A 0.11 pJ/Op, 0.32-128 TOPS, Scalable Multi-Chip-Module-based Deep Neural Network Accelerator with Ground-Reference Signaling in 16nm
2019 SYMPOSIUM ON VLSI CIRCUITS(2019)
摘要
This work presents a scalable deep neural network (DNN) accelerator consisting of 36 chips connected in a mesh network on a multi-chip-module (MCM) using ground-referenced signaling (GRS). While previous accelerators fabricated on a single monolithic die are limited to specific network sizes, the proposed architecture enables flexible scaling for efficient inference on a wide range of DNNs, from mobile to data center domains. The 16nm prototype achieves 1.29 TOPS/mm
2
, 0.11 pJ/op energy efficiency, 4.01 TOPS peak performance for a 1chip system, and 127.8 peak TOPS and 2615 images/s ResNet50 inference for a 36-chip system.
更多查看译文
关键词
ground-reference signaling,scalable deep neural network accelerator,mesh network,ground-referenced signaling,scalable multichip module,DNN,MCM,GRS,monolithic die,data center domains,ResNet50 inference,size 16.0 nm
AI 理解论文
溯源树
样例
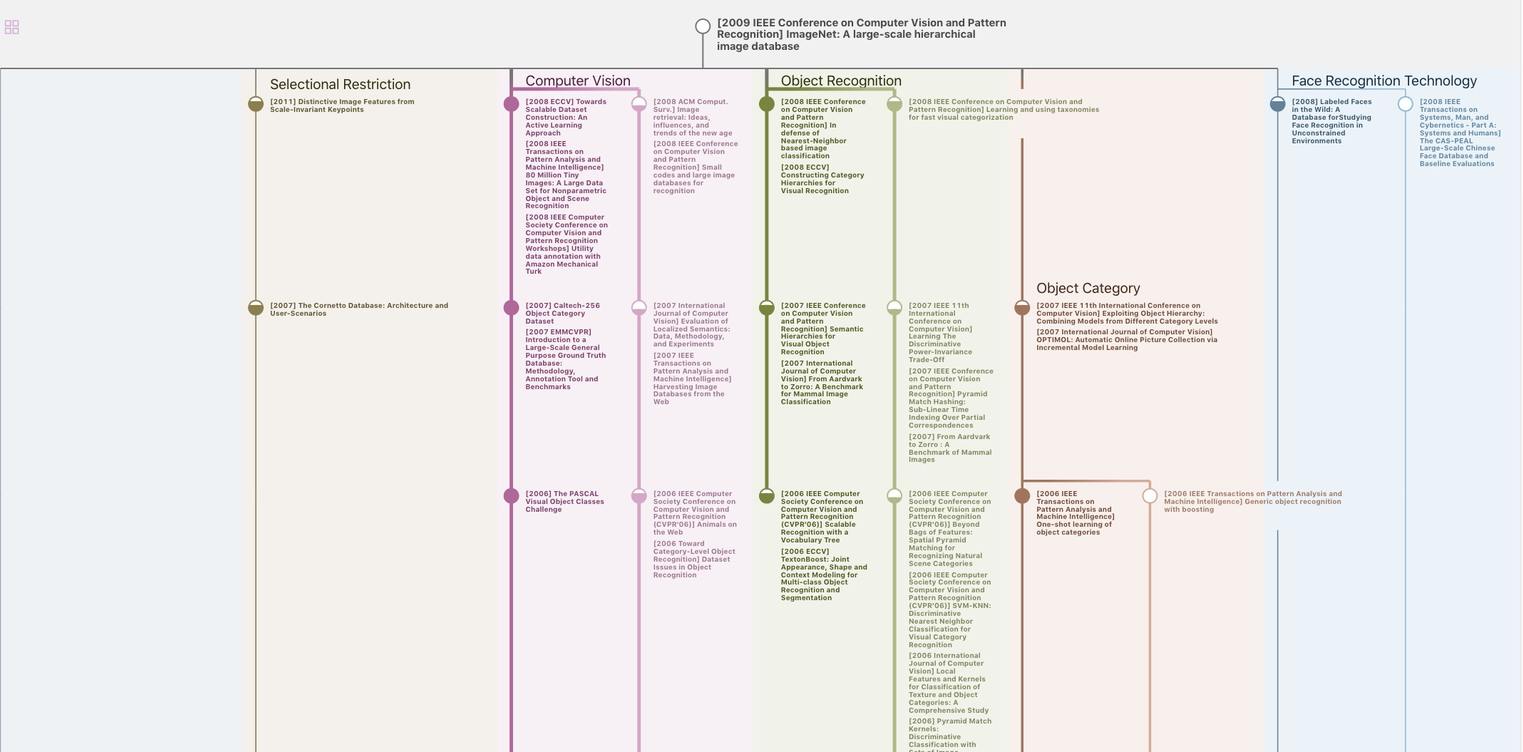
生成溯源树,研究论文发展脉络
Chat Paper
正在生成论文摘要