Intervention-Aware Early Warning
2019 19TH IEEE INTERNATIONAL CONFERENCE ON DATA MINING (ICDM 2019)(2019)
摘要
How can we early warn against an impending student drop out or an adverse health condition in near real-time? More challengingly, how do we learn to early warn from data containing confounding interventions-e.g., tutoring or medicines-while remaining interpretable to the human decision maker?We consider the problem of learning to interpretably early warn from labeled data tainted by interventions. We first identify three principles that an early warning system should follow. We then propose SmokeAlarm which provably obeys these principles and produces early warning scores in an online manner. Notably, learned model is "bi-inspectable", i.e., it can be visualized both in the presence and in the absence of interventions. Experiments demonstrate the efficacy of SmokeAlarm over prior approaches.
更多查看译文
关键词
early warning,early prediction,time series,intervention awareness,event detection
AI 理解论文
溯源树
样例
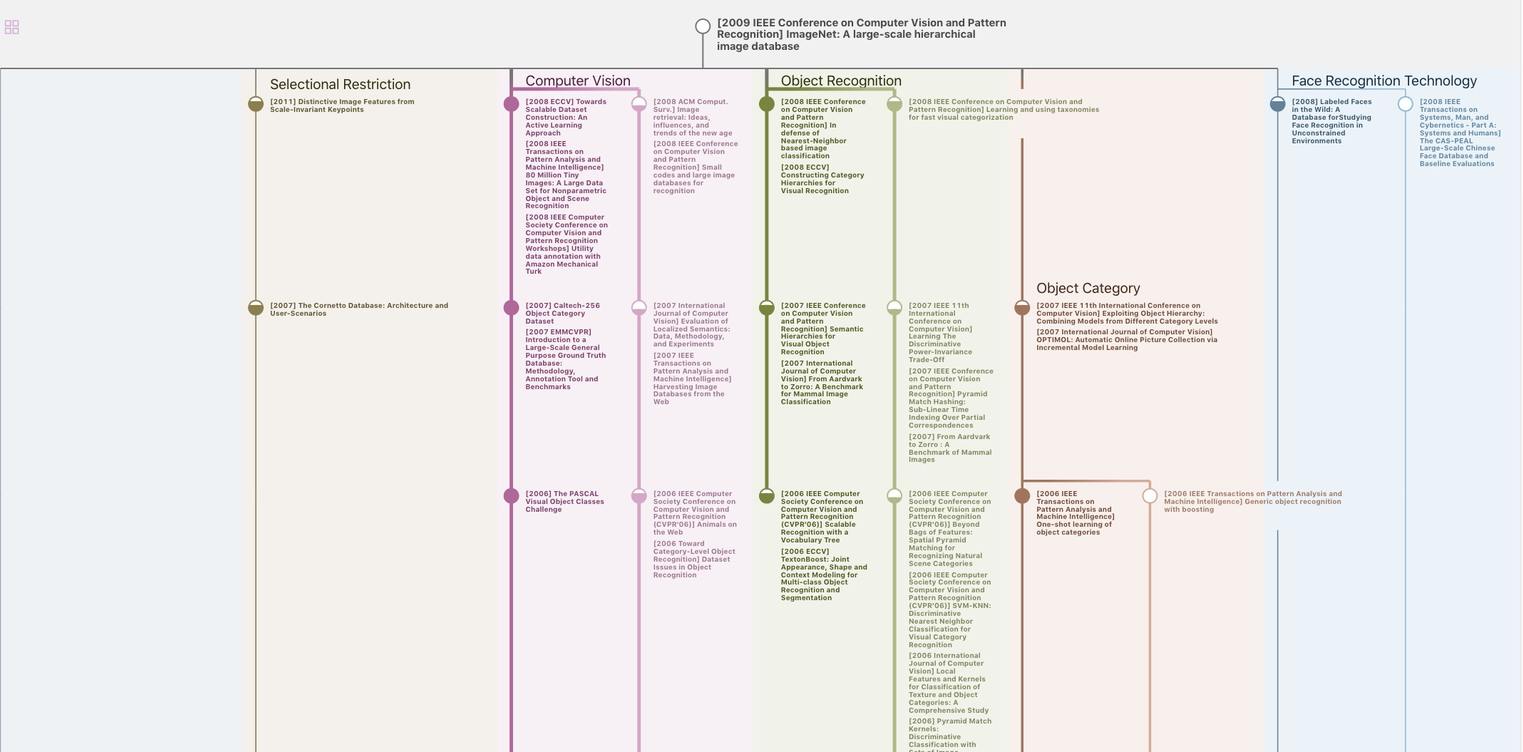
生成溯源树,研究论文发展脉络
Chat Paper
正在生成论文摘要