Automated tuning of query degree of parallelism via machine learning
SIGMOD/PODS '20: International Conference on Management of Data Portland Oregon June, 2020(2020)
摘要
Determining the degree of parallelism (DOP) for query execution is of great importance to both performance and resource provisioning. However, recent work that applies machine learning (ML) to query optimization and query performance prediction in relational database management systems (RDBMSs) has ignored the effect of intra-query parallelism. In this work, we argue that determining the optimal or near-optimal DOP for query execution is a fundamental and challenging task that benefits both query performance and cost-benefit tradeoffs. We then present promising preliminary results on how ML techniques can be applied to automate DOP tuning. We conclude with a list of challenges we encountered, as well as future directions for our work.
更多查看译文
AI 理解论文
溯源树
样例
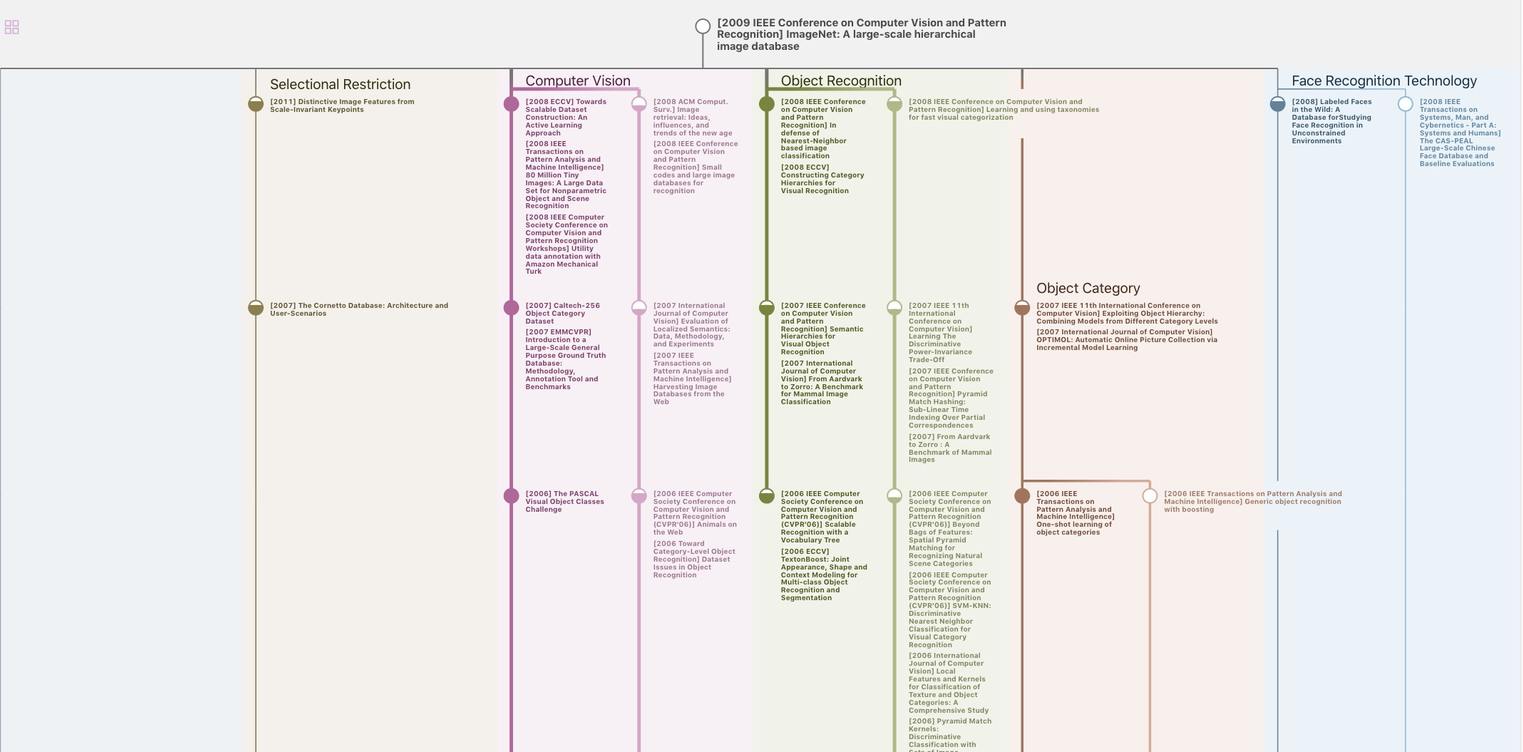
生成溯源树,研究论文发展脉络
Chat Paper
正在生成论文摘要