Src-Disp: Synthetic-Realistic Collaborative Disparity Learning For Stereo Matching
COMPUTER VISION - ACCV 2018, PT V(2018)
摘要
Stereo matching task has been greatly improved by convolutional neural networks, especially the fully-convolutional network. However, existing deep learning methods always overfit to specific domains. In this paper, focus on domain adaptation problem of disparity estimation, we present a novel training strategy to conduct synthetic-realistic collaborative learning. At first, we design a compact model that consists of shallow feature extractor, correlation feature aggregator and disparity encoder-decoder. Our model enables end-to-end disparity regression with fast speed and high accuracy. To perform collaborative learning, we then propose two distinct training schemes, including guided label distillation and semi-supervised regularization, both of which are used to alleviate the lack of disparity labels in realistic datasets. Finally, we evaluate the trained models on different datasets that belong to various domains. Comparative results demonstrate the capability of our designed model and the effectiveness of collaborative training strategy.
更多查看译文
关键词
Stereo matching, Collaborative learning, Disparity, Guided label distillation, Semi-supervised regularization
AI 理解论文
溯源树
样例
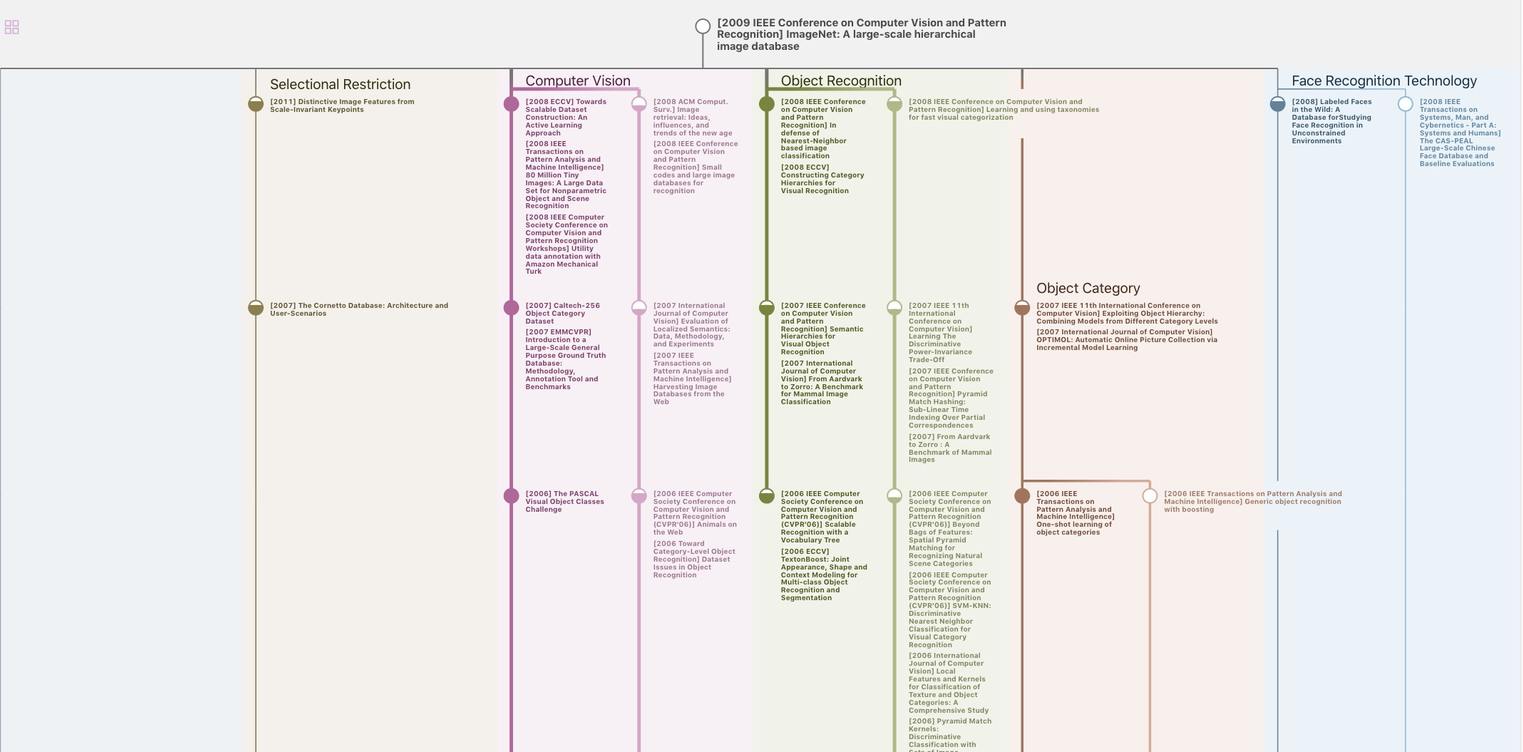
生成溯源树,研究论文发展脉络
Chat Paper
正在生成论文摘要