The Effect of Network Width on the Performance of Large-batch Training
NeurIPS(2018)
摘要
Distributed implementations of mini-batch stochastic gradient descent (SGD) suffer from communication overheads, attributed to the high frequency of gradient updates inherent in small-batch training. Training with large batches can reduce these overheads; however it besets the convergence of the algorithm and the generalization performance. In this work, we take a first step towards analyzing how the structure (width and depth) of a neural network affects the performance of large-batch training. We present new theoretical results which suggest that-for a fixed number of parameters-wider networks are more amenable to fast large-batch training compared to deeper ones. We provide extensive experiments on residual and fully-connected neural networks which suggest that wider networks can be trained using larger batches without incurring a convergence slow-down, unlike their deeper variants.
更多查看译文
关键词
neural networks,stochastic gradient descent,neural network,high frequency,first step
AI 理解论文
溯源树
样例
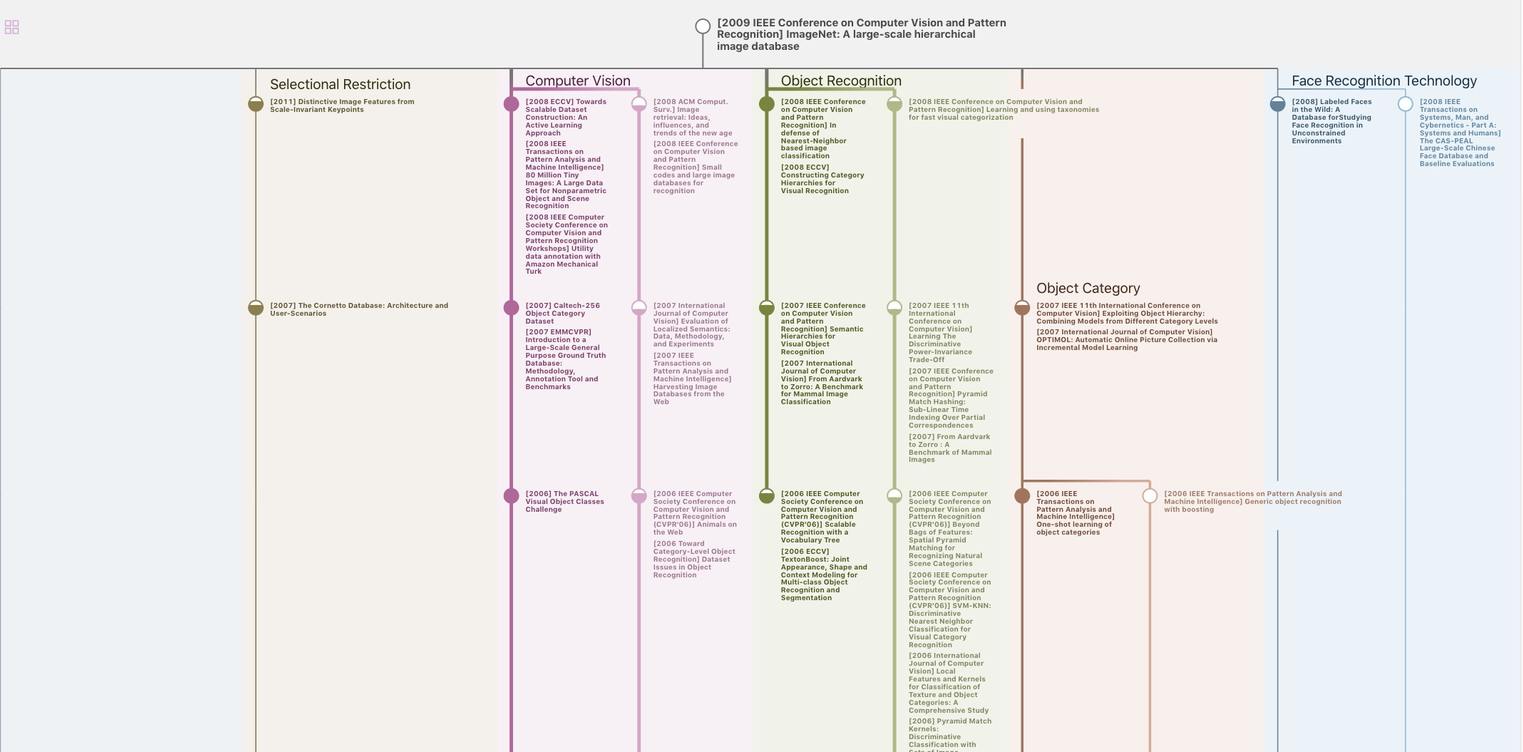
生成溯源树,研究论文发展脉络
Chat Paper
正在生成论文摘要