SC-Conv: Sparse-Complementary Convolution for Efficient Model Utilization on CNNs
2018 IEEE International Symposium on Multimedia (ISM)(2018)
摘要
We propose sparse-complementary convolution (SC-Conv) to improve model utilization of convolution neural networks (CNNs). The networks with SC-Conv achieve better accuracy than the regular convolution under similar computations and parameters. The proposed SC-Conv is paired with two deterministic sparse kernels, and one of kernels is complementary to the other one at in either spatial or channel domain or both; the deterministic sparsity increases the computational speed theoretically and practically; furthermore, by having the complementary characteristic, SC-Conv retains the same receptive field to the conventional convolution. This insightful but straightforward SC-Conv reuses of modern network architectures (ResNet and DenseNet), and at the same FLOPs and parameters, SC-Conv improves top-1 classification accuracy on ImageNet by 0.6 points for ResNet-101 and keep the same model complexity. Furthermore, SC-Conv also outperforms recent sparse networks by 1.3 points at top-1 accuracy for ImageNet, and after integrating SC-Conv with the sparse network, we further improve another 1.8 points accuracy at similar FLOPs and parameters.
更多查看译文
关键词
Deep learning,CNN,model utilization,sparse convolution
AI 理解论文
溯源树
样例
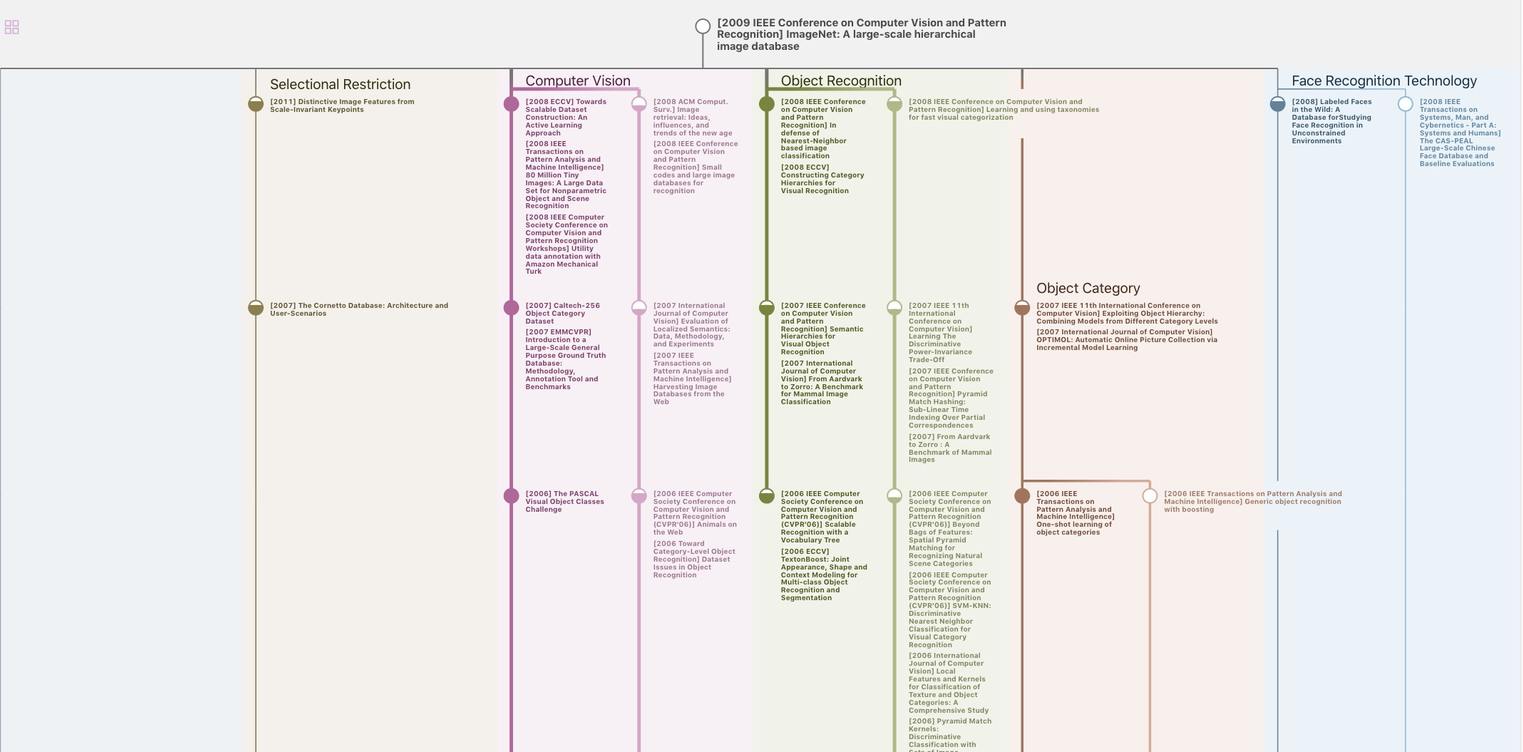
生成溯源树,研究论文发展脉络
Chat Paper
正在生成论文摘要