Inflated Episodic Memory With Region Self-Attention for Long-Tailed Visual Recognition
2020 IEEE/CVF CONFERENCE ON COMPUTER VISION AND PATTERN RECOGNITION (CVPR)(2020)
摘要
There have been increasing interests in modeling long-tailed data. Unlike artificially collected datasets, long-tailed data are naturally existed in the real-world and thus more realistic. To deal with the class imbalance problem, we introduce an Inflated Episodic Memory (IEM) for long-tailed visual recognition. First, our IEM augments the convolutional neural networks with categorical representative features for rapid learning on tail classes. In traditional few-shot learning, a single prototype is usually leveraged to represent a category. However, long-tailed data has higher intra-class variances. It could be challenging to learn a single prototype for one category. Thus, we introduce IEM to store the most discriminative feature for each category individually. Besides, the memory banks are updated independently, which further decreases the chance of learning skewed classifiers. Second, we introduce a novel region self-attention mechanism for multi-scale spatial feature map encoding. It is beneficial to incorporate more discriminative features to improve generalization on tail classes. We propose to encode local feature maps at multiple scales, and the spatial contextual information should be aggregated at the same time. Equipped with IEM and region self-attention, we achieve state-of-the-art performance on four standard long-tailed image recognition benchmarks. Besides, we validate the effectiveness of IEM on a long-tailed video recognition benchmark, i.e., YouTube-8M.
更多查看译文
关键词
Inflated Episodic Memory,IEM,long-tailed visual recognition,categorical representative features,tail classes,long-tailed data,intra-class variances,discriminative feature,multiscale spatial feature map encoding,region self-attention,long-tailed video recognition benchmark,Inflated episodic memory,class imbalance problem
AI 理解论文
溯源树
样例
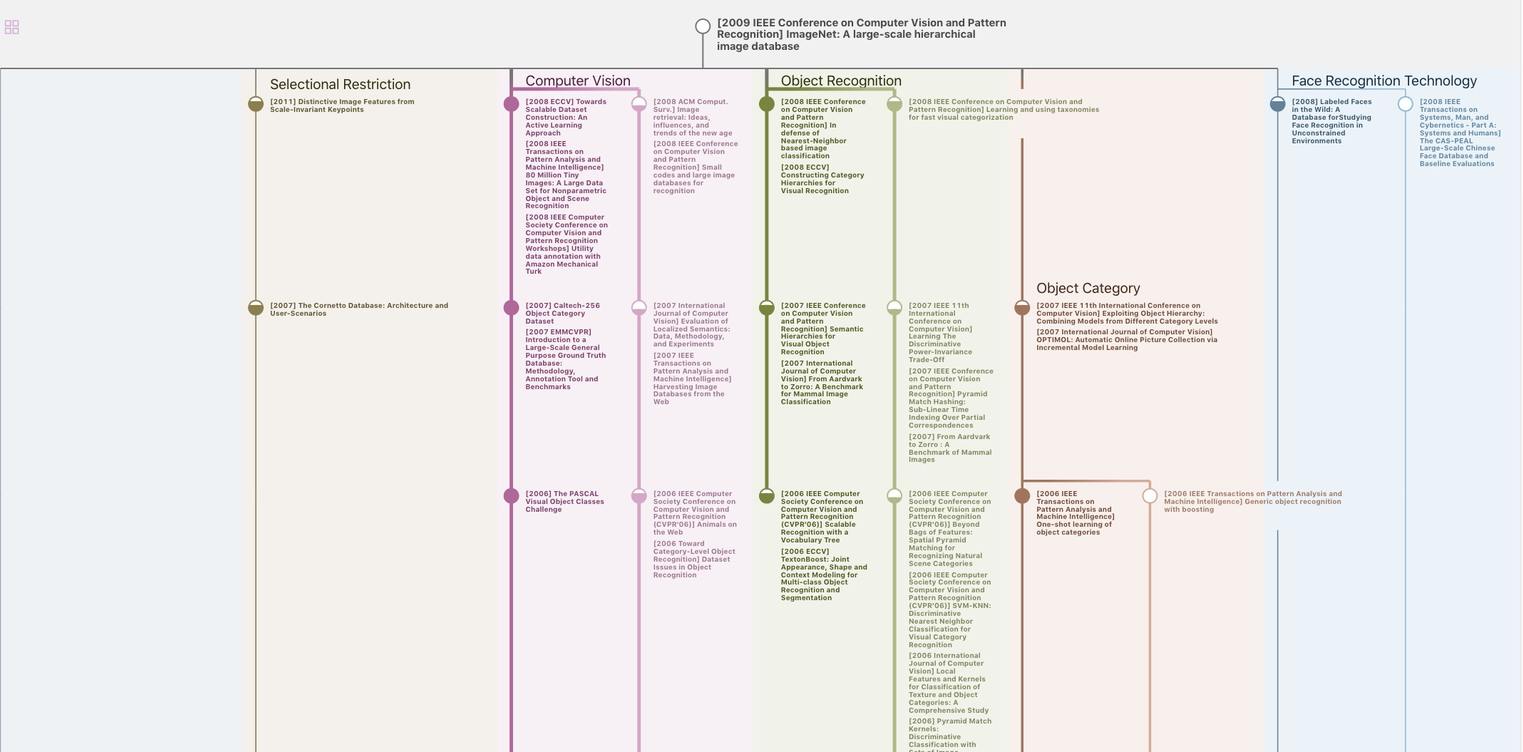
生成溯源树,研究论文发展脉络
Chat Paper
正在生成论文摘要