Bodies At Rest: 3d Human Pose And Shape Estimation From A Pressure Image Using Synthetic Data
2020 IEEE/CVF CONFERENCE ON COMPUTER VISION AND PATTERN RECOGNITION (CVPR)(2020)
摘要
People spend a substantial part of their lives at rest in bed. 3D human pose and shape estimation for this activity would have numerous beneficial applications, yet line-of-sight perception is complicated by occlusion from bedding. Pressure sensing mats are a promising alternative, but training data is challenging to collect at scale. We describe a physics-based method that simulates human bodies at rest in a bed with a pressure sensing mat, and present Pressure-Pose, a synthetic dataset with 206K pressure images with 3D human poses and shapes. We also present PressureNet, a deep learning model that estimates human pose and shape given a pressure image and gender. PressureNet incorporates a pressure map reconstruction (PMR) network that models pressure image generation to promote consistency between estimated 3D body models and pressure image input. In our evaluations, PressureNet performed well with real data from participants in diverse poses, even though it had only been trained with synthetic data. When we ablated the PMR network, performance dropped substantially.
更多查看译文
关键词
pressure image generation,diverse poses,pressure image input,estimated 3D body models,pressure map reconstruction network,deep learning model,PressureNet,synthetic dataset,human bodies,physics-based method,training data,pressure sensing mat,line-of-sight perception,synthetic data,shape estimation,3D human pose
AI 理解论文
溯源树
样例
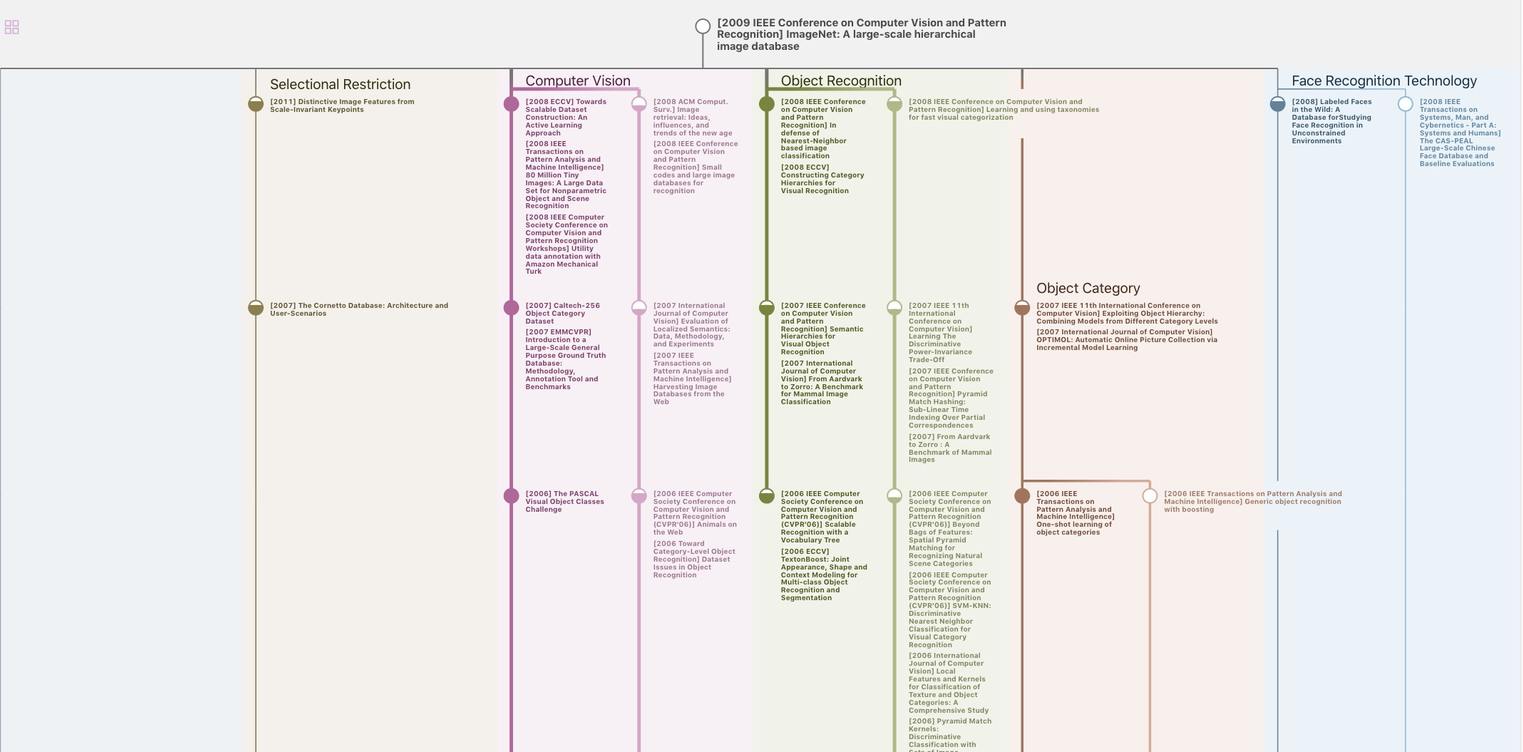
生成溯源树,研究论文发展脉络
Chat Paper
正在生成论文摘要