Deep Person Detection in Two-Dimensional Range Data
IEEE ROBOTICS AND AUTOMATION LETTERS(2018)
摘要
Detecting humans is a key skill for mobile robots and intelligent vehicles in a large variety of applications. Although the problem is well studied for certain sensory modalities such as image data, few works exist that address this detection task using two-dimensional (2-D) range data. However, a widespread sensory setup for many mobile robots in service and domestic applications contains a horizontally mounted 2-D laser scanner. Detecting people from 2-D range data is challenging due to the speed and dynamics of human leg motion and the high levels of occlusion and self-occlusion particularly in crowds of people. While previous approaches mostly relied on handcrafted features, we recently developed the deep learning based wheelchair and walker detector distance robust wheelchair/walker (DROW). In this letter, we show the generalization to people, including small modifications that significantly boost DROW's performance. Additionally, by providing a small, fully online temporal window in our network, we further boost our score. We extend the DROW dataset with person annotations, making this the largest dataset of person annotations in 2-D range data, recorded during several days in a real-world environment with high diversity. Extensive experiments with three current baseline methods indicate it is a challenging dataset, on which our improved DROW detector beats the current state-of-the-art.
更多查看译文
关键词
Deep learning in robotics and automation human detection and tracking recognition
AI 理解论文
溯源树
样例
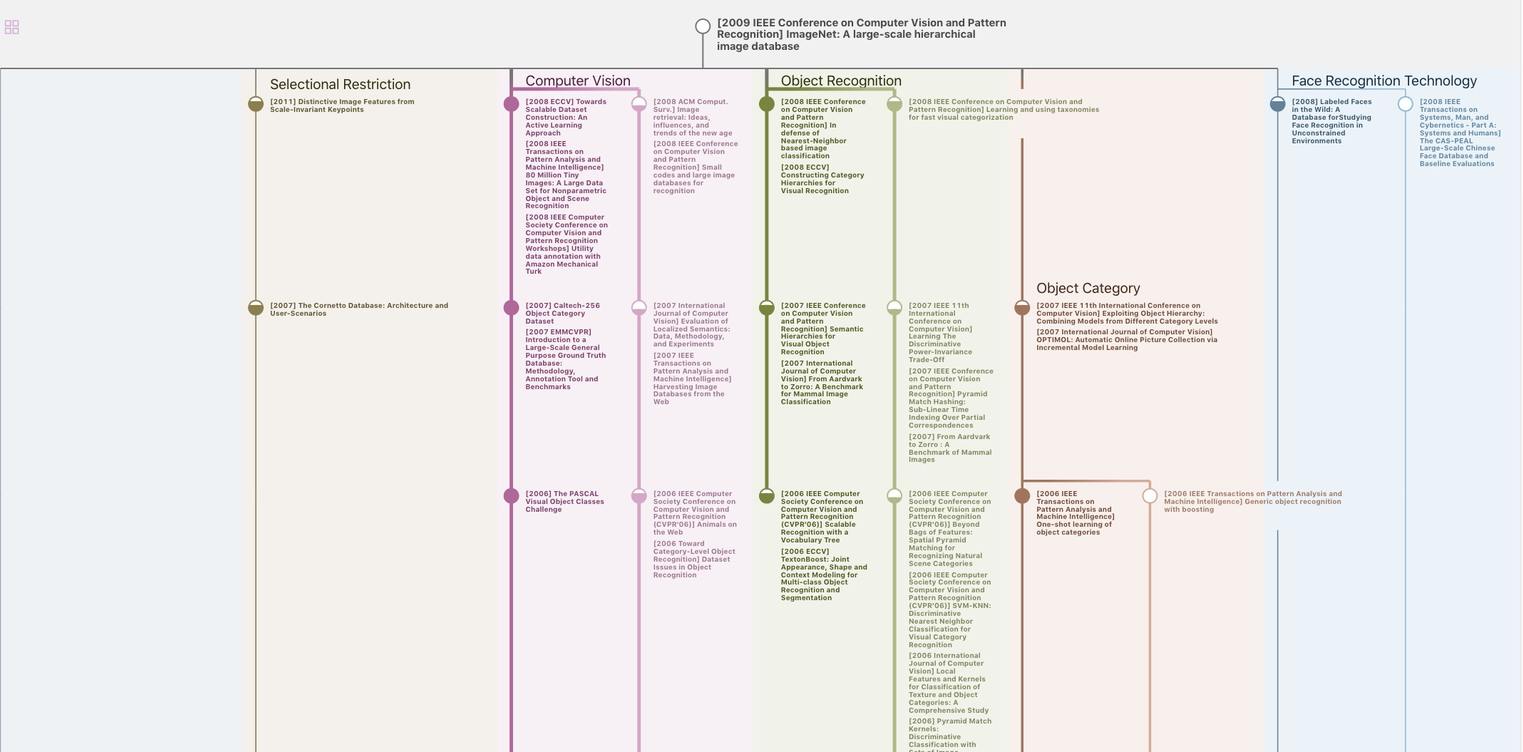
生成溯源树,研究论文发展脉络
Chat Paper
正在生成论文摘要