Deep Self-Paced Learning For Semi-Supervised Person Re-Identification Using Multi-View Self-Paced Clustering
2019 IEEE INTERNATIONAL CONFERENCE ON IMAGE PROCESSING (ICIP)(2019)
摘要
Semi-supervised person re-identification (Re-ID) is an extension of the existing popular Re-ID research, which only uses a small portion of labeled data, while the majority of the training samples are unlabeled. This paper approaches the problem by constructing a set of heterogeneous convolutional neural networks (CNNs) fine-tuned by utilizing the labeled training samples, and then propagating the labels to the unlabeled portion for further fine-tuning the overall system in a self-paced manner. In this work, a novel self-paced multi-view clustering is presented to generate pseudo labels for unlabeled training samples, which combines multiple heterogeneous CNNs features to cluster. In our clustering method, we introduce a self-paced regularizer to select reliable samples for fine-tuning each CNNs by minimizing ranking loss and identification loss. Specifically, we select a small portion of unlabeled training data when multiple CNNs are weak. With CNNs become stronger, more and more unlabeled samples are selected. Pseudo label estimation and CNNs training are improved simultaneously, which optimize alternatively until all the unlabeled training samples are selected. In our framework, both the optimization of multiple CNNs training and multi-view clustering on unlabeled training samples are self-paced optimizing procedure. Extensive experiments have been conducted on two large-scale Re-ID datasets to demonstrate the superiority of the proposed method.
更多查看译文
关键词
Person Re-Identification, Semi-Supervised Learning, Convolutional Neural Network, Multi-View Clustering, Self-paced Learning
AI 理解论文
溯源树
样例
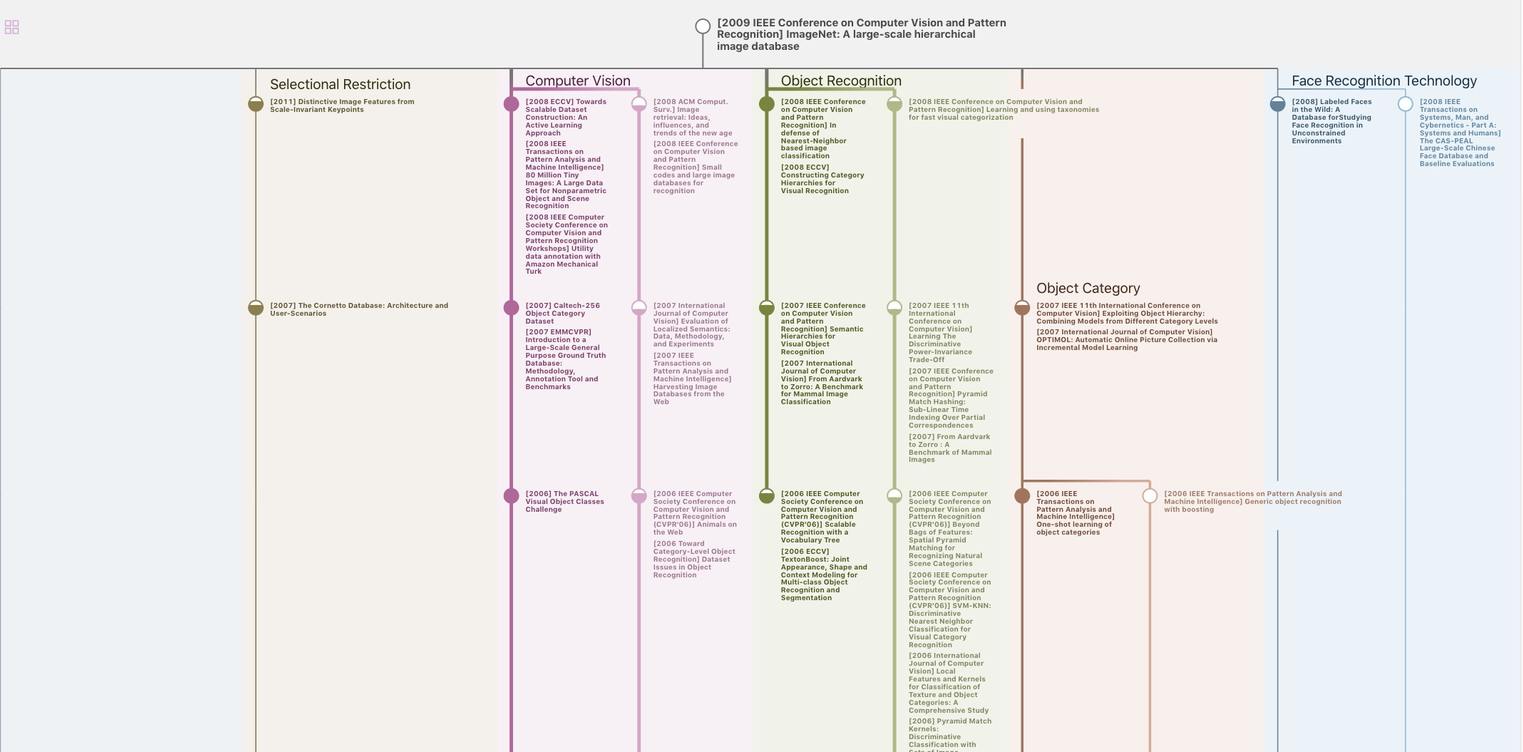
生成溯源树,研究论文发展脉络
Chat Paper
正在生成论文摘要