Learning-In-The-Loop Optimization: End-To-End Control And Co-Design of Soft Robots Through Learned Deep Latent Representations
NeurIPS(2019)
摘要
Soft robots have continuum solid bodies that can deform in an infinite number of ways. Controlling soft robots is very challenging as there are no closed form solutions. We present a learning-in-the-loop co-optimization algorithm in which a latent stale representation is learned as the robot figures out how to solve the task. Our solution marries hybrid particle-grid-based simulation with deep, variational convolutional autoencoder architectures that can capture salient features of robot dynamics with high efficacy. We demonstrate our dynamics-aware feature learning algorithm on both 21) and 31) soft robots, and show that it is more robust and faster converging than the dynamics-oblivious baseline. We validate the behavior of our algorithm with visualizations of the learned representation.
更多查看译文
关键词
infinite number
AI 理解论文
溯源树
样例
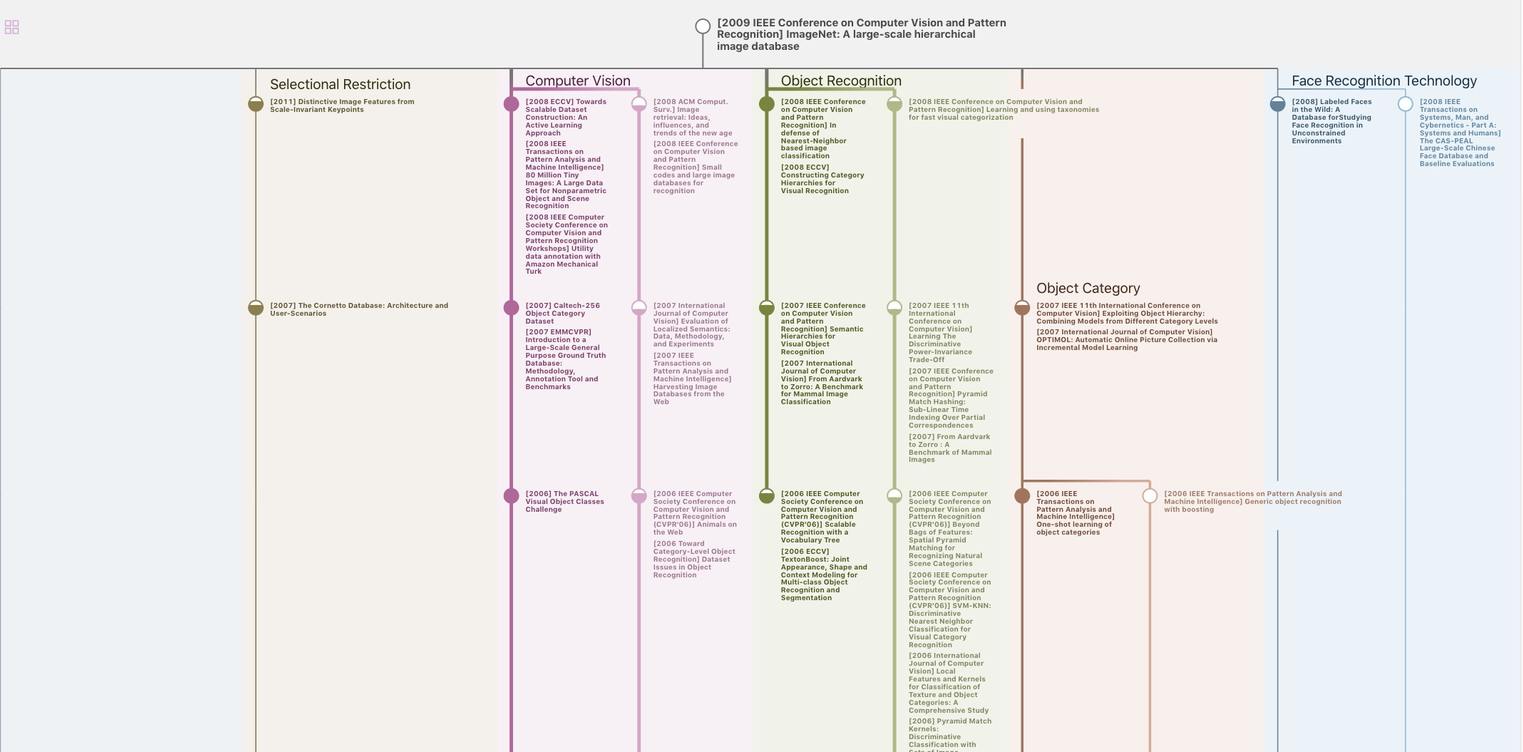
生成溯源树,研究论文发展脉络
Chat Paper
正在生成论文摘要