Learning a Local Feature Descriptor for 3D LiDAR Scans
2018 IEEE/RSJ International Conference on Intelligent Robots and Systems (IROS)(2018)
摘要
Robust data association is necessary for virtually every SLAM system and finding corresponding points is typically a preprocessing step for scan alignment algorithms. Traditionally, handcrafted feature descriptors were used for these problems but recently learned descriptors have been shown to perform more robustly. In this work, we propose a local feature descriptor for 3D LiDAR scans. The descriptor is learned using a Convolutional Neural Network (CNN). Our proposed architecture consists of a Siamese network for learning a feature descriptor and a metric learning network for matching the descriptors. We also present a method for estimating local surface patches and obtaining ground-truth correspondences. In extensive experiments, we compare our learned feature descriptor with existing 3D local descriptors and report highly competitive results for multiple experiments in terms of matching accuracy and computation time. \end{abstract}
更多查看译文
关键词
learned feature descriptor,3D local descriptors,local feature descriptor,3D LiDAR scans,robust data association,scan alignment algorithms,handcrafted feature descriptors,metric learning network,local surface patches,convolutional neural network,ground-truth correspondences,SLAM system,CNN,Siamese network
AI 理解论文
溯源树
样例
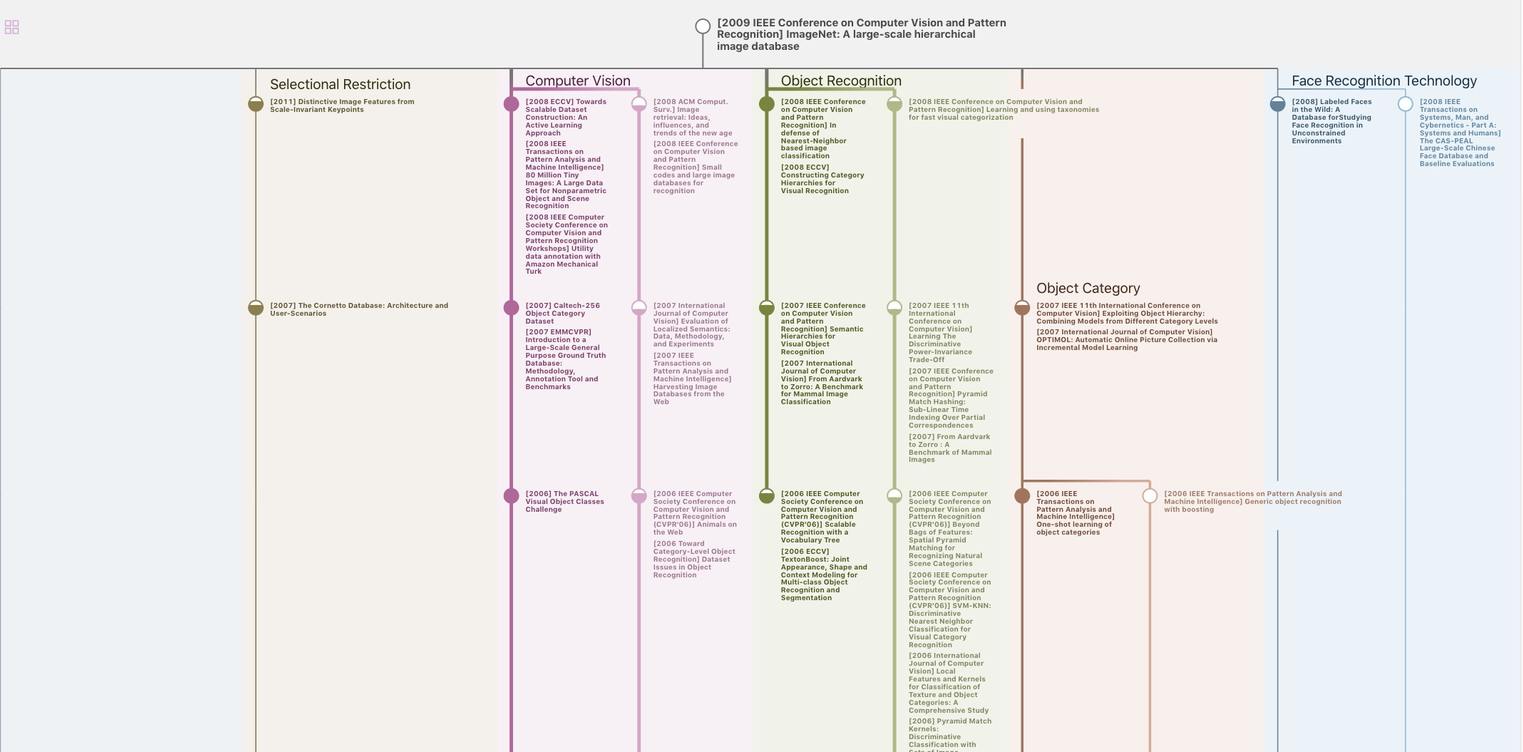
生成溯源树,研究论文发展脉络
Chat Paper
正在生成论文摘要