Learning compositional functions via multiplicative weight updates
NIPS 2020(2020)
摘要
Compositionality is a basic structural feature of both biological and artificial neural networks. Learning compositional functions via gradient descent incurs well known problems like vanishing and exploding gradients, making careful learning rate tuning essential for real-world applications. This paper proves that multiplicative weight updates satisfy a descent lemma tailored to compositional functions. Based on this lemma, we derive Madam – a multiplicative version of the Adam optimiser – and show that it can train state of the art neural network architectures without learning rate tuning. We further show that Madam is easily adapted to train natively compressed neural networks by representing their weights in a logarithmic number system. We conclude by drawing connections between multiplicative weight updates and recent findings about synapses in biology.
更多查看译文
关键词
compositional functions,learning
AI 理解论文
溯源树
样例
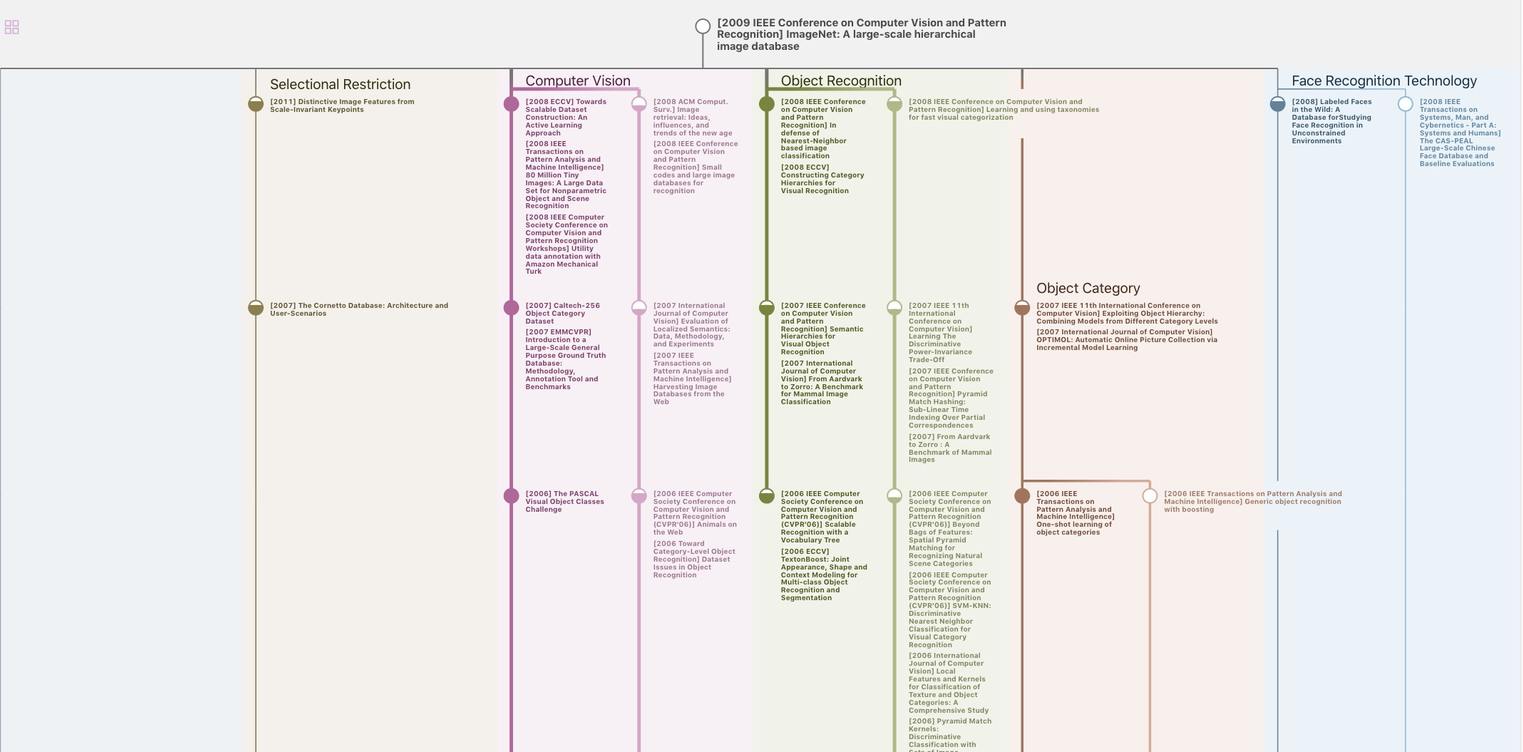
生成溯源树,研究论文发展脉络
Chat Paper
正在生成论文摘要