Reinforced Temporal Attention and Split-Rate Transfer for Depth-Based Person Re-Identification
COMPUTER VISION - ECCV 2018, PT V(2018)
摘要
We address the problem of person re-identification from commodity depth sensors. One challenge for depth-based recognition is data scarcity. Our first contribution addresses this problem by introducing split-rate RGB-to-Depth transfer, which leverages large RGB datasets more effectively than popular fine-tuning approaches. Our transfer scheme is based on the observation that the model parameters at the bottom layers of a deep convolutional neural network can be directly shared between RGB and depth data while the remaining layers need to be fine-tuned rapidly. Our second contribution enhances re-identification for video by implementing temporal attention as a Bernoulli-Sigmoid unit acting upon frame-level features. Since this unit is stochastic, the temporal attention parameters are trained using reinforcement learning. Extensive experiments validate the accuracy of our method in person re-identification from depth sequences. Finally, in a scenario where subjects wear unseen clothes, we show large performance gains compared to a state-of-the-art model which relies on RGB data.
更多查看译文
关键词
Person re-identification from depth, Reinforced temporal attention, Split-rate transfer
AI 理解论文
溯源树
样例
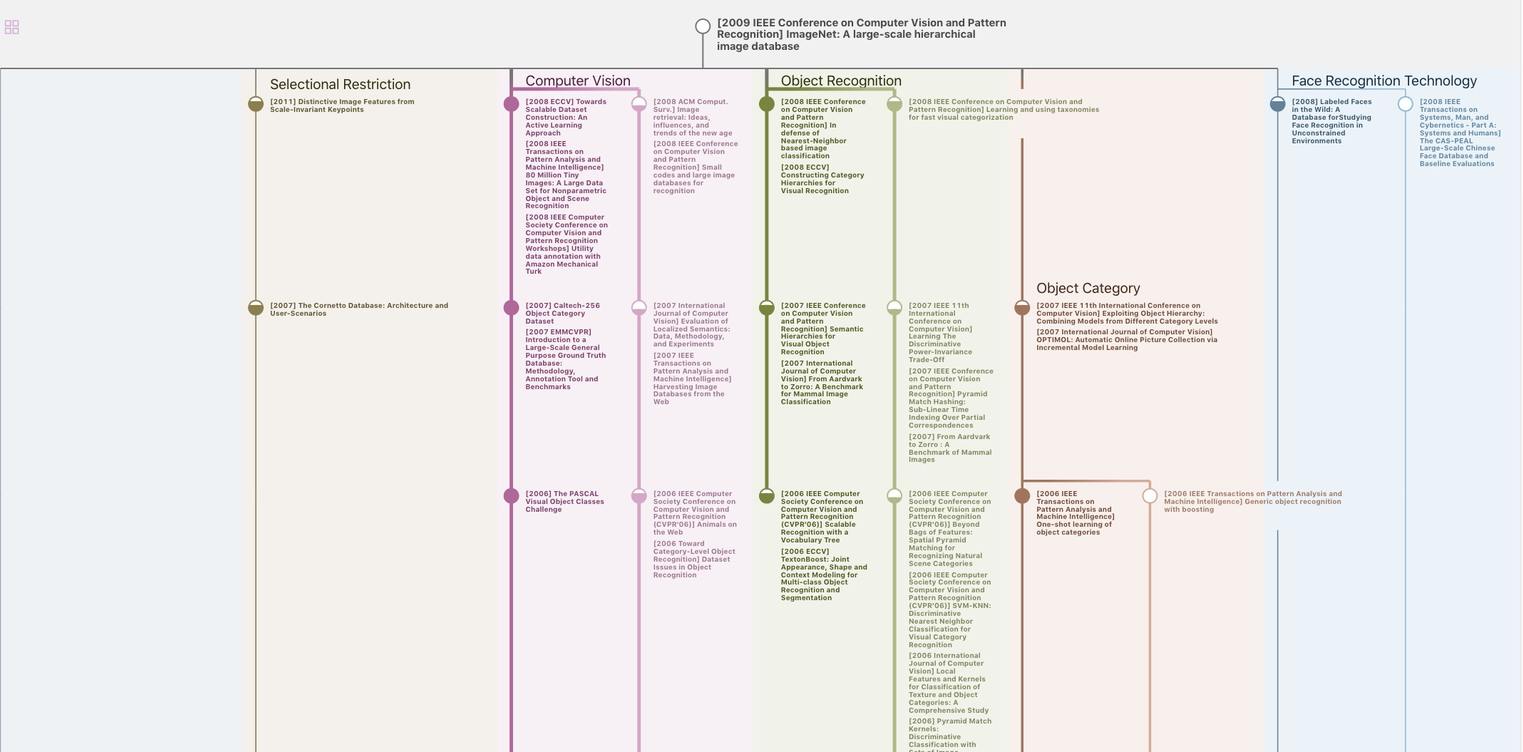
生成溯源树,研究论文发展脉络
Chat Paper
正在生成论文摘要