Building Dense Reflectance Maps Of Indoor Environments Using An Rgb-D Camera
2018 IEEE/RSJ INTERNATIONAL CONFERENCE ON INTELLIGENT ROBOTS AND SYSTEMS (IROS)(2018)
摘要
The ability to build models of the environment is an essential prerequisite for many robotic applications. In recent years, mapping of dense surface geometry using RGB-D cameras has seen extensive progress. Many approaches build colored models, typically directly using the intensity values provided by the camera. Unfortunately, these intensities are inherently affected by illumination. Therefore, the resulting maps only represent the environment for one specific lighting condition. To overcome this limitation, we propose to build reflectance maps that are invariant against changes in lighting. Our approach estimates the diffuse reflectance of a surface by recovering its radiosity and the corresponding irradiance. As imperfections in this process can significantly degrade the reflectance estimate, we remove outliers in the high dynamic range radiosity estimation and propose a method to refine the reflectance estimate. Our system implements the whole pipeline for offline reconstruction of dense reflectance maps including the segmentation of light emitters in the scene. We demonstrate the applicability of our approach in real-world experiments under varying lighting conditions.
更多查看译文
关键词
lighting conditions,light emitters,high dynamic range radiosity estimation,reflectance estimate,diffuse reflectance,specific lighting condition,colored models,extensive progress,RGB-D cameras,dense surface geometry,robotic applications,indoor environments,building dense reflectance maps
AI 理解论文
溯源树
样例
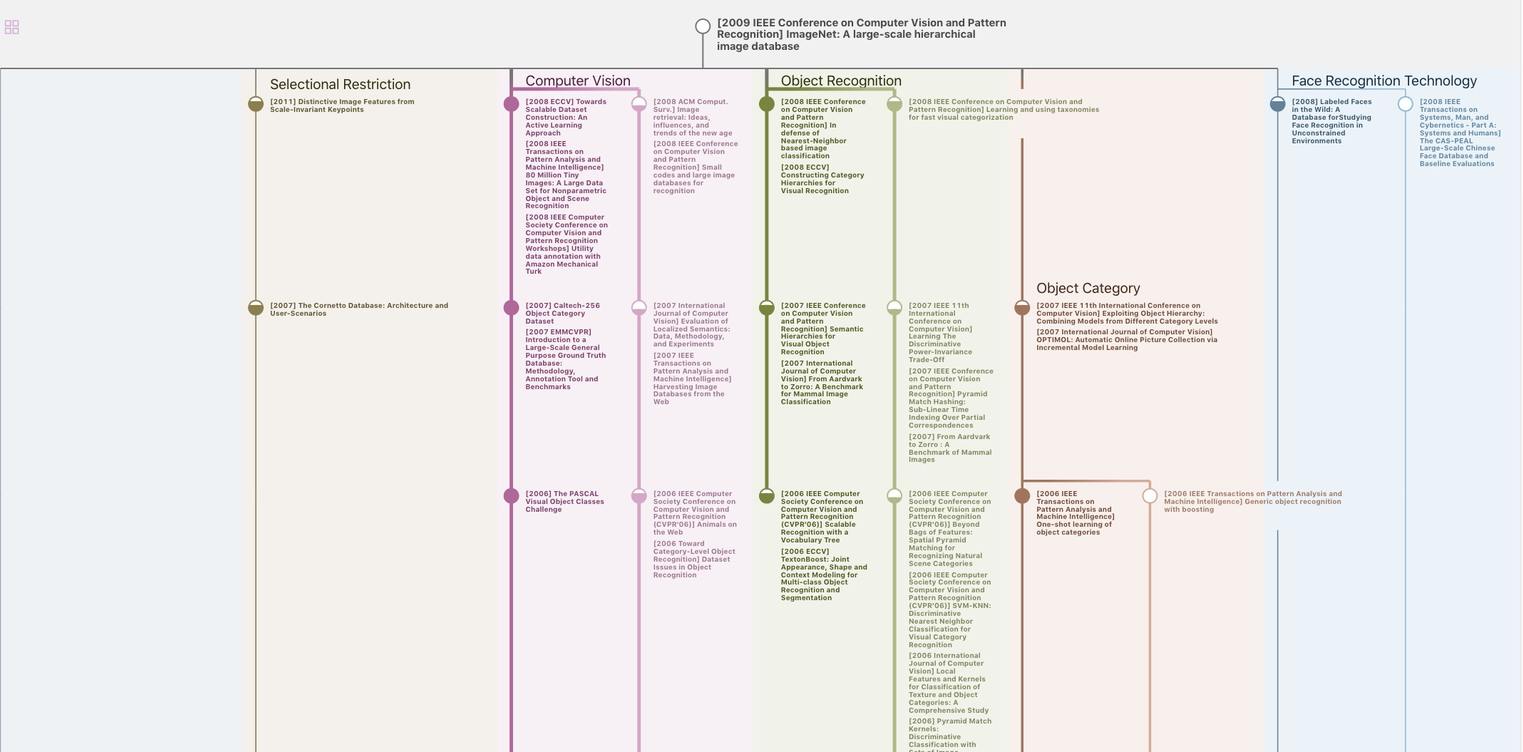
生成溯源树,研究论文发展脉络
Chat Paper
正在生成论文摘要