RMoR-Aion: Robust Multioutput Regression by Simultaneously Alleviating Input and Output Noises
IEEE Transactions on Neural Networks and Learning Systems(2021)
摘要
Multioutput regression, referring to simultaneously predicting multiple continuous output variables with a single model, has drawn increasing attention in the machine learning community due to its strong ability to capture the correlations among multioutput variables. The methodology of output space embedding, built upon the low-rank assumption, is now the mainstream for multioutput regression since it can effectively reduce the parameter numbers while achieving effective performance. The existing low-rank methods, however, are sensitive to the noises of both inputs and outputs, referring to the noise problem. In this article, we develop a novel multioutput regression method by simultaneously alleviating input and output noises, namely, robust multioutput regression by alleviating input and output noises (RMoR-Aion), where both the noises of the input and output are exploited by leveraging auxiliary matrices. Furthermore, we propose a prediction output manifold constraint with the correlation information regarding the output variables to further reduce the adversarial effects of the noise. Our empirical studies demonstrate the effectiveness of RMoR-Aion compared with the state-of-the-art baseline methods, and RMoR-Aion is more stable in the settings with artificial noise.
更多查看译文
关键词
Manifold regularization,multioutput regression,noisy responses,unobservable latent features
AI 理解论文
溯源树
样例
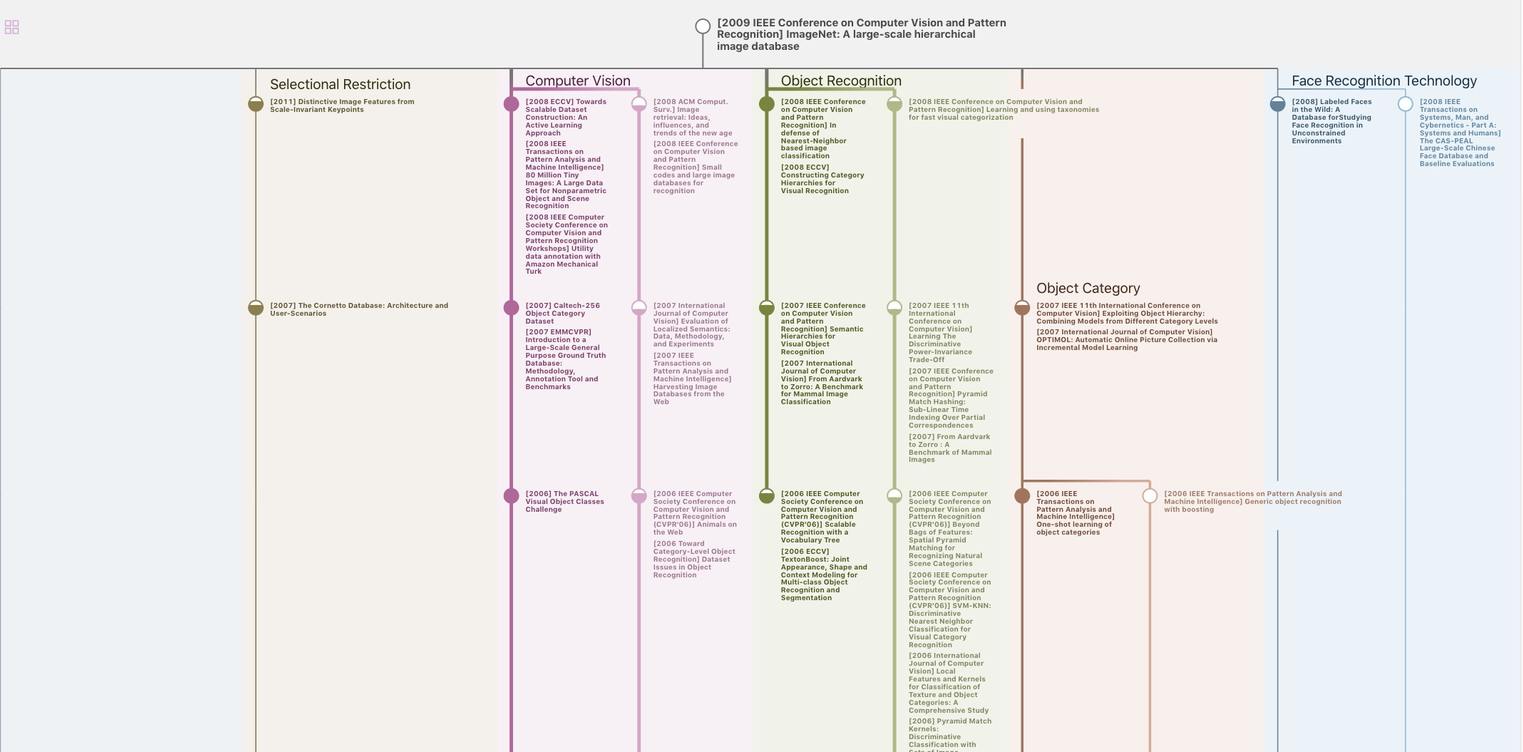
生成溯源树,研究论文发展脉络
Chat Paper
正在生成论文摘要