Improving Student-System Interaction Through Data-driven Explanations of Hierarchical Reinforcement Learning Induced Pedagogical Policies
UMAP '20: 28th ACM Conference on User Modeling, Adaptation and Personalization Genoa Italy July, 2020(2020)
摘要
Motivated by the recent advances of reinforcement learning and the traditional grounded Self Determination Theory (SDT), we explored the impact of hierarchical reinforcement learning (HRL) induced pedagogical policies and data-driven explanations of the HRL-induced policies on student experience in an Intelligent Tutoring System (ITS). We explored their impacts first independently and then jointly. Overall our results showed that 1) the HRL induced policies could significantly improve students' learning performance, and 2) explaining the tutor's decisions to students through data-driven explanations could improve the student-system interaction in terms of students' engagement and autonomy.
更多查看译文
关键词
Hierarchical Reinforcement Learning, Pedagogical Policy, Data-driven Explanations, Intelligent Tutoring System
AI 理解论文
溯源树
样例
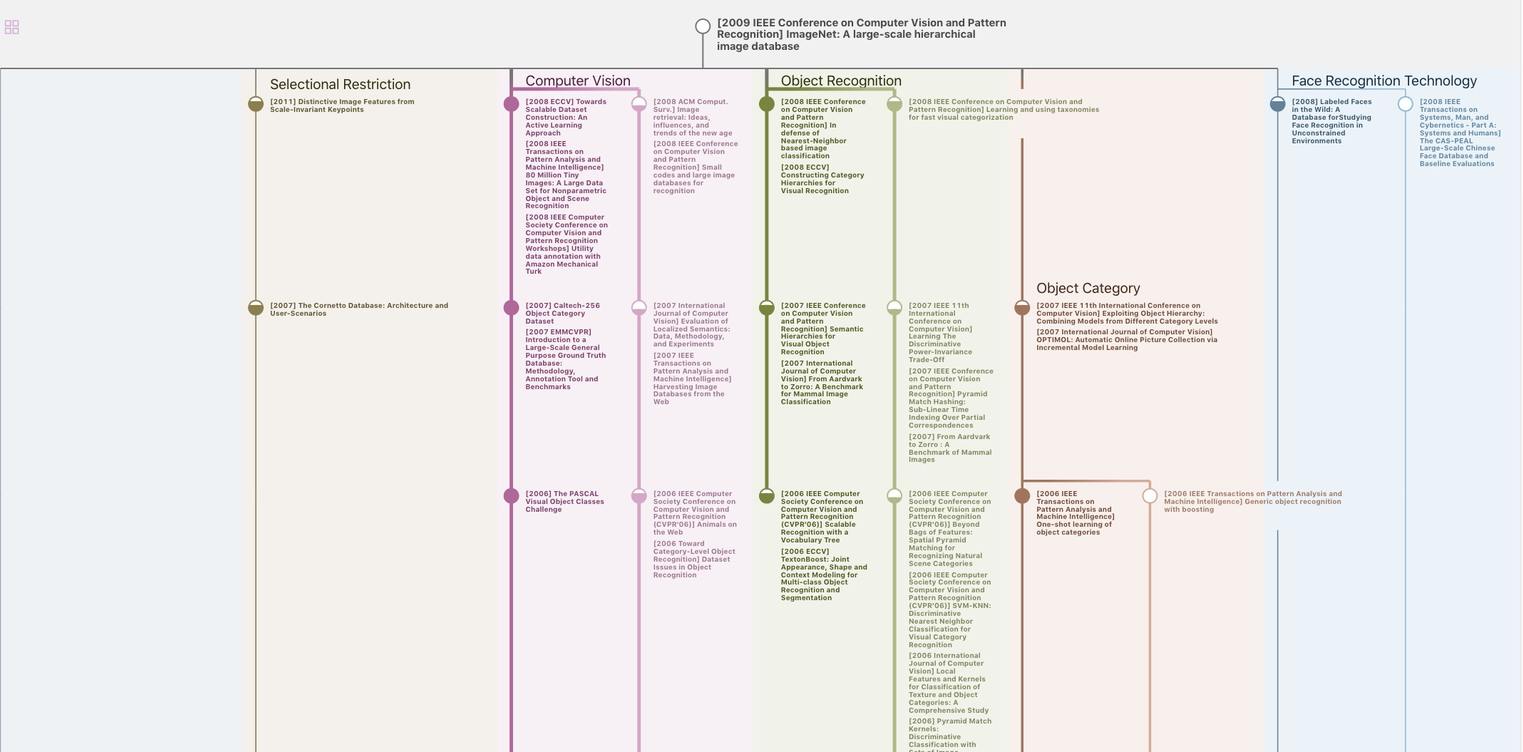
生成溯源树,研究论文发展脉络
Chat Paper
正在生成论文摘要