Predicting of Associations between MicroRNA and Human Diseases Based on Multiple Similarities and Arbitrarily-order Proximity Network Embedding
IEEE Access(2019)
摘要
Microbe-RNAs (miRNAs) play an important role and are associated with human diseases. However, considering the high cost and time-consuming biological experiments, using effective computational methods to discover the underlying association between the miRNAs and diseases would be valuable. This study presents a novel computational prediction model based on multiple-similarity and arbitrarily-order proximity network embedding. We obtain the Gaussian similarity from the disease-miRNA interaction matrix for the miRNA and disease. Then, considering the Gaussian similarity, disease semantic, phenotype similarity, and the miRNA functional similarity, we compute the miRNA-miRNA similarity matrix and the disease-disease similarity matrix. Most importantly, we improved the SVD matrix decomposition to extract the primary feature vector. We called it arbitrarily-order proximity network embedding method. By multiplying the feature vectors together, we calculate the final miRNA-disease association score matrix. According to the ranking scores, we can know which miRNA is mostly relevant to a disease. This process proved that our method achieved better prediction performance than other methods. In the experiment, after adding arbitrarily-order proximity network embedding to the inductive matrix completion method [1], the AUC of our method in leave-one-out cross-validation increased dramatically from 0.8034 to 0.92306. Meanwhile, the studies of three cases, namely, prostate neoplasms, breast neoplasms, and lung neoplasms, of the top 50 potential miRNAs predicted by our method were validated by the database of dbDEMC and mir2disease. This finding indicated that our method can effectively obtain the potential disease miRNA candidates. Comparison of our work with other algorithms reveals its reliable performance.
更多查看译文
关键词
Bioinformatics,biomedical informatics,biological interactions,prediction methods
AI 理解论文
溯源树
样例
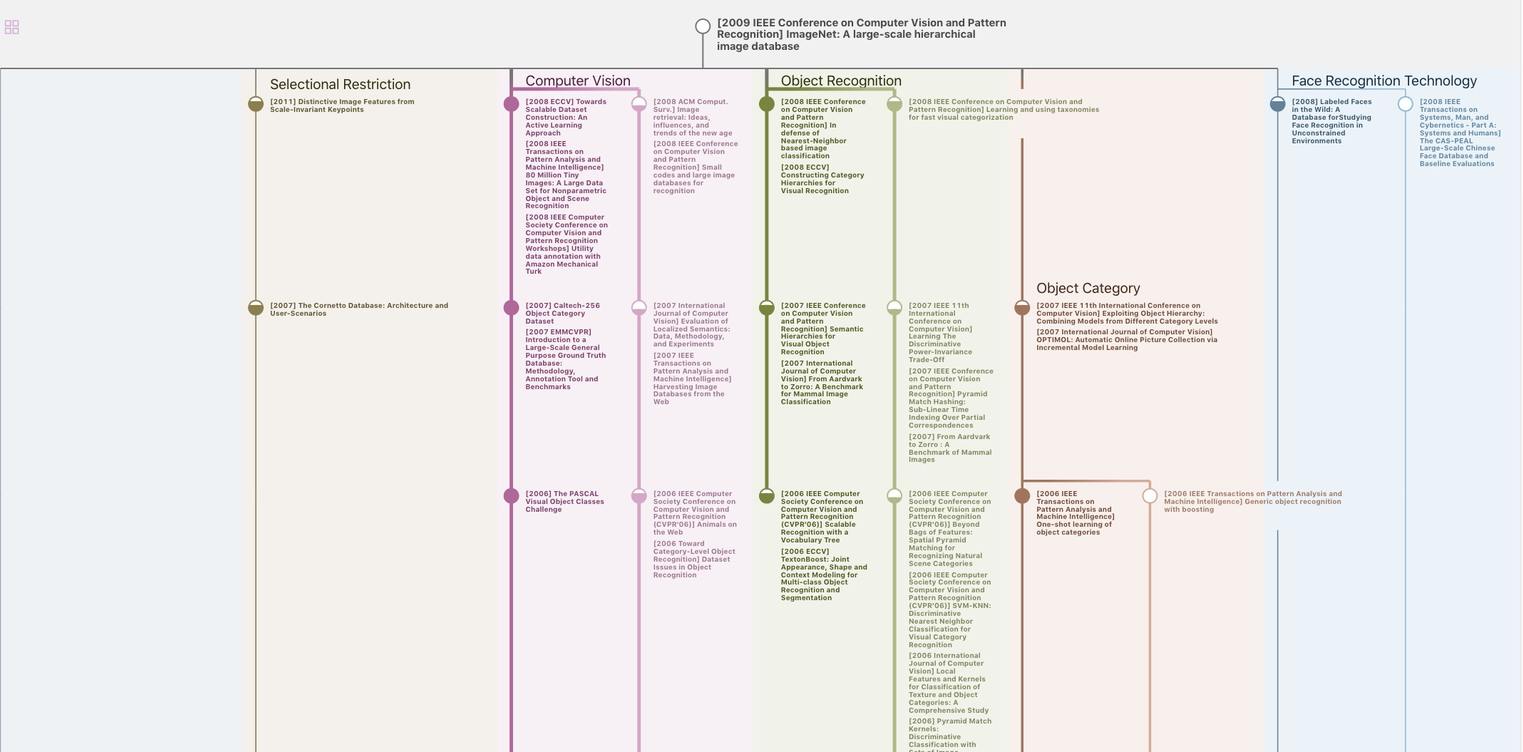
生成溯源树,研究论文发展脉络
Chat Paper
正在生成论文摘要