrate that the proposed framework outperforms baseline models and state-of-the-art spread-out regularization techniques in the context of retrieval. The SSL framework shows larger improvement with less supervision compared to the counterparts.
更多查看译文
关键词
deep models,recommendations,learning,self-supervised
AI 理解论文
溯源树
样例
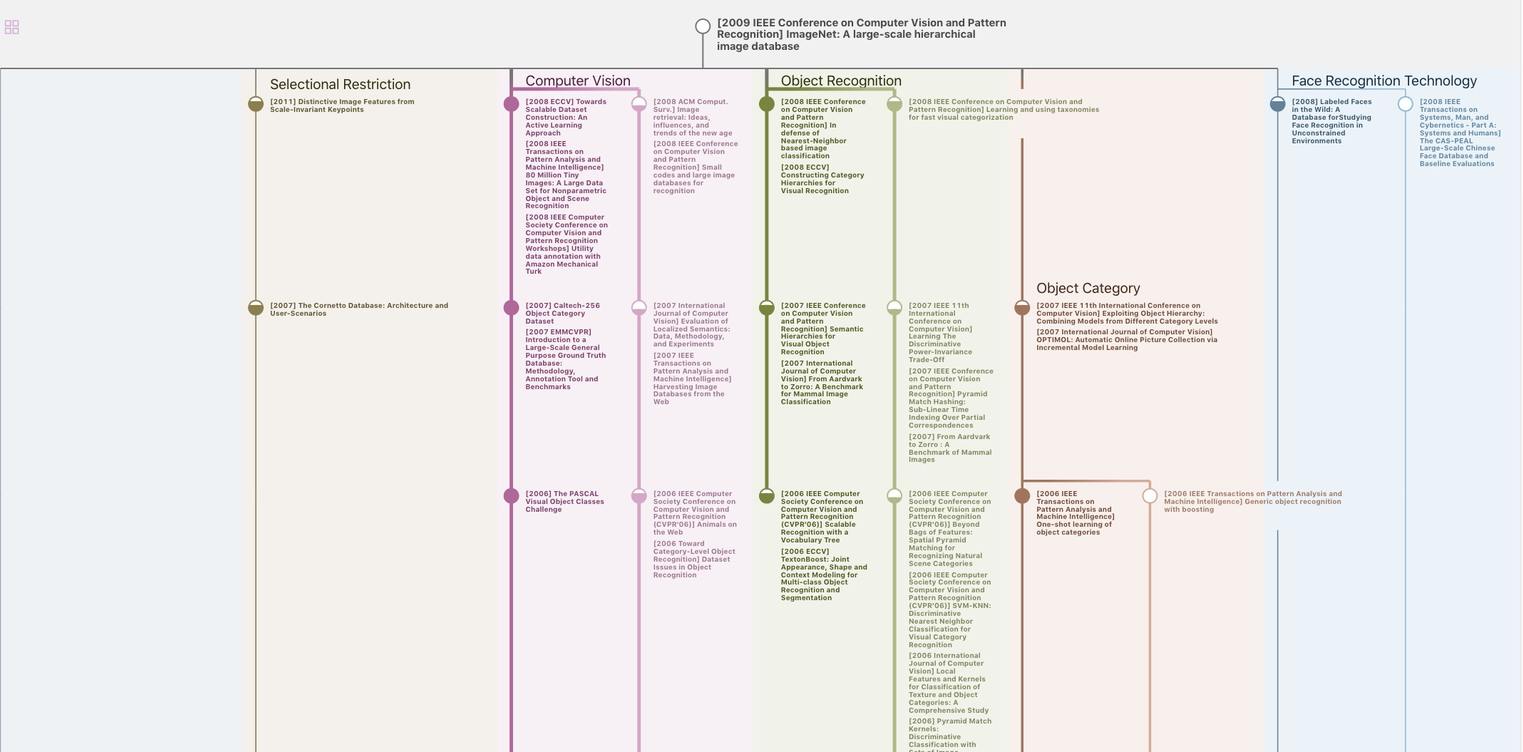
生成溯源树,研究论文发展脉络
Chat Paper
正在生成论文摘要