Meta Learning Deep Visual Words for Fast Video Object Segmentation
CoRR(2019)
摘要
Accurate video object segmentation methods finetune a model using the first annotated frame, and/or use additional inputs such as optical flow and complex post-processing. In contrast, we develop a fast algorithm that requires no finetuning, auxiliary inputs or post-processing, and segments a variable number of objects in a single forward-pass. We represent an object with clusters, or "visual words", in the embedding space, which correspond to object parts in the image space. This allows us to robustly match to the reference objects throughout the video, because although the global appearance of an object changes as it undergoes occlusions and deformations, the appearance of more local parts may stay consistent. We learn these visual words in an unsupervised manner, using meta-learning to ensure that our training objective matches our inference procedure. We achieve comparable accuracy to finetuning based methods, and state-of-the-art in terms of speed/accuracy trade-offs on four video segmentation datasets.
更多查看译文
关键词
meta-learning deep visual words,fast video object segmentation,personal robots,driverless cars,object classes,previous accurate methods,annotated frame,additional inputs,optical flow,complex post-processing,fast algorithm,causal algorithm,auxiliary inputs,single forward-pass,embedding space,image space,object changes,training objective,finetuning based methods,video segmentation datasets
AI 理解论文
溯源树
样例
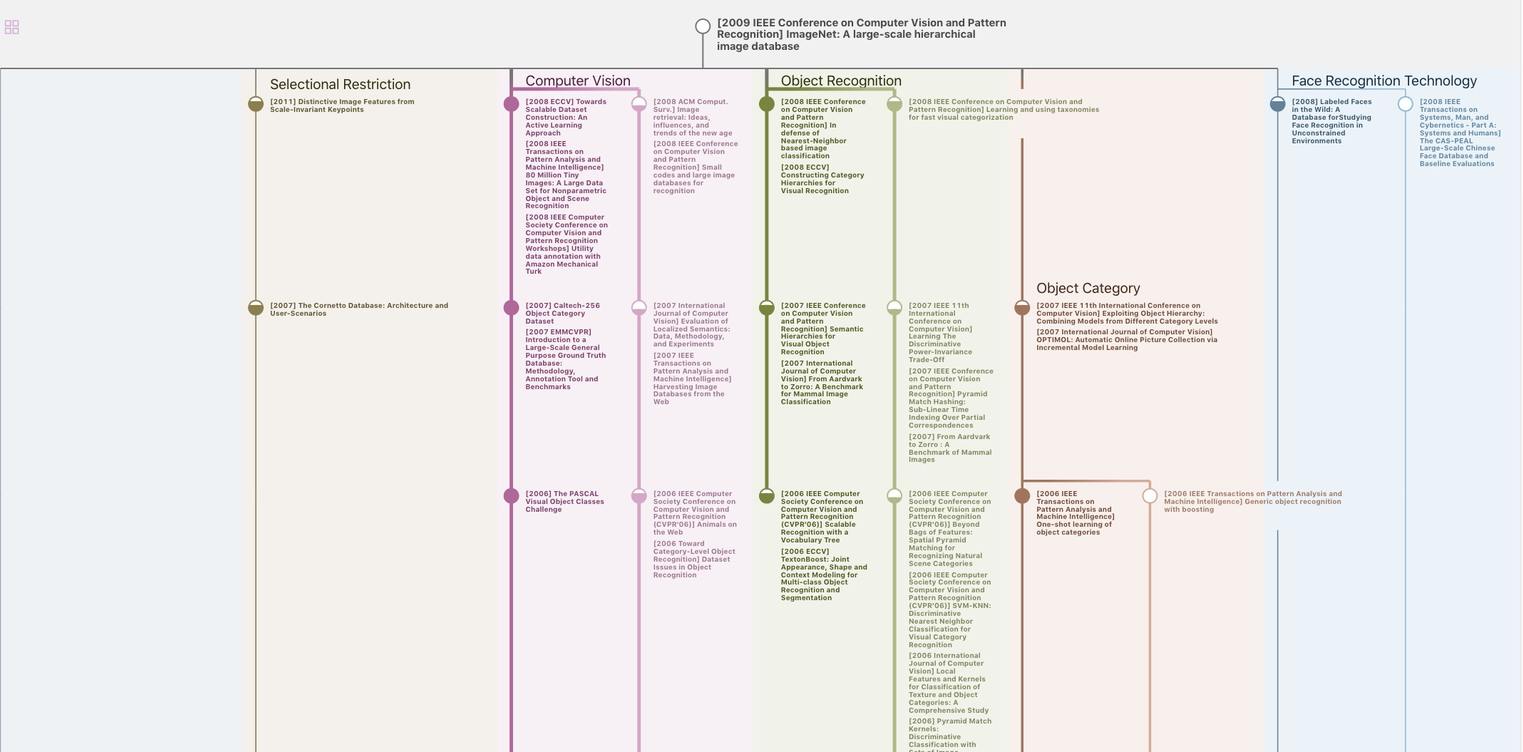
生成溯源树,研究论文发展脉络
Chat Paper
正在生成论文摘要