Improve computational efficiency and estimation accuracy of multi-channel surface EMG decomposition via dimensionality reduction.
Computers in Biology and Medicine(2019)
摘要
Electromyography (EMG) decomposition serves as a powerful tool to provide valuable information regarding motor control strategy and muscle pathology. Various approaches have recently been developed to decompose surface electromyography (sEMG) signals into motor unit action potential (MUAP) trains, but there is still room to improve decomposition accuracy and efficiency. In this article, the singular value decomposition (SVD) method is employed to decompose extended multi-channel sEMG signals based on the linear minimum mean square error (LMMSE) estimation and convolution kernel compensation (CKC). The column dimension of the right unitary matrix obtained via SVD is compressed to reduce the run-time of the decomposition process. As such, an innervation pulse train (IPT) can be extracted only using the compressed right unitary matrix. We studied the effect of reduced dimensionality on the computational efficiency and accuracy of the proposed method. Results from both simulated and experimental data demonstrate that this algorithm can decompose multi-channel sEMG efficiently and accurately. In most cases, 20%–60% of run-time can be reduced. In addition, the number of extracted IPTs can also be increased, especially when the signal-to-noise ratio of the EMG data is low. The method developed in this study has potential applications in clinical diagnosis, rehabilitation engineering and research on human motion control systems.
更多查看译文
关键词
LMMSE,SVD,Multi-channel,Clinical diagnosis,Rehabilitation engineering
AI 理解论文
溯源树
样例
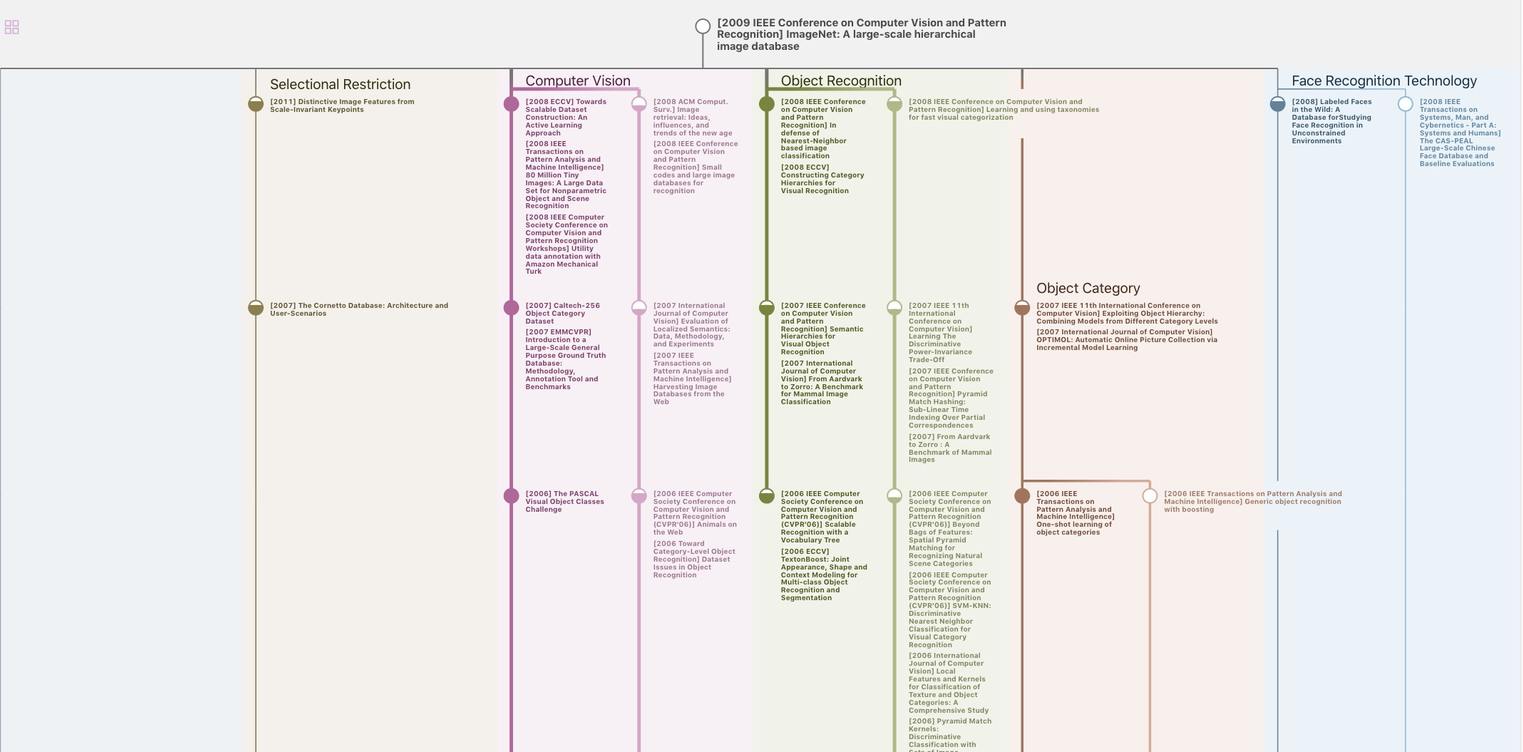
生成溯源树,研究论文发展脉络
Chat Paper
正在生成论文摘要