Deep Neighborhood Component Analysis for Visual Similarity Modeling
ACM Transactions on Intelligent Systems and Technology(2020)
摘要
AbstractLearning effective visual similarity is an essential problem in multimedia research. Despite the promising progress made in recent years, most existing approaches learn visual features and similarities in two separate stages, which inevitably limits their performance. Once useful information has been lost in the feature extraction stage, it can hardly be recovered later. This article proposes a novel end-to-end approach for visual similarity modeling, called deep neighborhood component analysis, which discriminatively trains deep neural networks to jointly learn visual features and similarities. Specifically, we first formulate a metric learning objective that maximizes the intra-class correlations and minimizes the inter-class correlations under the neighborhood component analysis criterion, and then train deep convolutional neural networks to learn a nonlinear mapping that projects visual instances from original feature space to a discriminative and neighborhood-structure-preserving embedding space, thus resulting in better performance. We conducted extensive evaluations on several widely used and challenging datasets, and the impressive results demonstrate the effectiveness of our proposed approach.
更多查看译文
关键词
Metric learning, visual similarity modeling, neighborhood component analysis
AI 理解论文
溯源树
样例
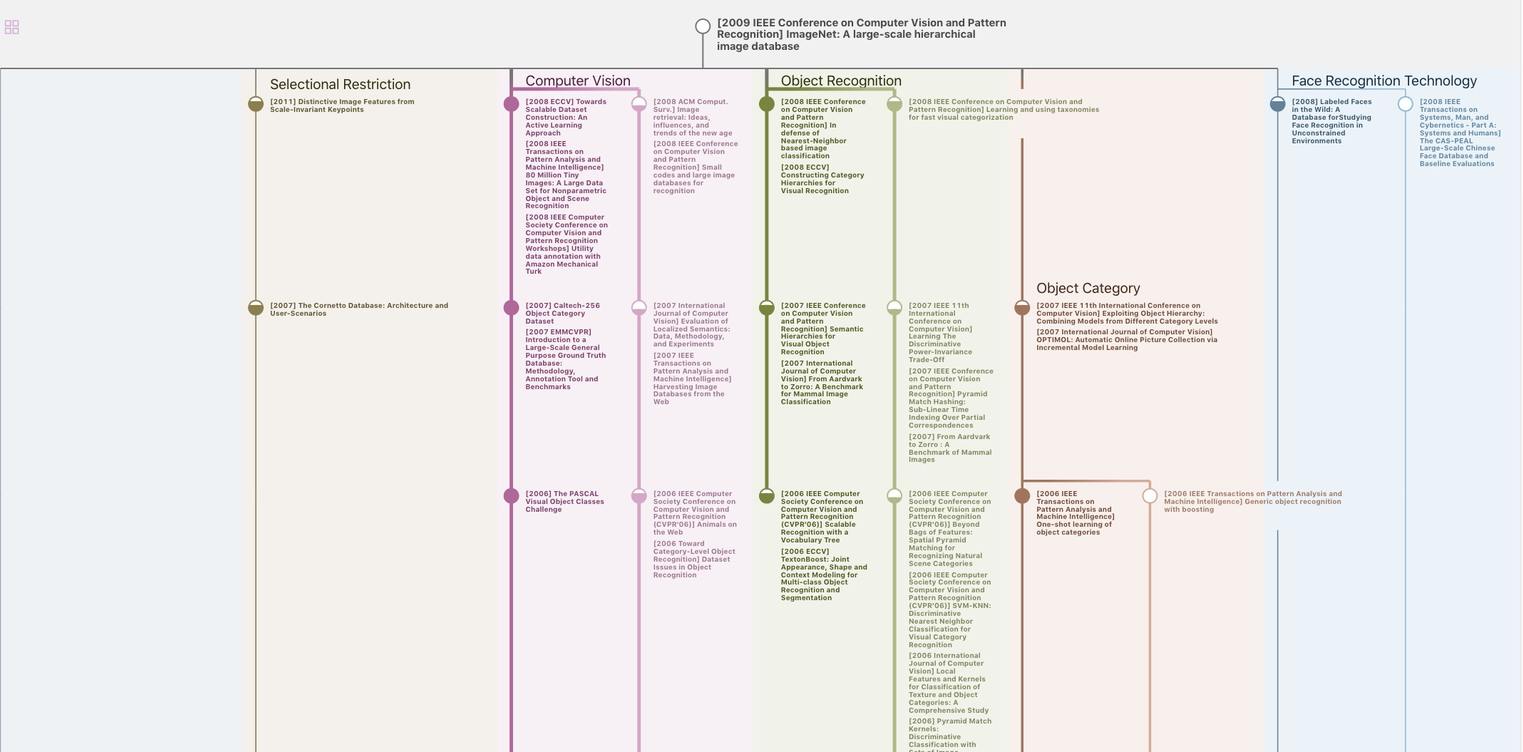
生成溯源树,研究论文发展脉络
Chat Paper
正在生成论文摘要