Sharp Analysis of Learning with Discrete Losses
HAL (Le Centre pour la Communication Scientifique Directe)(2018)
摘要
The problem of devising learning strategies for discrete losses (e.g., multilabeling, ranking) is currently addressed with methods and theoretical analyses ad-hoc for each loss. In this paper we study a least-squares framework to systematically design learning algorithms for discrete losses, with quantitative characterizations in terms of statistical and computational complexity. In particular we improve existing results by providing explicit dependence on the number of labels for a wide class of losses and faster learning rates in conditions of low-noise. Theoretical results are complemented with experiments on real datasets, showing the effectiveness of the proposed general approach.
更多查看译文
关键词
discrete losses,learning,analysis
AI 理解论文
溯源树
样例
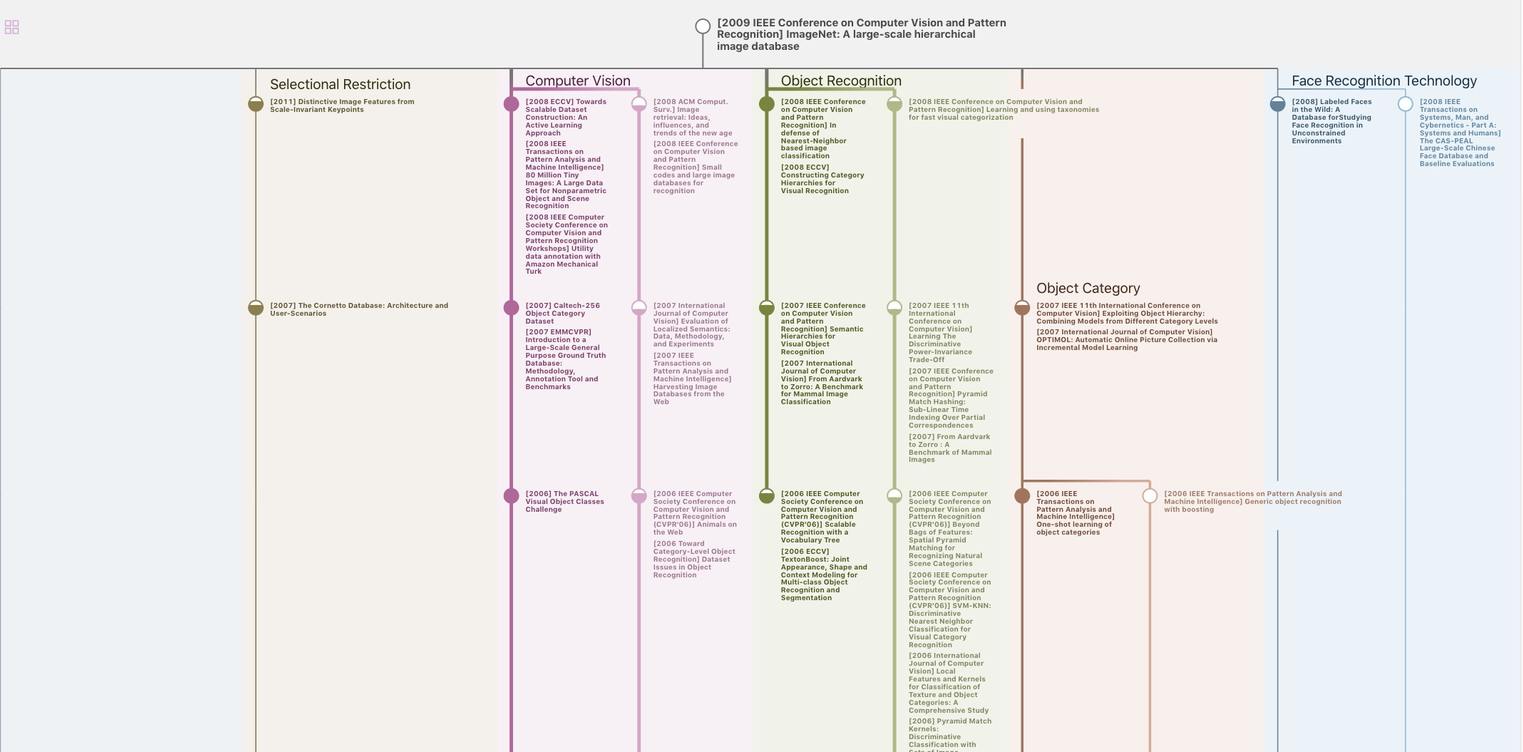
生成溯源树,研究论文发展脉络
Chat Paper
正在生成论文摘要