Title-Aware Neural News Topic Prediction
CHINESE COMPUTATIONAL LINGUISTICS, CCL 2019(2019)
摘要
Online news platforms have gained huge popularity for online news reading. The topic categories of news are very important for these platforms to target user interests and make personalized recommendations. However, massive news articles are generated everyday, and it too expensive and time-consuming to manually categorize all news. The news bodies usually convey the detailed information of news, and the news titles usually contain summarized and complementary information of news. However, existing news topic prediction methods usually simply aggregate news titles and bodies together and ignore the differences of their characteristics. In this paper, we propose a title-aware neural news topic prediction approach to classify the topic categories of online news articles. In our approach, we propose a multi-view learning framework to incorporate news titles and bodies as different views of news to learn unified news representations. In the title view, we learn title representations from words via a long-short term memory (LSTM) network, and use attention mechanism to select important words according to their contextual representations. In the body view, we propose to use a hierarchical LSTM network to first learn sentence representations from words, and then learn body representations from sentences. In addition, we apply attention networks at both word and sentence levels to recognize important words and sentences. Besides, we use the representation vector of news title to initialize the hidden states of the LSTM networks for news body to capture the summarized news information condensed by news titles. Extensive experiments on a real-world dataset validate that our approach can achieve good performance in news topic prediction and consistently outperform many baseline methods.
更多查看译文
关键词
News topic prediction, Multi-view learning, Attention mechanism
AI 理解论文
溯源树
样例
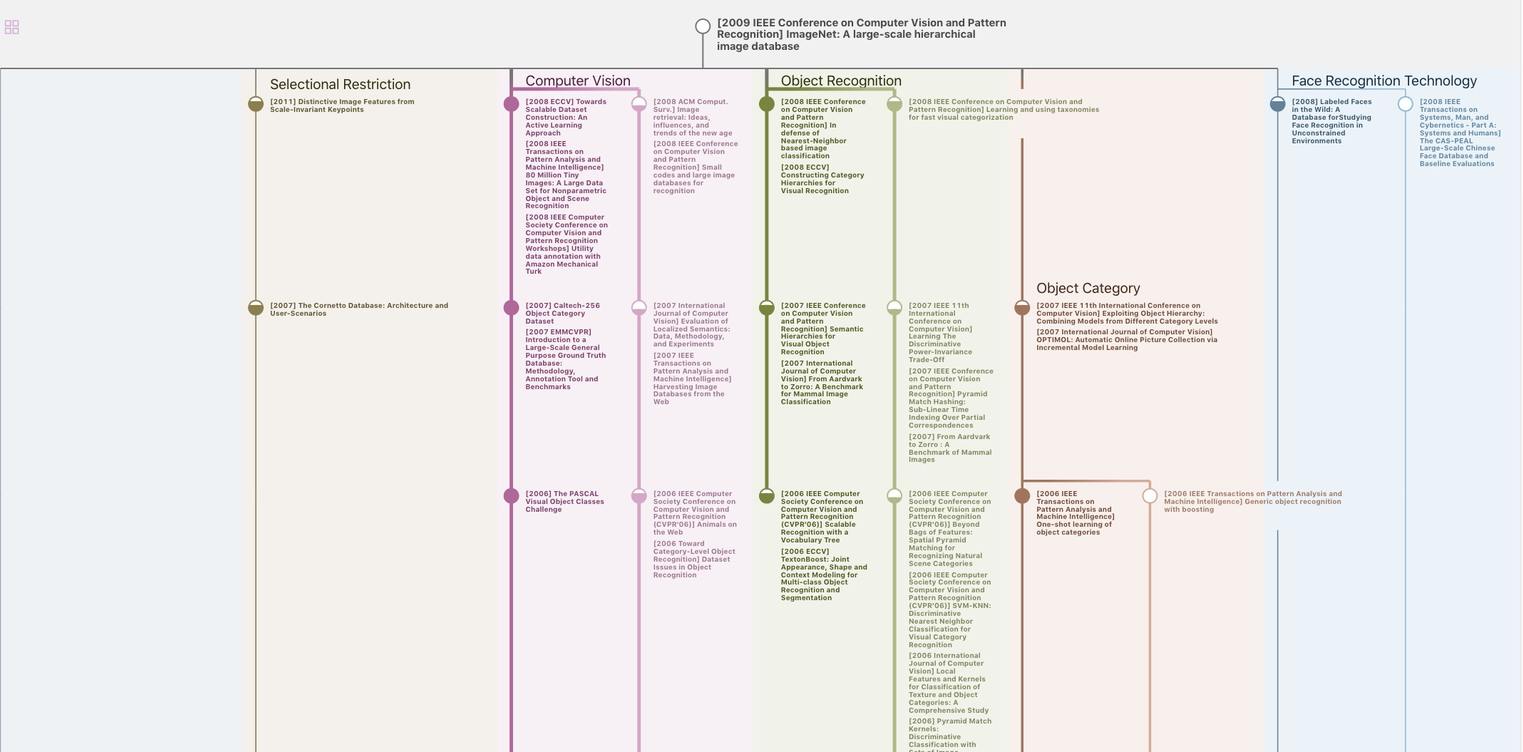
生成溯源树,研究论文发展脉络
Chat Paper
正在生成论文摘要