Fast Monte-Carlo Localization on Aerial Vehicles using Approximate Continuous Belief Representations
2018 IEEE/CVF CONFERENCE ON COMPUTER VISION AND PATTERN RECOGNITION (CVPR)(2018)
摘要
Size, weight, and power constrained platforms impose constraints on computational resources that introduce unique challenges in implementing localization algorithms. We present a framework to perform fast localization on such platforms enabled by the compressive capabilities of Gaussian Mixture Model representations of point cloud data. Given raw structural data from a depth sensor and pitch and roll estimates from an on-board attitude reference system, a multi-hypothesis particle filter localizes the vehicle by exploiting the likelihood of the data originating from the mixture model. We demonstrate analysis of this likelihood in the vicinity of the ground truth pose and detail its utilization in a particle filter-based vehicle localization strategy, and later present results of real-time implementations on a desktop system and an off-the-shelf embedded platform that outperform localization results from running a state-of-the-art algorithm on the same environment.
更多查看译文
关键词
aerial vehicles,approximate continuous belief representations,pitch estimates,raw structural data,Gaussian mixture model representations,fast Monte-carlo localization,off-the-shelf embedded platform,desktop system,particle filter-based vehicle localization strategy,ground truth pose,multihypothesis particle filter,on-board attitude reference system,roll estimates,depth sensor,point cloud data,compressive capabilities,computational resources,power constrained platforms
AI 理解论文
溯源树
样例
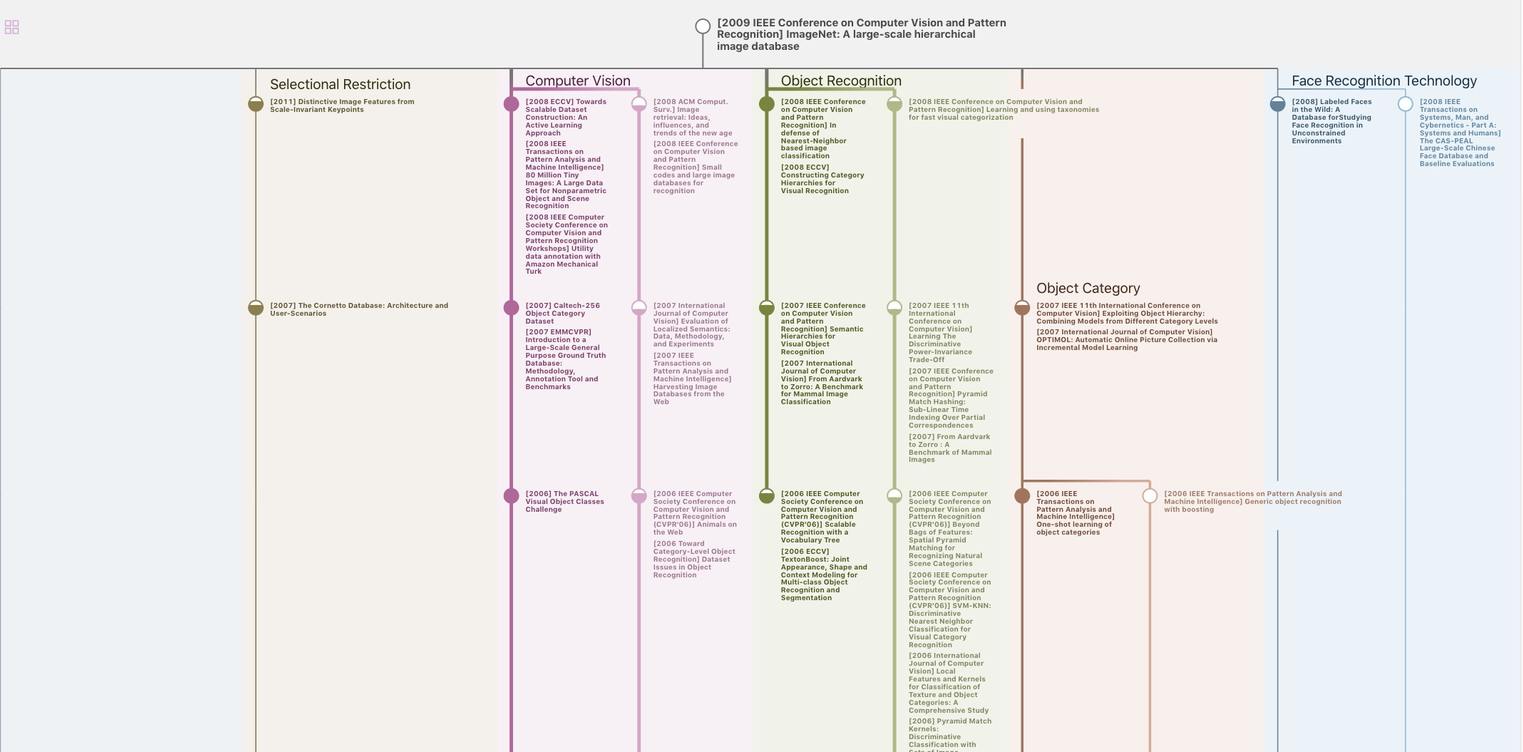
生成溯源树,研究论文发展脉络
Chat Paper
正在生成论文摘要