Event2Vec: Learning Event Representations Using Spatial-Temporal Information for Recommendation
pacific-asia conference on knowledge discovery and data mining(2019)
摘要
Event-based social networks (EBSN), such as meetup.com and plancast.com, have witnessed increased popularity and rapid growth in recent years. In EBSN, a user can choose to join any events such as a conference, house party, or drinking event. In this paper, we present a novel model—Event2Vec, which explores how representation learning for events incorporating spatial-temporal information can help event recommendation in EBSN. The spatial-temporal information represents the physical location and the time where and when an event will take place. It typically has been modeled as a bias in conventional recommendation models. However, such an approach ignores the rich semantics associated with the spatial-temporal information. In Event2Vec, the spatial-temporal influences are naturally incorporated into the learning of latent representations for events, so that Event2Vec predicts user’s preference on events more accurately. We evaluate the effectiveness of the proposed model on three real datasets; our experiments show that with a proper modeling of the spatial-temporal information, we can significantly improve event recommendation performance.
更多查看译文
AI 理解论文
溯源树
样例
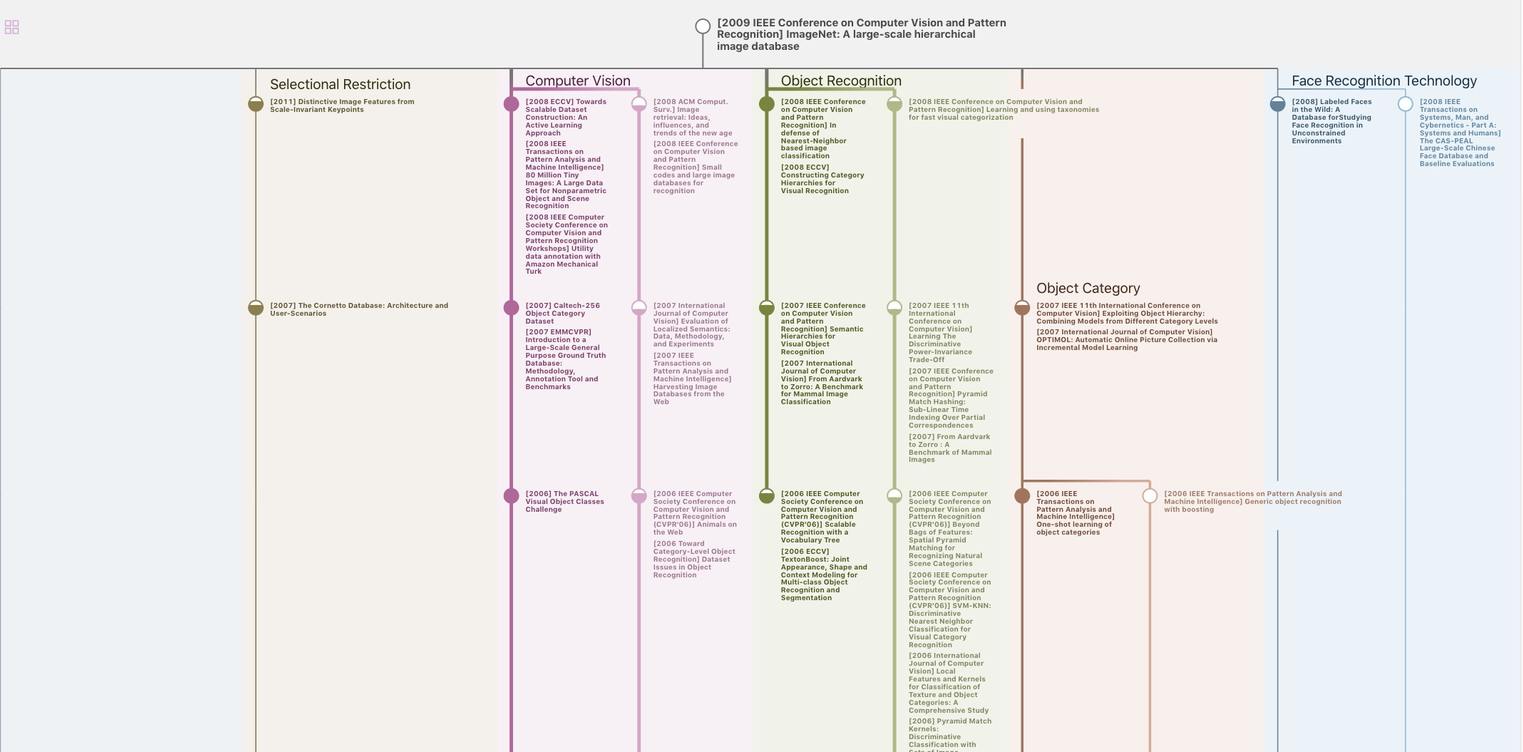
生成溯源树,研究论文发展脉络
Chat Paper
正在生成论文摘要