Mid-Level Visual Representations Improve Generalization and Sample Efficiency for Learning Active Tasks.
arXiv: Computer Vision and Pattern Recognition(2018)
摘要
One of the ultimate promises of computer is to help robotic agents perform active tasks, like delivering packages or doing household chores. However, the conventional approach to solving vision is to define a set of offline recognition problems (e.g. object detection) and solve those first. This approach faces a challenge from the recent rise of Deep Reinforcement Learning frameworks that learn active tasks from scratch using images as input. This poses a set of fundamental questions: what is the role of computer if everything can be learned from scratch? Could intermediate tasks actually be useful for performing arbitrary downstream active tasks? show that proper use of mid-level perception confers significant advantages over training from scratch. We implement a perception module as a set of mid-level visual representations and demonstrate that learning active tasks with mid-level features is significantly more sample-efficient than scratch and able to generalize in situations where the from-scratch approach fails. However, we show that realizing these gains requires careful selection of the particular mid-level features for each downstream task. Finally, we put forth a simple and efficient perception module based on the results of our study, which can be adopted as a rather generic perception module for active frameworks.
更多查看译文
AI 理解论文
溯源树
样例
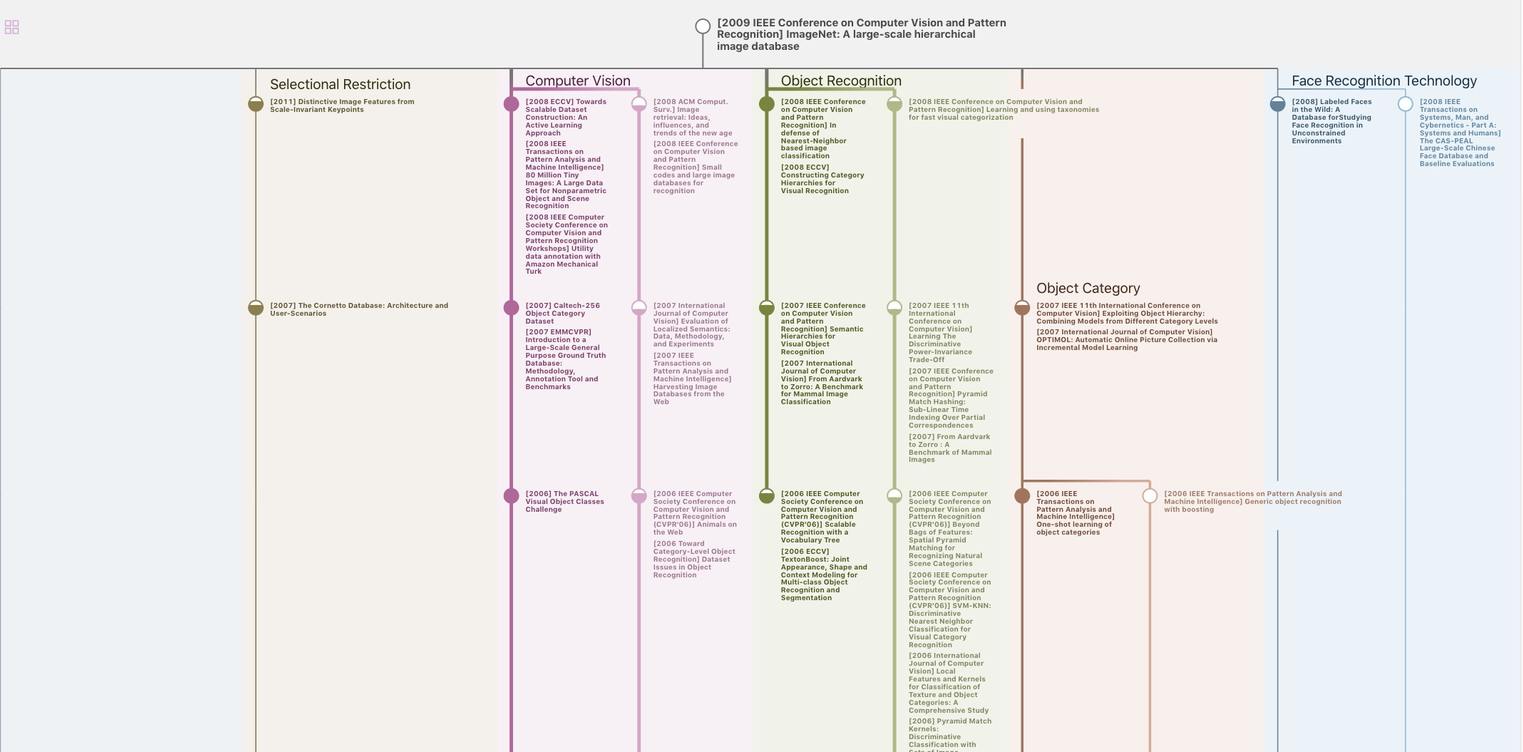
生成溯源树,研究论文发展脉络
Chat Paper
正在生成论文摘要