Sparse online collaborative filtering with dynamic regularization.
Information Sciences(2019)
摘要
Collaborative filtering (CF) approaches are widely applied in recommender systems. Traditional CF approaches have high costs to train the models and cannot capture changes in user interests and item popularity. Most CF approaches assume that user interests remain unchanged throughout the whole process. However, user preferences are always evolving and the popularity of items is always changing. Additionally, in a sparse matrix, the amount of known rating data is very small. In this paper, we propose a method of online collaborative filtering with dynamic regularization (OCF-DR), that considers dynamic information and uses the neighborhood factor to track the dynamic change in online collaborative filtering (OCF). The results from experiments on the MovieLens100K, MovieLens1M, and HetRec2011 datasets show that the proposed methods are significant improvements over several baseline approaches.
更多查看译文
关键词
Collaborative filtering,Dynamic regularization,Online collaborative filtering,Neighborhood factor
AI 理解论文
溯源树
样例
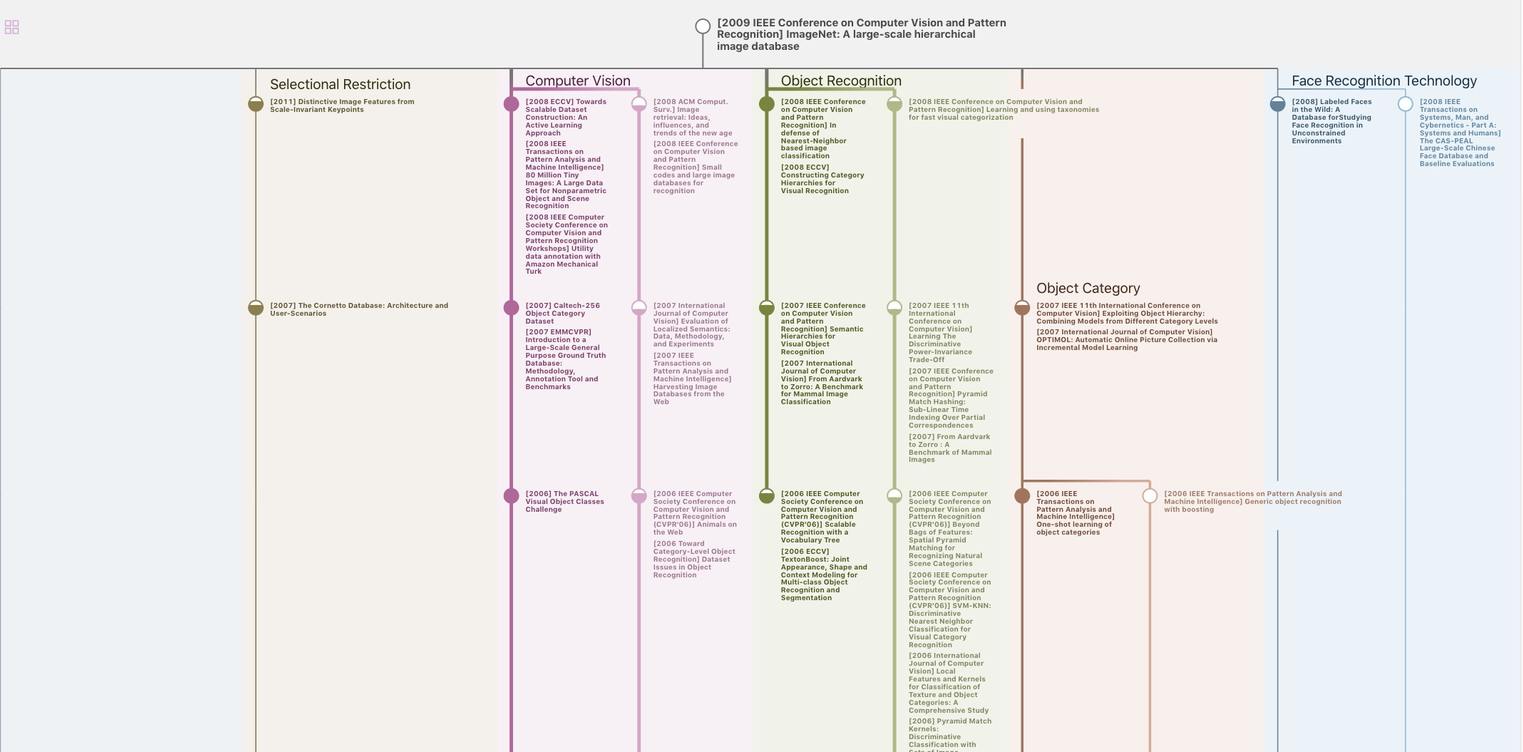
生成溯源树,研究论文发展脉络
Chat Paper
正在生成论文摘要