Drivable Road Detection Based On Dilated Fpn With Feature Aggregation
2017 IEEE 29TH INTERNATIONAL CONFERENCE ON TOOLS WITH ARTIFICIAL INTELLIGENCE (ICTAI 2017)(2017)
摘要
In the field of ADAS and self-driving car, lane and drivable road detection play an essential role in reliably accomplishing other tasks, such as objects detection. For monocular vision based semantic segmentation of lane and road, we propose a dilated feature pyramid network (FPN) with feature aggregation, called DFFA, where feature aggregation is employed to combine multi-level features enhanced with dilated convolution operations and FPN under the framework of ResNet. Experimental results validate effectiveness and efficiency of the proposed deep learning model for semantic segmentation of lane and drivable road. Our DFFA achieves the best performance both on Lane Estimation Evaluation and Behavior Evaluation tasks in KITTI-ROAD and take the second place on UU_ROAD task.
更多查看译文
关键词
dilated convolution, feature pyramid, feature aggregation
AI 理解论文
溯源树
样例
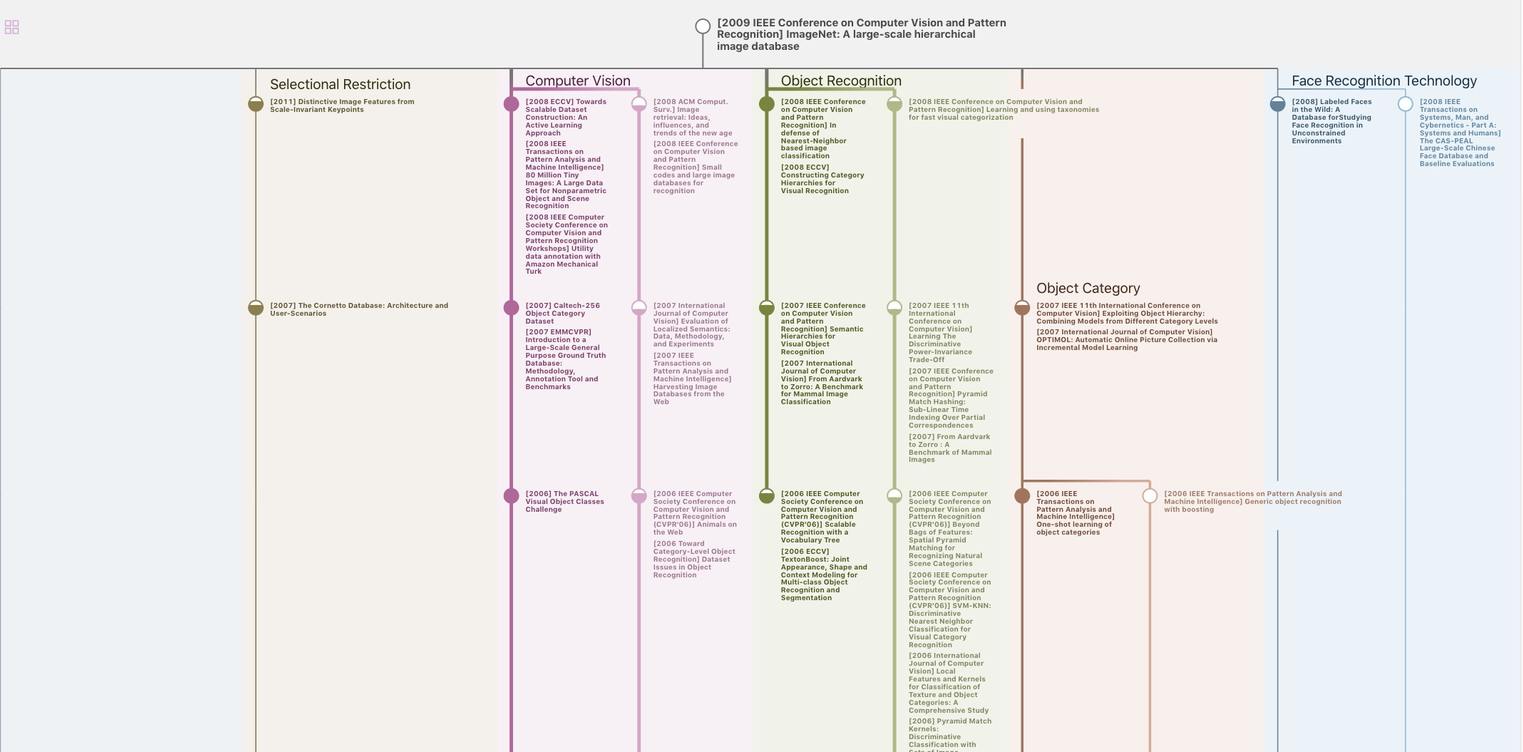
生成溯源树,研究论文发展脉络
Chat Paper
正在生成论文摘要