Off-policy Learning in Two-stage Recommender Systems
WWW '20: The Web Conference 2020 Taipei Taiwan April, 2020(2020)
摘要
Many real-world recommender systems need to be highly scalable: matching millions of items with billions of users, with milliseconds latency. The scalability requirement has led to widely used two-stage recommender systems, consisting of efficient candidate generation model(s) in the first stage and a more powerful ranking model in the second stage.
Logged user feedback, e.g., user clicks or dwell time, are often used to build both candidate generation and ranking models for recommender systems. While it’s easy to collect large amount of such data, they are inherently biased because the feedback can only be observed on items recommended by the previous systems. Recently, off-policy correction on such biases have attracted increasing interest in the field of recommender system research. However, most existing work either assumed that the recommender system is a single-stage system or only studied how to apply off-policy correction to the candidate generation stage of the system without explicitly considering the interactions between the two stages.
In this work, we propose a two-stage off-policy policy gradient method, and showcase that ignoring the interaction between the two stages leads to a sub-optimal policy in two-stage recommender systems. The proposed method explicitly takes into account the ranking model when training the candidate generation model, which helps improve the performance of the whole system. We conduct experiments on real-world datasets with large item space and demonstrate the effectiveness of our proposed method.
更多查看译文
关键词
Recommender Systems, Off-policy Learning, Two-stage Systems, Neural Networks
AI 理解论文
溯源树
样例
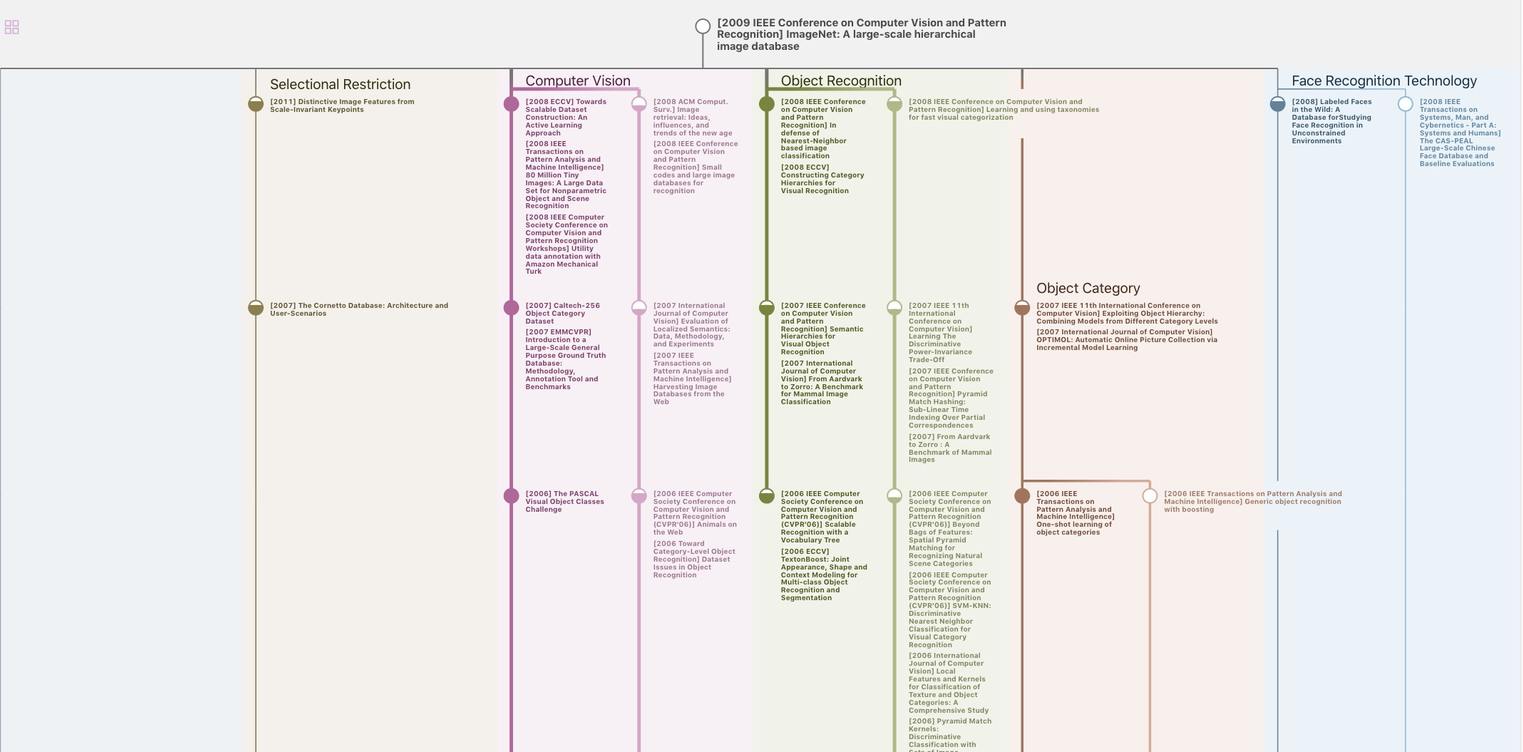
生成溯源树,研究论文发展脉络
Chat Paper
正在生成论文摘要