Solving symbolic regression problems with formal constraints
Genetic and Evolutionary Computation Conference(2019)
摘要
ABSTRACTIn many applications of symbolic regression, domain knowledge constrains the space of admissible models by requiring them to have certain properties, like monotonicity, convexity, or symmetry. As only a handful of variants of genetic programming methods proposed to date can take such properties into account, we introduce a principled approach capable of synthesizing models that simultaneously match the provided training data (tests) and meet user-specified formal properties. To this end, we formalize the task of symbolic regression with formal constraints and present a range of formal properties that are common in practice. We also conduct a comparative experiment that confirms the feasibility of the proposed approach on a suite of realistic symbolic regression benchmarks extended with various formal properties. The study is summarized with discussion of results, properties of the method, and implications for symbolic regression.
更多查看译文
关键词
genetic programming, symbolic regression, constraints, formal verification, generalization
AI 理解论文
溯源树
样例
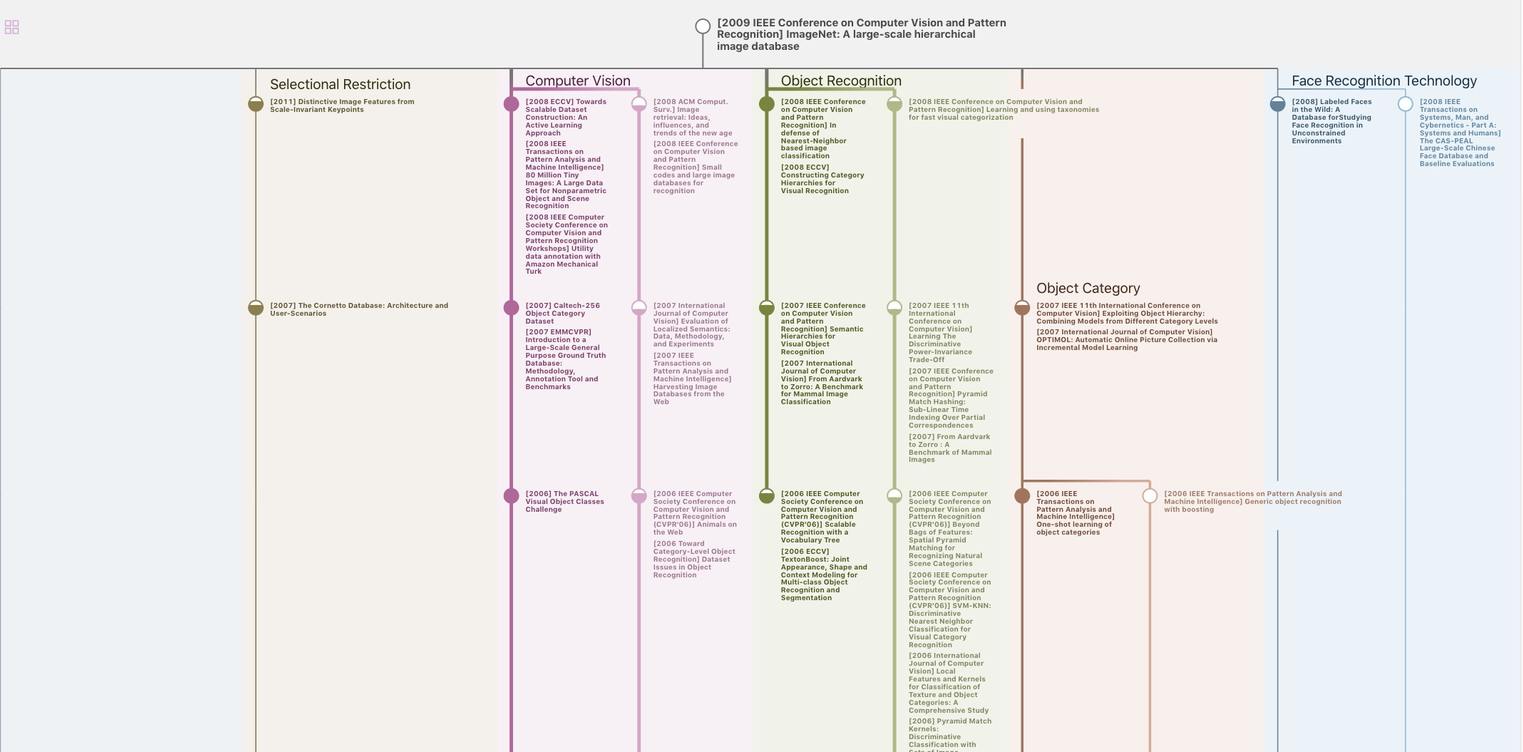
生成溯源树,研究论文发展脉络
Chat Paper
正在生成论文摘要