On Distributed Stochastic Gradient Descent For Nonconvex Functions In The Presence Of Byzantines
2020 IEEE INTERNATIONAL CONFERENCE ON ACOUSTICS, SPEECH, AND SIGNAL PROCESSING(2020)
摘要
We consider the distributed stochastic optimization problem of minimizing a nonconvex function f in an adversarial setting. All the w worker nodes in the network are expected to send their stochastic gradient vectors to the fusion center (or server). However, some (at most alpha-fraction) of the nodes may be Byzantines, which may send arbitrary vectors instead. Vanilla implementation of distributed stochastic gradient descent (SGD) cannot handle such misbehavior from the nodes. We propose a robust variant of distributed SGD which is resilient to the presence of Byzantines. The fusion center employs a novel filtering rule that identifies and removes the Byzantine nodes. We show that T = (O) over tilde (1/wc(2) + alpha(2)/c(2)) iterations are needed to achieve an epsilon-approximate stationary point (x such that parallel to del f(x)parallel to(2) <= epsilon) for the nonconvex learning problem. Unlike other existing approaches, the proposed algorithm is independent of the problem dimension.
更多查看译文
关键词
Byzantines, Stochastic Gradient Descent, Distributed optimization, Adversarial machine learning
AI 理解论文
溯源树
样例
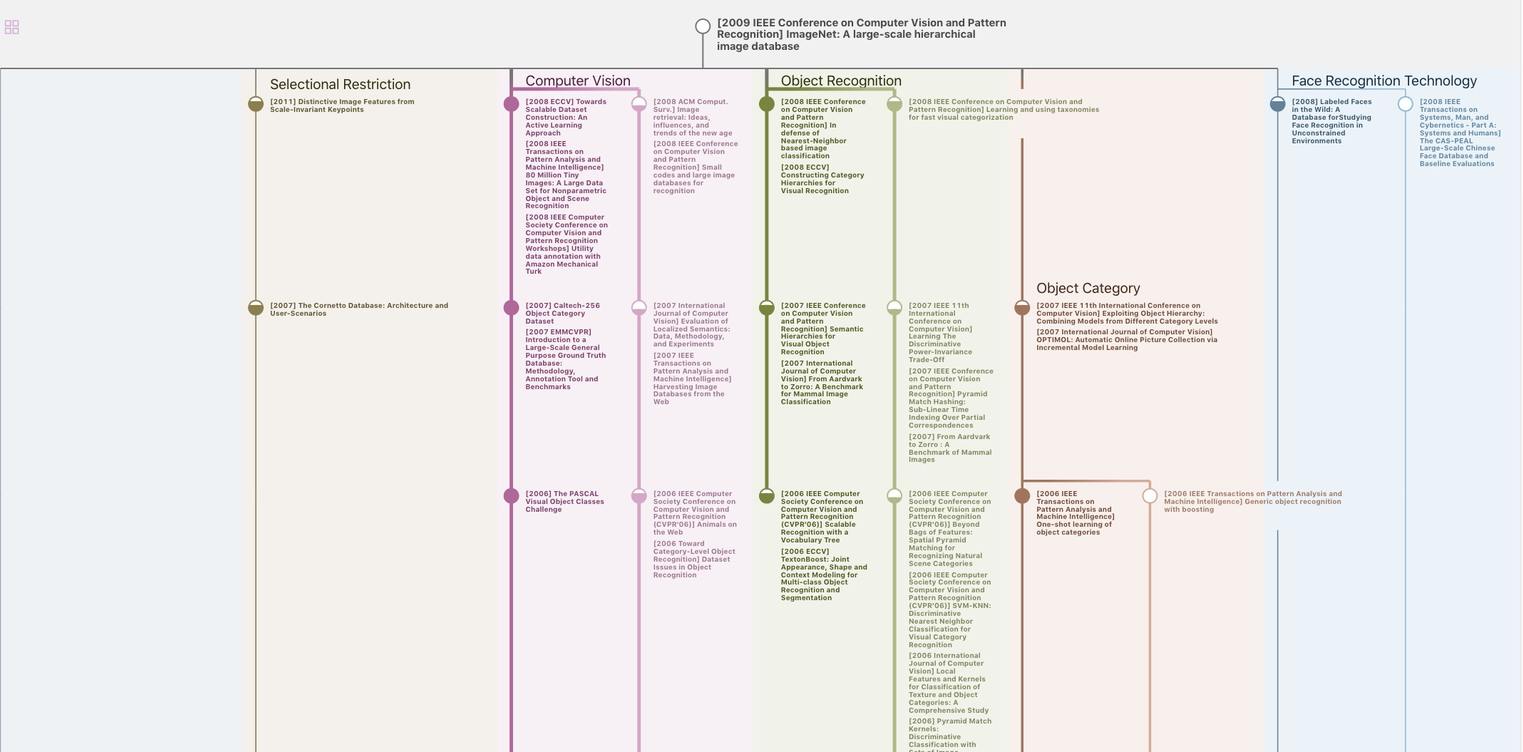
生成溯源树,研究论文发展脉络
Chat Paper
正在生成论文摘要