Cross-Modality Feature Learning via Convolutional Autoencoder
ACM Transactions on Multimedia Computing, Communications, and Applications (TOMM)(2019)
摘要
Learning robust and representative features across multiple modalities has been a fundamental problem in machine learning and multimedia fields. In this article, we propose a novel MUltimodal Convolutional AutoEncoder (MUCAE) approach to learn representative features from visual and textual modalities. For each modality, we integrate the convolutional operation into an autoencoder framework to learn a joint representation from the original image and text content. We optimize the convolutional autoencoders of different modalities jointly by exploiting the correlation between the hidden representations from the convolutional autoencoders, in particular by minimizing both the reconstructing error of each modality and the correlation divergence between the hidden feature of different modalities. Compared to the conventional solutions relying on hand-crafted features, the proposed MUCAE approach encodes features from image pixels and text characters directly and produces more representative and robust features. We evaluate MUCAE on cross-media retrieval as well as unimodal classification tasks over real-world large-scale multimedia databases. Experimental results have shown that MUCAE performs better than the state-of-the-arts methods.
更多查看译文
关键词
Cross modality, convolutional autoencoder, feature learning
AI 理解论文
溯源树
样例
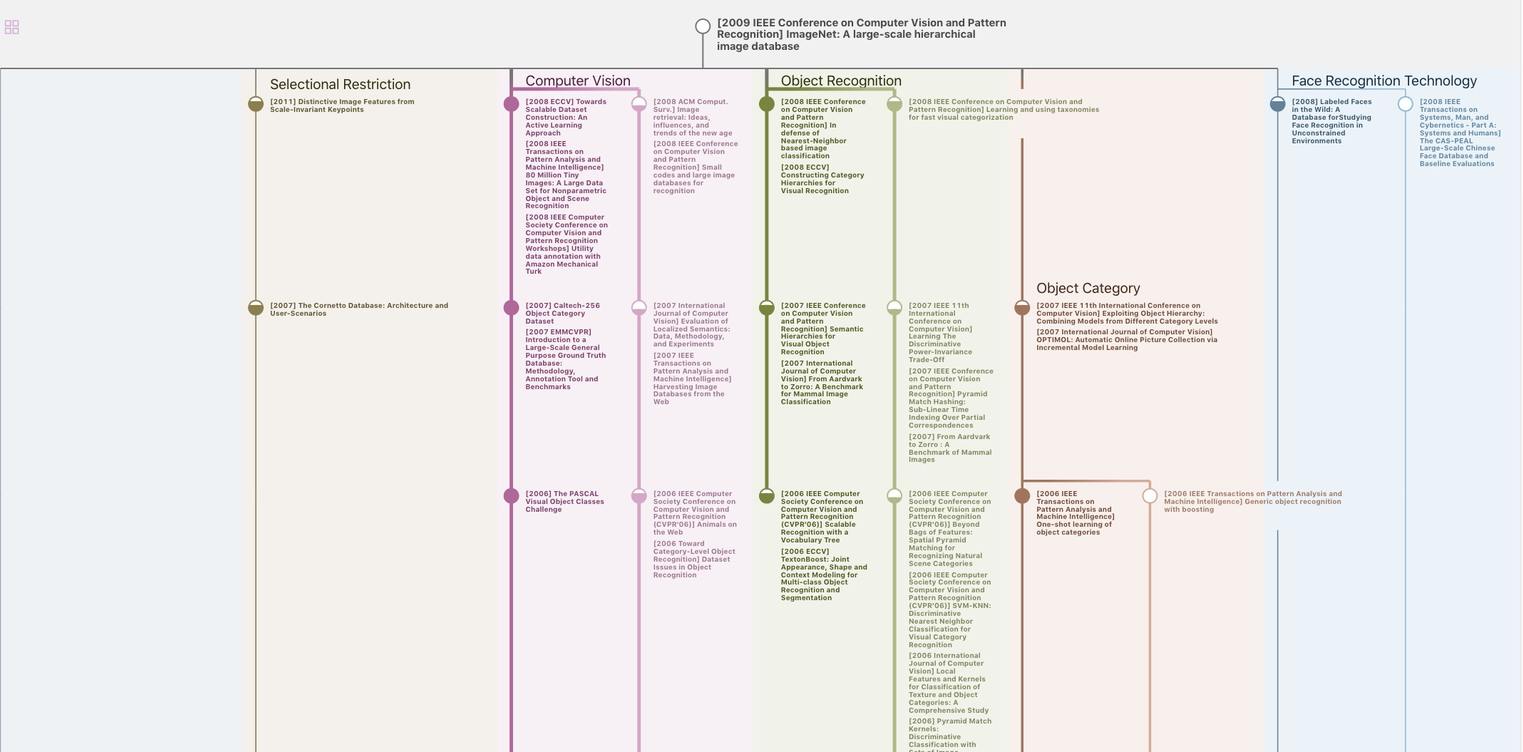
生成溯源树,研究论文发展脉络
Chat Paper
正在生成论文摘要