Beyond A/B Testing: Sequential Randomization for Developing Interventions in Scaled Digital Learning Environments.
Proceedings of the 9th International Conference on Learning Analytics & Knowledge(2019)
摘要
Randomized experiments ensure robust causal inference that is critical to effective learning analytics research and practice. However, traditional randomized experiments, like A/B tests, are limiting in large scale digital learning environments. While traditional experiments can accurately compare two treatment options, they are less able to inform how to adapt interventions to continually meet learners' diverse needs. In this work, we introduce a trial design for developing adaptive interventions in scaled digital learning environments -- the sequential randomized trial (SRT). With the goal of improving learner experience and developing interventions that benefit all learners at all times, SRTs inform how to sequence, time, and personalize interventions. In this paper, we provide an overview of SRTs, and we illustrate the advantages they hold compared to traditional experiments. We describe a novel SRT run in a large scale data science MOOC. The trial results contextualize how learner engagement can be addressed through culturally-targeted reminder emails. We also provide practical advice for researchers who aim to run their own SRTs to develop adaptive interventions in scaled digital learning environments.
更多查看译文
关键词
Experimental design, MOOCs, sequential randomization
AI 理解论文
溯源树
样例
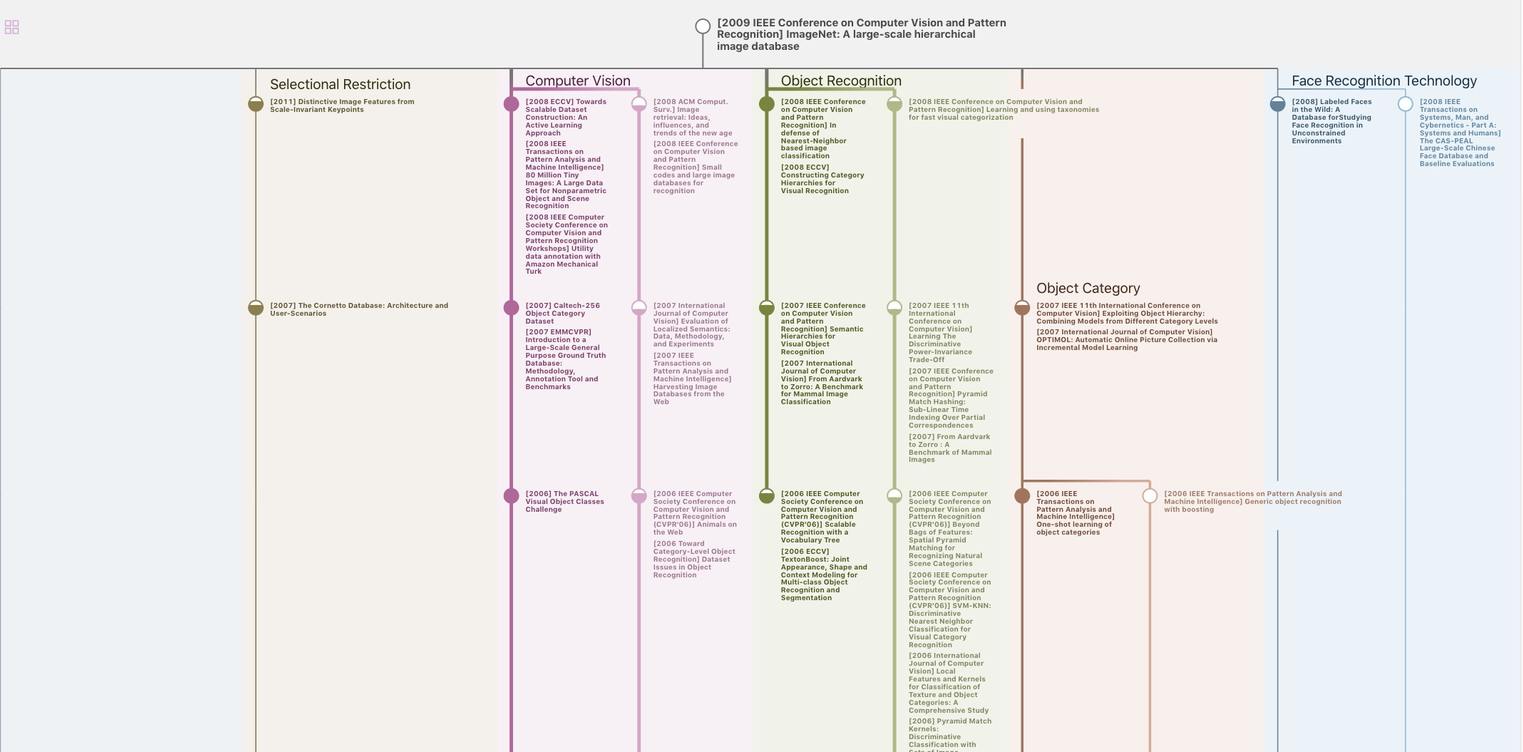
生成溯源树,研究论文发展脉络
Chat Paper
正在生成论文摘要