Cross-Scene Suture Thread Parsing For Robot Assisted Anastomosis Based On Joint Feature Learning
2018 IEEE/RSJ INTERNATIONAL CONFERENCE ON INTELLIGENT ROBOTS AND SYSTEMS (IROS)(2018)
摘要
Task autonomy is an important consideration for the development of future surgical robots. For robot-assisted anastomosis, suture thread detection is a prerequisite for subsequent robot manipulation. Previous works on automatic thread detection are focused on the learning of the models with specific surgical settings that are poorly generalisable to generic settings. In this paper, we propose a joint feature learning framework that caters for the foreground and background adaptation for surgical suture thread detection. The proposed method is developed in the context of semi-supervised and unsupervised domain adaptation, leveraging the labelled training data from the source domain to learn the detection model for unlabelled or partially labelled target domain, which can also be from different types of threads or organs. Based on adversarial learning, we further preserve the semantic identity and introduce curriculum adaptation to generate synthetic data. Experiments on four domain adaptation tasks for suture thread detection demonstrate the strength of the proposed method being able to generate good quality synthetic data and transfer between specific domains with limited or even no labelled data of the target domain.
更多查看译文
关键词
joint feature learning framework,background adaptation,surgical suture thread detection,unsupervised domain adaptation,labelled training data,partially labelled target domain,organs,adversarial learning,cross-scene suture thread parsing,task autonomy,robot-assisted anastomosis,automatic thread detection,surgical robots,robot manipulation,surgical settings,foreground adaptation,semisupervised domain adaptation,detection model learning,semantic identity
AI 理解论文
溯源树
样例
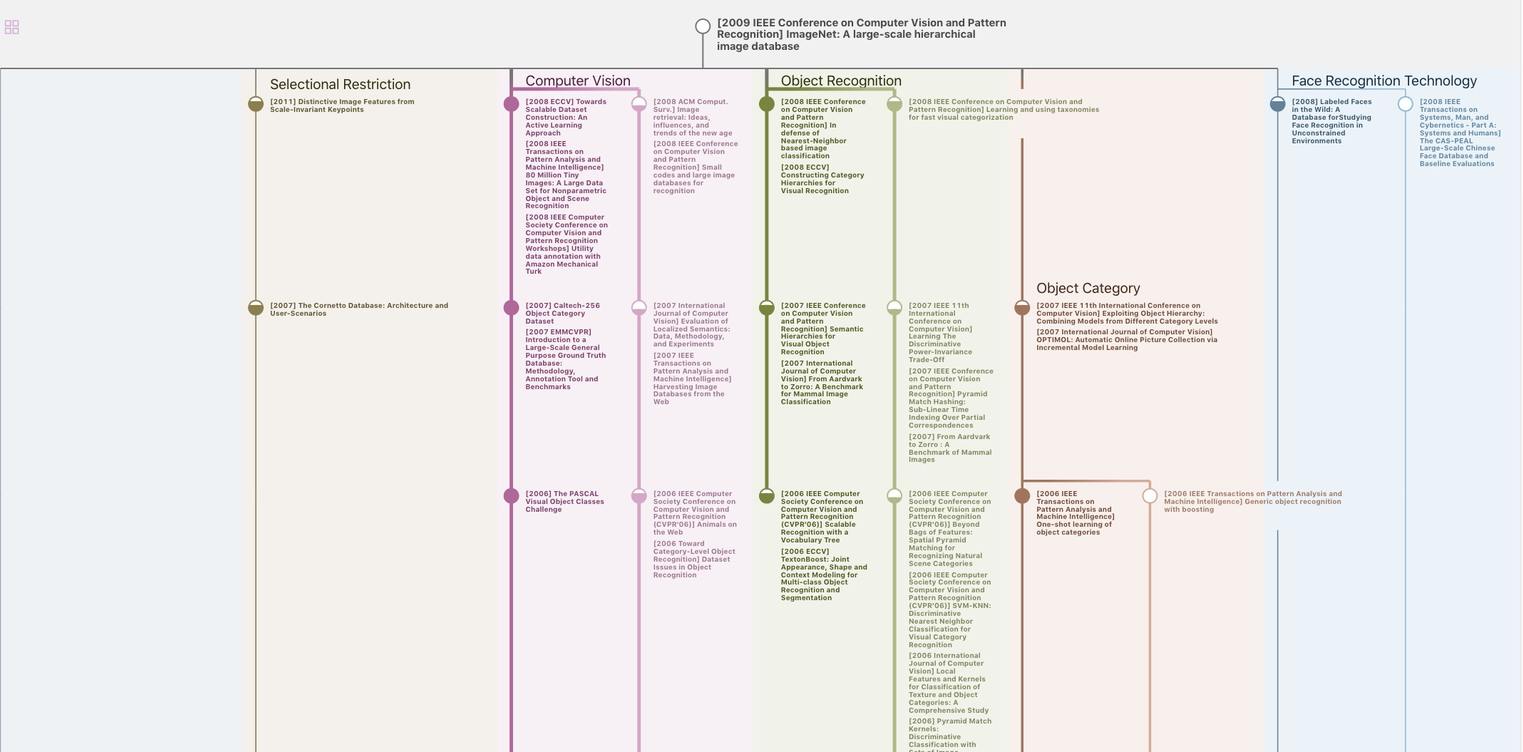
生成溯源树,研究论文发展脉络
Chat Paper
正在生成论文摘要