Enriched Feature Guided Refinement Network For Object Detection
2019 IEEE/CVF INTERNATIONAL CONFERENCE ON COMPUTER VISION (ICCV 2019)(2019)
摘要
We propose a single-stage detection framework that jointly tackles the problem of multi-scale object detection and class imbalance. Rather than designing deeper networks, we introduce a simple yet effective feature enrichment scheme to produce multi-scale contextual features. We further introduce a cascaded refinement scheme which first instills multi-scale contextual features into the prediction layers of the single-stage detector in order to enrich their discriminative power for multi-scale detection. Second, the cascaded refinement scheme counters the class imbalance problem by refining the anchors and enriched features to improve classification and regression. Experiments are performed on two benchmarks: PASCAL VOC and MS COCO. For a 320x320 input on the MS COCO test-dev, our detector achieves state-of-the-art single-stage detection accuracy with a COCO AP of 33.2 in the case of single-scale inference, while operating at 21 milliseconds on a Titan XP GPU. For a 512x512 input on the MS COCO test-dev, our approach obtains an absolute gain of 1.6% in terms of COCO AP, compared to the best reported singlestage results [5]. Source code and models are available at: https://github.com/Ranchentx/EFGRNet.
更多查看译文
关键词
single-stage detection framework,multiscale object detection,deeper networks,multiscale contextual features,single-stage detector,class imbalance problem,MS COCO test-dev,COCO AP,single-scale inference,single-stage detection accuracy,effective feature enrichment scheme,enriched feature guided refinement network,prediction layers,cascaded refinement scheme,PASCAL VOC,Titan XP GPU,time 21.0 ms
AI 理解论文
溯源树
样例
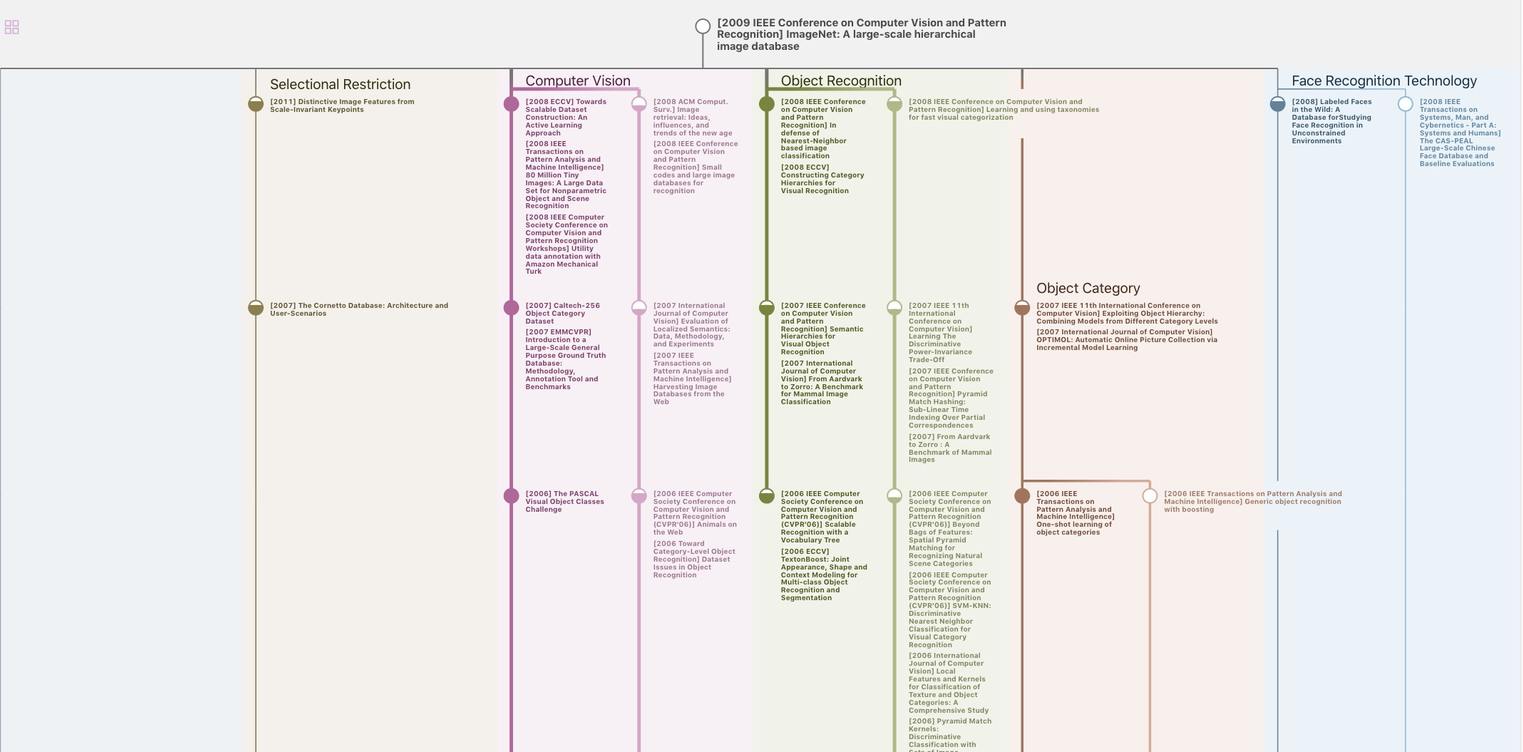
生成溯源树,研究论文发展脉络
Chat Paper
正在生成论文摘要