Rlt: Residual-Loop Training In Collaborative Filtering For Combining Factorization And Global-Local Neighborhood
WEB SERVICES - ICWS 2018(2018)
摘要
Collaborative filtering (CF) is an important recommendation problem focusing on predicting users' future preferences by exploiting their historical tastes. One typical training paradigm for this problem is called residual training (RT), which is usually built on two basic components of factorization- and local neighborhood-based methods in a sequential manner. RT has been well recognized with the ability of achieving higher recommendation accuracy than either factorization- or neighborhood-based method. In this paper, we design a new residual training paradigm called residual-loop training (RLT), which aims to fully exploit the complementarity of factorization, global neighborhood and local neighborhood in one single algorithm. Experimental results on three public datasets show the promising results of our RLT compared with several state-of-the-art methods.
更多查看译文
关键词
Residual training, Residual-loop training, Collaborative filtering
AI 理解论文
溯源树
样例
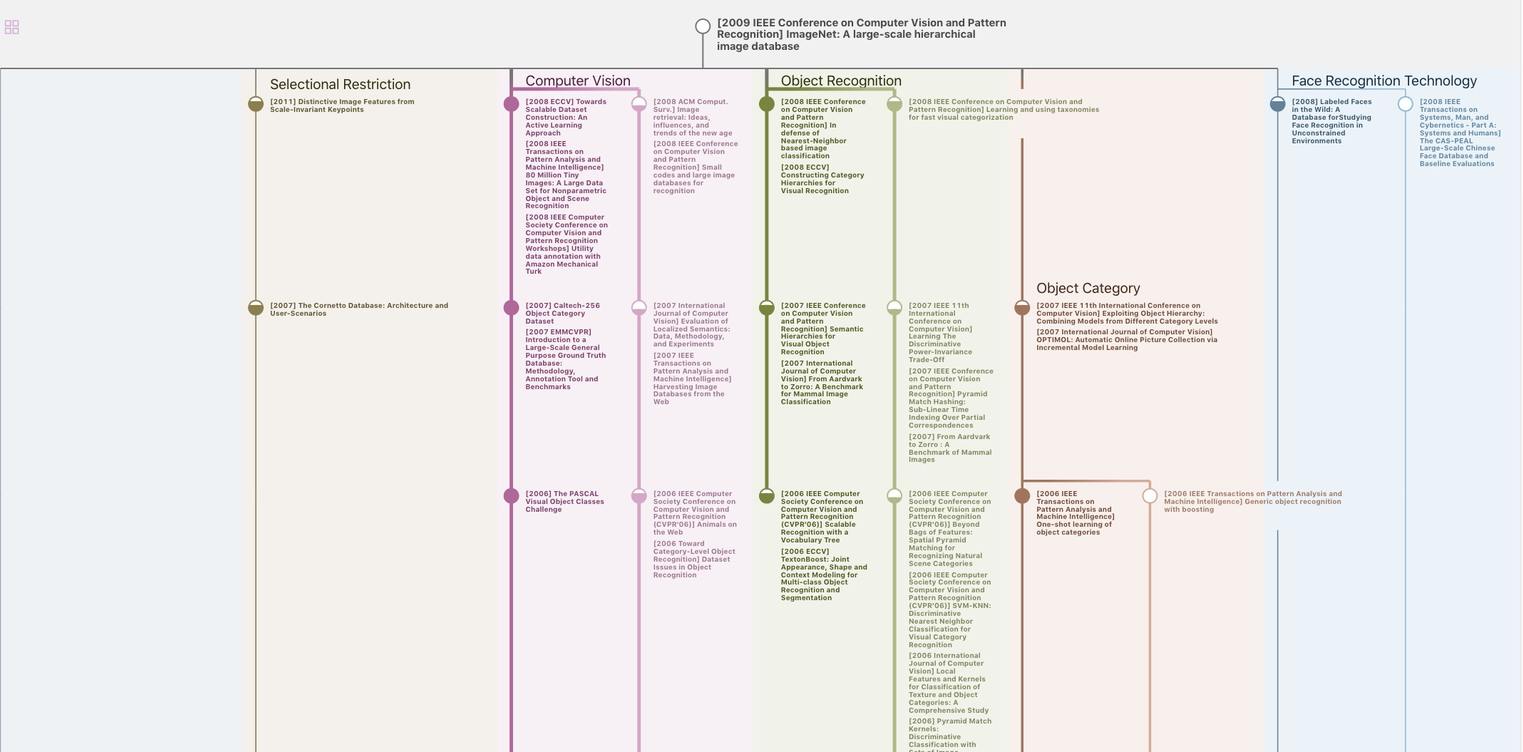
生成溯源树,研究论文发展脉络
Chat Paper
正在生成论文摘要