Recognizing Part Attributes With Insufficient Data
2019 IEEE/CVF International Conference on Computer Vision (ICCV)(2019)
摘要
Recognizing the attributes of objects and their parts is central to many computer vision applications. Although great progress has been made to apply object-level recognition, recognizing the attributes of parts remains less applicable since the training data for part attributes recognition is usually scarce especially for internet-scale applications. Furthermore, most existing part attribute recognition methods rely on the part annotations which are more expensive to obtain. In order to solve the data insufficiency problem and get rid of dependence on the part annotation, we introduce a novel Concept Sharing Network (CSN) for part attribute recognition. A great advantage of CSN is its capability of recognizing the part attribute (a combination of part location and appearance pattern) that has insufficient or zero training data, by learning the part location and appearance pattern respectively from the training data that usually mix them in a single label. Extensive experiments on CUB, Celeb A, and a newly proposed human attribute dataset demonstrate the effectiveness of CSN and its advantages over other methods, especially for the attributes with few training samples. Further experiments show that CSN can also perform zero-shot part attribute recognition.
更多查看译文
关键词
part location,zero training data,CSN,computer vision applications,object-level recognition,Internet-scale applications,data insufficiency problem,part annotation,human attribute dataset,concept sharing network,CUB,Celeb A,zero-shot part attribute recognition
AI 理解论文
溯源树
样例
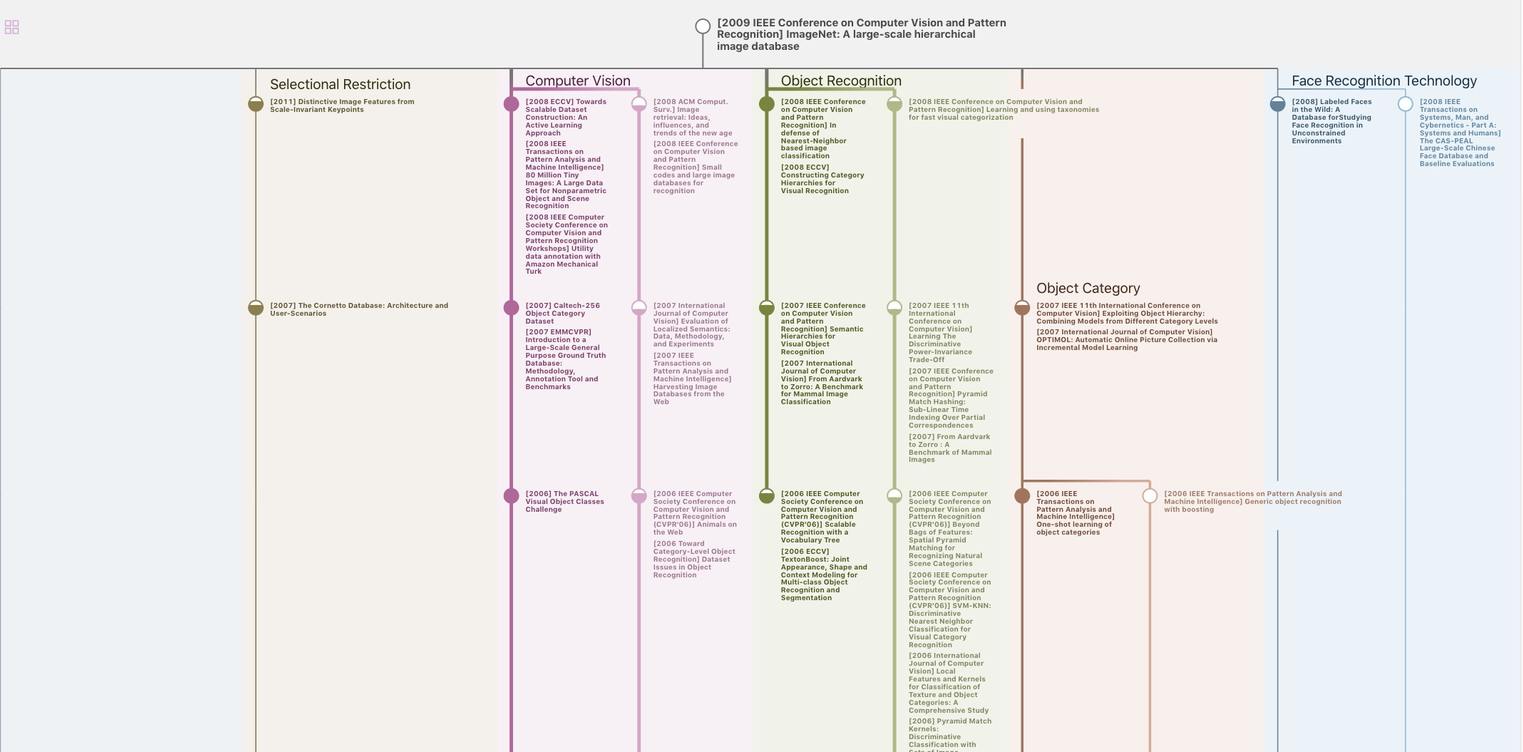
生成溯源树,研究论文发展脉络
Chat Paper
正在生成论文摘要