Helpfulness-aware review based neural recommendation
CCF Transactions on Pervasive Computing and Interaction(2019)
摘要
Reviews contain rich information of user interests and item characteristics. Incorporating reviews into recommendation has attracted increasing attention in recent years, which can help learn more accurate user and item representations for recommendation. Existing review based recommendation methods usually utilize the content of reviews while ignoring the helpfulness scores associated with them. Since different reviews have different informativeness and many reviews are noisy and even misleading, incorporating the helpfulness information of reviews can help better exploit the reviews for recommendation. In this paper, we propose a helpfulness-aware review based recommendation approach. The core of our approach is a review encoder and a user/item encoder. In the review encoder we learn representations of reviews from their content in a hierarchical way. We first learn sentence representations from words and then learn review representations from sentences, using a hierarchical attention network to select important words and sentences. In the user/item encoder, we learn representations of users/items from their reviews using an attention network. The query vector of the attention network comes from the helpfulness scores of these reviews. Since many reviews do not have helpfulness scores, we propose a neural helpfulness prediction model to predict the helpfulness scores of these reviews. The neural helpfulness prediction model is trained on the limited reviews with helpfulness scores voted by users. Extensive experiments on four benchmark datasets show that incorporating the helpfulness of reviews can effectively improve the performances of review based neural recommendation methods.
更多查看译文
关键词
Recommender systems, Review helpfulness, Hierarchical attention network
AI 理解论文
溯源树
样例
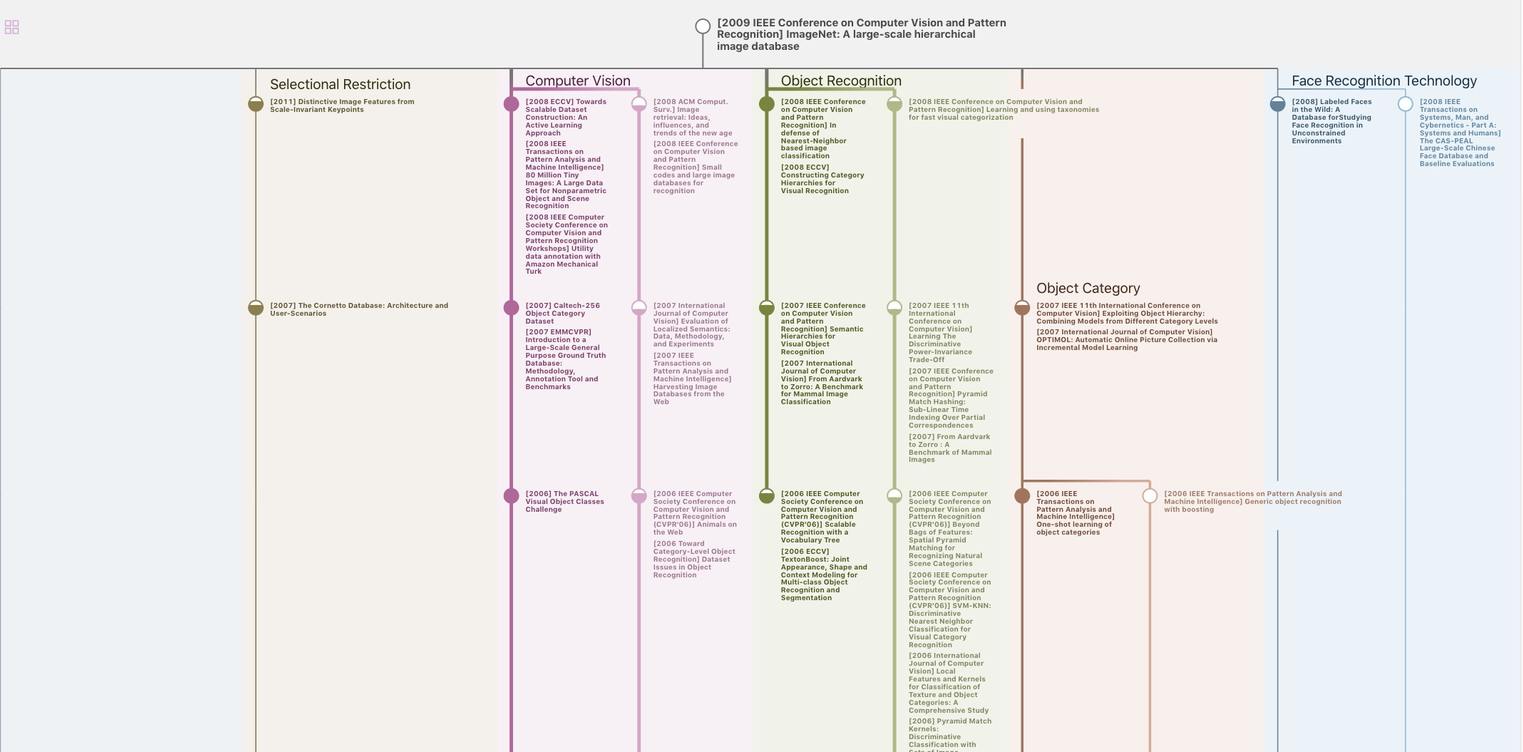
生成溯源树,研究论文发展脉络
Chat Paper
正在生成论文摘要