Comparing the interpretability of deep networks via network dissection
Explainable AI: Interpreting, Explaining and Visualizing Deep Learning(2019)
摘要
In this chapter, we introduce Network Dissection (The complete paper and code are available at http://netdissect.csail.mit.edu ), a general framework to quantify the interpretability of the units inside a deep convolutional neural networks (CNNs). We compare the different vocabularies of interpretable units as concept detectors emerged from the networks trained to solve different supervised learning tasks such as object recognition on ImageNet and scene classification on Places. The network dissection is further applied to analyze how the units acting as semantic detectors grow and evolve over the training iterations both in the scenario of the train-from-scratch and in the stage of the fine-tuning between data sources. Our results highlight that interpretability is an important property of deep neural networks that provides new insights into their hierarchical structure.
更多查看译文
AI 理解论文
溯源树
样例
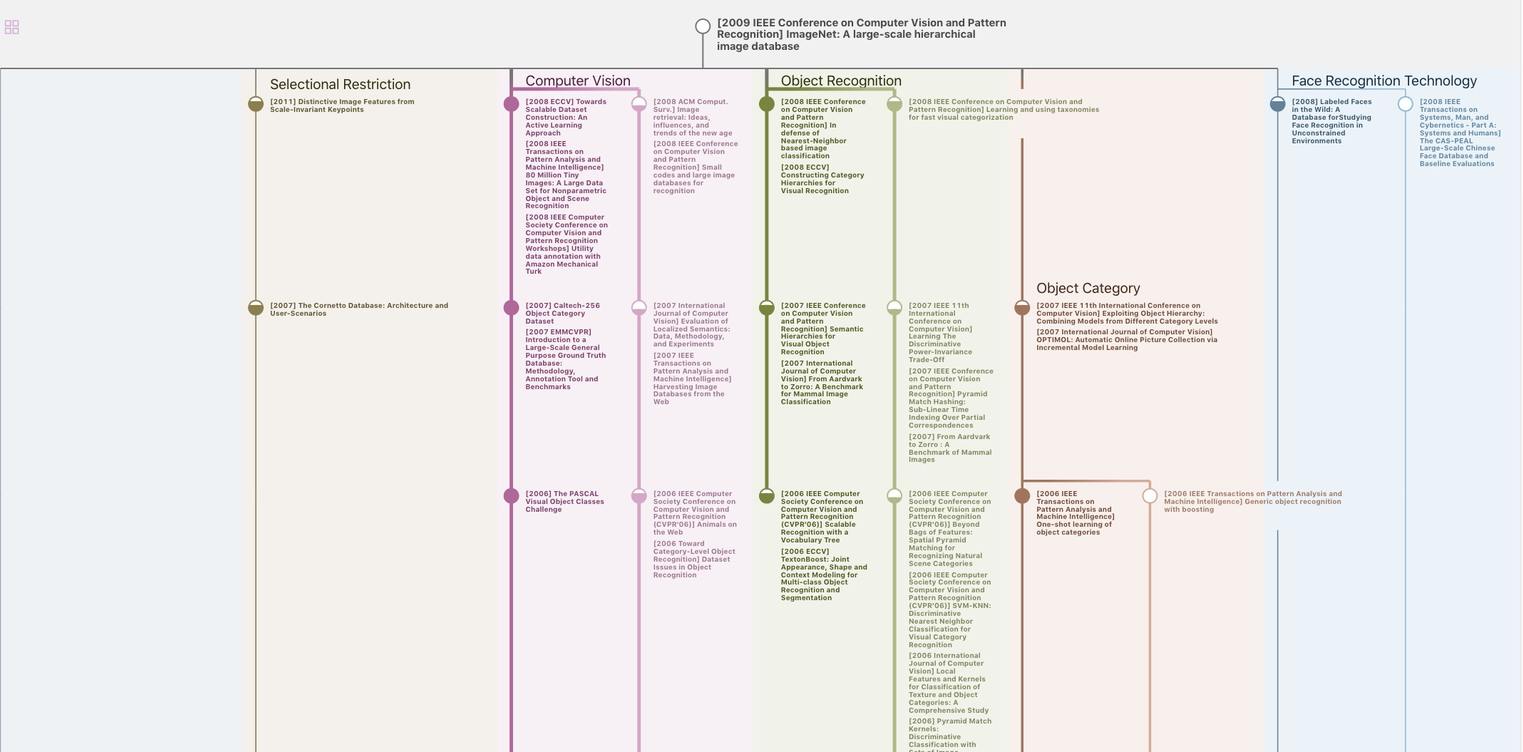
生成溯源树,研究论文发展脉络
Chat Paper
正在生成论文摘要