Session 1A: Global Agricultural Modeling for development and climate analysis #1
semanticscholar(2018)
摘要
Managing shared data is becoming increasingly important as we move towards an open data world. For sharable data to be actionable, it needs to be FAIR: findable, accessible, interoperable and re-usable. In applied interdisciplinary research, data from many different sources are used. Socio-economic data is often referred to as being of relatively low quality. In part, this is due to a lack of standardization. Socio-economic data is messy. Data sets tend to be a mix of structured semi-structured, and unstructured data. The community of practice on socio-economic data (#CoP_SED) , which is part of the CGIAR Platform for Big Data in Agriculture aims at providing the tools to make socioeconomic data FAIR. In this presentation, we will address some of the latest developments in managing actionable open data, touching on issues such as ontologies, metadata schemas, key indicators, data curation and confidentiality. This is ongoing work, which has the potential of revolutionizing the way we use socio-economic data. Title: Shared protocols and data template in agronomic trials Presenter: Davide Cammarano Author: Cammarano, Davide, Drexler, Dora, Martre, Pierre, Cooper, Julia, Drayer, Xavier, Helga, Willer, Pecchioni, Nicola, Sessitch, Angela, Voicu, Adriana, Hinsinger, Philippe 1 James Hutton Institute 2 OMKI, Hungarian Research Institute of Organic Agriculture 3 INRA Montpellier 4 University of Newcastle 5 Universite' Catholique de Louvain (UCL) 6 FiBL Switzerland 7 Centre for Cereal Research 8 Austrian Institute of Technology (AIT) Abstract: The aim of the study is to show how a H2020-Funded project is proposing to tackle the issue of data standardization and shared protocols among 25 partners. The project SolACE (Solutions for improving Agroecosystem and Crop Efficiency for water and nutrient use) is proposing to create a common data dictionary for all the data-collection activities among partners. The data dictionary contains the header of the variables, the units in which will be reported, and the definition. An existing data dictionary (ICASA Data Standard) has been used as baseline. But, additional variables can be added if they are not in the dictionary. In addition, a common set of templates has been created and shared among partners so that all the agronomic trials will be reported in a same "textdelimited" file. Its structure is flexible because it should allow users to add only the variable they measure in the field. But, if they are reported according to our dictionary it will facilitate data analysis and the creation of tools that will help to visualize data. Finally, a "living" handbook of protocols is shared among partners with the aim of clearly report what steps and methodology have been taken in order to collect the data. Title: AgMIP Data Interoperability: Moving beyondRegional Integrated Assessments Presenter: Cheryl Porter Author: Martre Porter, Cheryl, Villalobos, Chris, White, Jeff, Hoogenboom, Gerrit, Devare, Medha 1 University of Florida 2 University of Florida 3 USDA-ARS 4 University of Florida 5 CGIAR Consortium Abstract: AgMIP promotes comparisons among cropping system models through use of common datasets. Interoperability tools were developed to allow multiple crop models to access consistent input data and to harmonize model outputs regardless of internal model requirements. AgMIP use cases for data interoperability tools include the DFIDfundedRegional Integrated Assessments activities in Sub-Saharan Africa and South Asia, the wheat model intercomparison activities, and the PSIMs gridded modeling framework. The AgMIP data interoperability tools are increasingly being used by non-AgMIP researchers, who recognize the need for harmonizing agricultural data from diverse sources for use in quantitative analyses. Applications include CGIAR CCAFS Regional Agricultural Forecast Tool (CRAFT), S-World global soil mapping software, DOE Terra project, and direct use as input to crop models. Additionally, the ICASA Data Dictionary has been aligned with the CGIAR Crop and Agronomy ontologies to facilitate the future use of semantic technologies for discovery and access of data relevant to modeling and other quantitative analyses. We have found that it is difficult to balance the use of scarce resources to solve the immediate needs of users for data curation and interoperability tools versus dealing with longer-term development of robust frameworks based on ontologies and semantic technologies for data discovery and access. The AgMIP community of users shared an immediate demand for data interoperability tools, which was most readily satisfied by building on the existing ICASA Data Dictionary. The existing system is flexible, efficient, extensible, and forms a solid foundation for next generation data interoperability systems. Title: Mobile phone based advisories for smallholder farmers; lessons from the field Presenter: Peter Craufurd Author: Craufurd, Peter and Andersson, Jens 1 CIMMYT Abstract: The use of Information and communications technologies (ICT) for knowledge sharing, and especially advice via decision-support tools (DST) on mobile devices, is frequently proposed as a means to improve small-holder farming. Mobile phones are also a potentially useful source of spatial and temporal data on variables that are hard to collect remotely, such as yield and prices, i.e.crowd-sourcing. In TAMASA (Taking Maize Agronomy to Scale in Africa) we have been evaluating, with a range of different users, mobile-phone based decision support tools for maize crop. Stakeholders, both individuals and more importantly service providers, commonly demand information on weather (when to plant, risk of drought), fertiliser use (what type, rate and timing), variety selection, and pest and disease advisories. We also observe that most smallholder maize farmers do not plant or achieve anywhere near optimal plant population densities. We have therefore been co-developing and evaluating with users DSTs for nutrient management, variety selection, planting date, and seed rate/ plant population density. This presentation will share many of the lessons we have learnt and question whether this is the panacea many believe. Key lessons include: the absolute importance of the user-interface (UI) and the experience for the user; enabling advice to be actioned; and institutionalisation of the app and background analytics. Title: Evolving the AgMIP Impacts Explorer Presenter: Sander Janssen Author: Sander Janssen, Alex Ruane, Roberto Valdivia, Senthold Asseng, John Antle, Cheryl Porter, Carolyn Mutter, Joske Hootkamp Abstract: The AgMIP Impacts Explorer is a web-based tool, developed to make the outcomes of AgMIP Phase 2 regional studies accessible for use in policy and research. The Impacts Explorer allows a variety of audiences to explore future projections of risk and vulnerabilities in the agricultural sector, implications of food security, and adaptation options, through three components: (1) a descriptive overview of main outcomes of the regional studies; (2) a dashboard with infographics of key findings for comparing results across regions and systems; and (3) an interactive Data Exploration Tool, providing access to model outputs and economic evaluations underlying the analysis. Because of the modular design and the utility of the components, the Impacts Explorer can be extended to include results of other research projects, offering an easy to use platform for dissemination. Moreover, the tool makes results comparable along different dimensions such as regions or farming systems, substantially augmenting the value of the individual studies. This paper presents use cases illustrating this potential of the Impacts Explorer; and discusses possible modifications of the platform, and data requirements for participating projects. Back to Top Session 2C: Data Assimilation and Seasonal Forecasting of Agricultural Shocks Title: Crop Yield Predictions Multi-scale Statistical Model for Intra-season Forecasts Applied to Corn in the US Presenter: Yiqing Cai Author: Cai, Yiqing , Moore, Kristen , Pellegrini, Adam , Elhaddad, Aymn , Lessel Jerrod , Townsend Christianna , Solak Hayley , Zhao Yi , Semret, Nemo , 1 Gro Intelligence, Inc Abstract: Accurately forecasting crop yields has broad implications for economic trading, food production monitoring, and global food security. However, the lack of highly accurate environmental measurements makes it difficult to capture the variation of yield across space and time. To maximize the information gain from the available data, we developed a sequence of hierarchical machine-learning based models accurately forecasting end-of-season corn yields for the US at both the county and national levels, including in very anomalous seasons. Furthermore, we incorporated crop physiological processes in our model’s feature selection. Our model has run live since 2016. In 2017, our forecast was in the correct direction (above trend) ahead of the market and USDA’s forecast since mid August, and was already within 2% of the USDA January forecast since mid September. In the backtesting of the 2000-2016 period, our model predicts national yield within 2.55% of the actual yield on average already by mid-August. At the county level, our model predicts 80% of the variation in final yield by the beginning of October, with 77% counties predicted within 10% of the average yield. Further, the most significant producing regions had the lowest error rates, resulting in our high precision national-level forecasts. In addition, we identify the changes of important variables throughout the season, specifically early-season land surface temperature, and mid-season land surface temperature and vegetation index. As the first example of Gro yield model framework, this can be extended to other crops and regions with consistent performance evaluation. Title: The Agricultural Productivity Indicator Analysis System (APIAS) Presenter:
更多查看译文
AI 理解论文
溯源树
样例
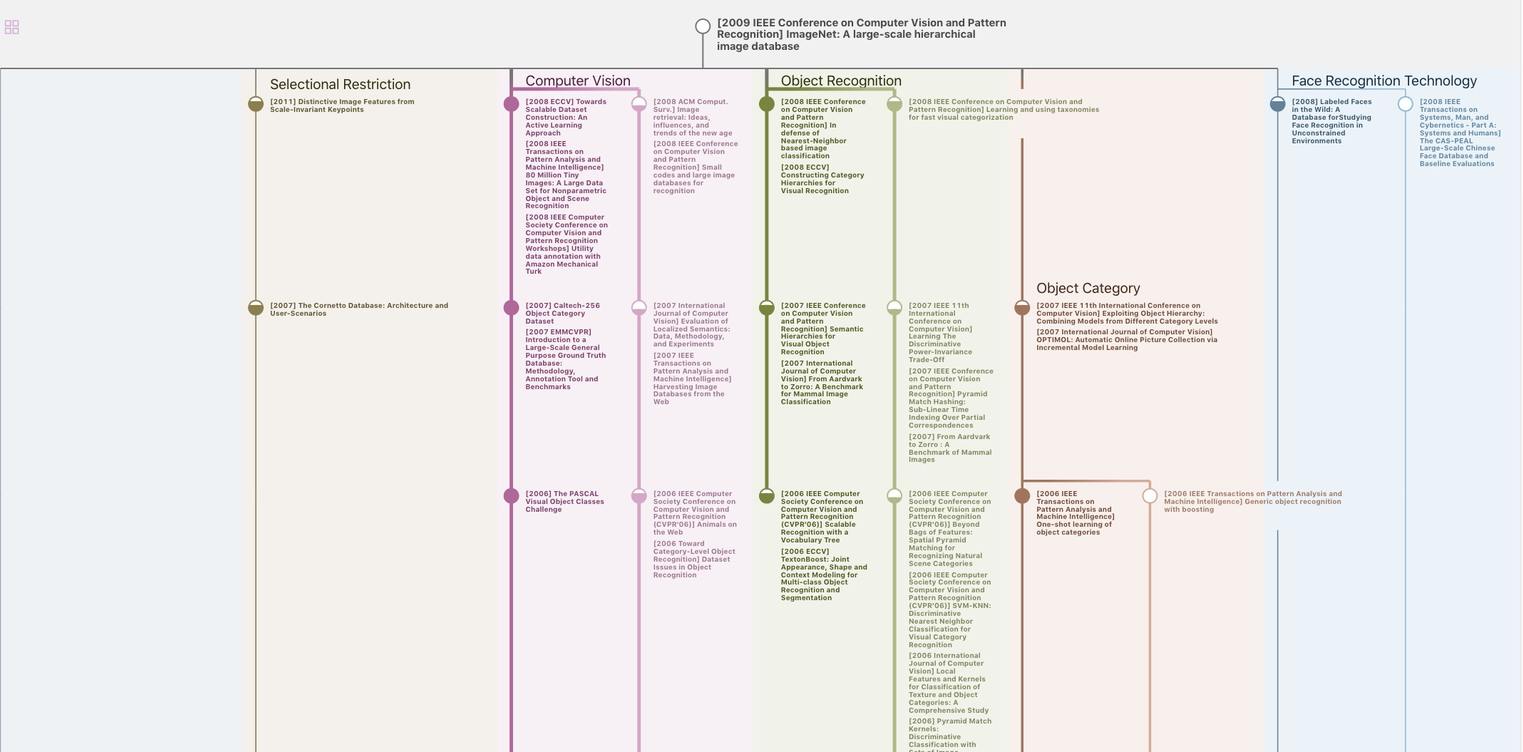
生成溯源树,研究论文发展脉络
Chat Paper
正在生成论文摘要