Approximating Marginalization with Sparse Global Priors for Sliding Window SLAM-Graphs
2019 Third IEEE International Conference on Robotic Computing (IRC)(2019)
摘要
Most autonomous vehicles rely on some kind of map for localization or navigation. Outdated maps however are a risk to the performance of any map-based localization system applied in autonomous vehicles. It is necessary to update the used maps to ensure stable and long-term operation. We address the problem of computing landmark updates live in the vehicle, which requires efficient use of the computational resources. In particular, we employ a graph-based sliding window approach for simultaneous localization and incremental map refinement. We propose a novel method that approximates sliding window marginalization without inducing fill-in. Our method maintains the exact same sparsity pattern as without performing marginalization, but simultaneously improves the landmark estimates. The main novelty of this work is the derivation of sparse global priors that approximate dense marginalization. In comparison to state-of-the-art work, our approach utilizes global instead of local linearization points, but still minimizes linearization errors. We first approximate marginalization via Kullback-Leibler divergence and then recalculate the mean to compensate linearization errors. We evaluate our approach on simulated and real data from a prototype vehicle and compare our approach to state-of-the-art sliding window marginalization.
更多查看译文
关键词
Microsoft Windows,Optimization,Autonomous vehicles,Jacobian matrices,Robots,Navigation,Trajectory
AI 理解论文
溯源树
样例
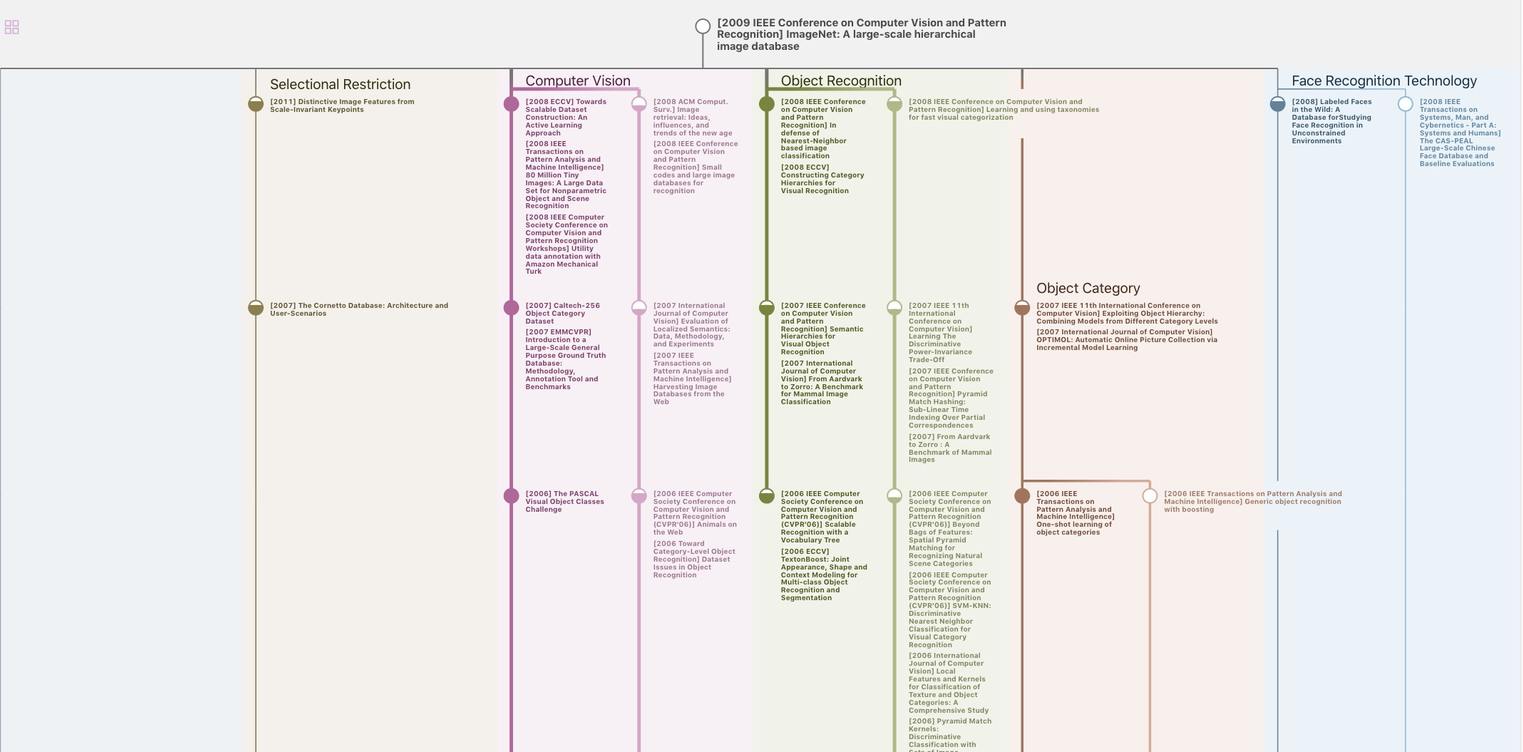
生成溯源树,研究论文发展脉络
Chat Paper
正在生成论文摘要