MARES: multitask learning algorithm for Web-scale real-time event summarization
World Wide Web(2018)
摘要
Automatic real-time summarization of massive document streams on the Web has become an important tool for quickly transforming theoverwhelming documents into a novel, comprehensive and concise overview of an event for users. Significant progresses have been made in static text summarization. However, most previous work does not consider the temporal features of the document streams which are valuable in real-time event summarization. In this paper, we propose a novel M ultitask learning A lgorithm for Web-scale R eal-time E vent S ummarization ( MARES ), which leverages the benefits of supervised deep neural networks as well as a reinforcement learning algorithm to strengthen the representation learning of documents. Specifically, MARES consists two key components: (i) A relevance prediction classifier, in which a hierarchical LSTM model is used to learn the representations of queries and documents; (ii) A document filtering model learns to maximize the long-term rewards with reinforcement learning algorithm, working on a shared document encoding layer with the relevance prediction component. To verify the effectiveness of the proposed model, extensive experiments are conducted on two real-life document stream datasets: TREC Real-Time Summarization Track data and TREC Temporal Summarization Track data. The experimental results demonstrate that our model can achieve significantly better results than the state-of-the-art baseline methods.
更多查看译文
关键词
Multitask learning,Real-time event summarization,Relevance prediction,Document filtering
AI 理解论文
溯源树
样例
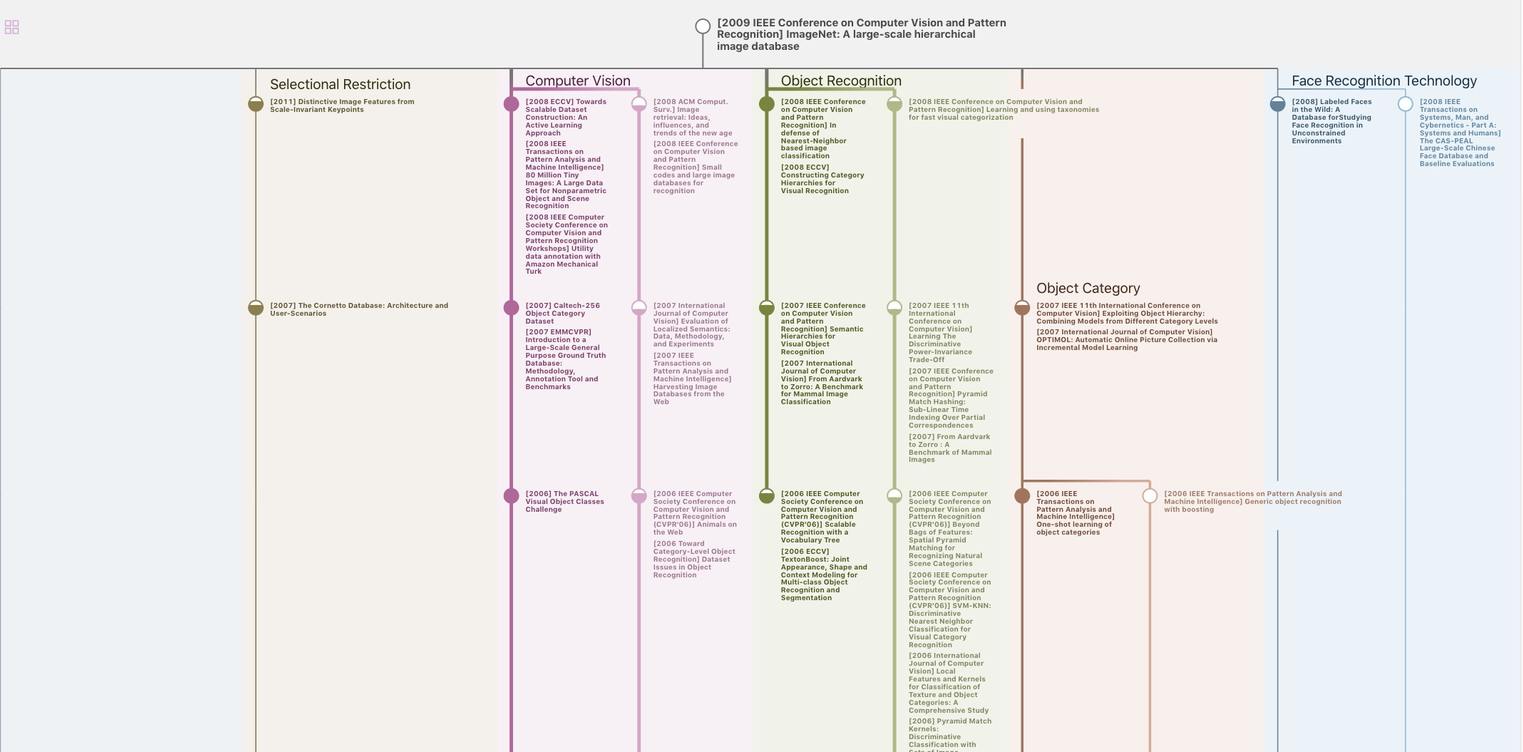
生成溯源树,研究论文发展脉络
Chat Paper
正在生成论文摘要