Sequential Context Modeling For Smart Devices By Collaborative Hidden Markov Model
2018 IEEE 4TH WORLD FORUM ON INTERNET OF THINGS (WF-IOT)(2018)
摘要
Smart devices become prevalent in our daily lives, as the advancement of mobile networks, sensor technologies and distributed computing. They easily collect rich context information (e.g., time, GPS location, Wifi info, app usage, etc.) from the device owners (i.e., the users). Modeling such sequential context data is important - it not only helps to profile the user, but also enables many context-aware applications, such as location-based services with the next location prediction and app usage predictions. However, context modeling is challenging, because the context data is heterogeneous and high-dimensional in terms of the huge number of possible contexts. In this paper, we propose a novel Collaborative Hidden Markov Model (CHMM). CHMM extends the sequential generative model HMM to a collaborative setting, such that only those users sharing similar behaviors can pool their data together to build a reliable model. We evaluate CHMM with real-world data for both next location prediction and app usage prediciton, and the results show that CHMM outperforms the baselines in both cases.
更多查看译文
关键词
sequential context,smart devices,mobile networks,sensor technologies,rich context information,GPS location,Wifi info,device owners,context-aware applications,location-based services,location prediction,app usage predictions,context modeling,context data,CHMM,sequential generative model HMM,collaborative setting,app usage prediciton,collaborative hidden Markov model
AI 理解论文
溯源树
样例
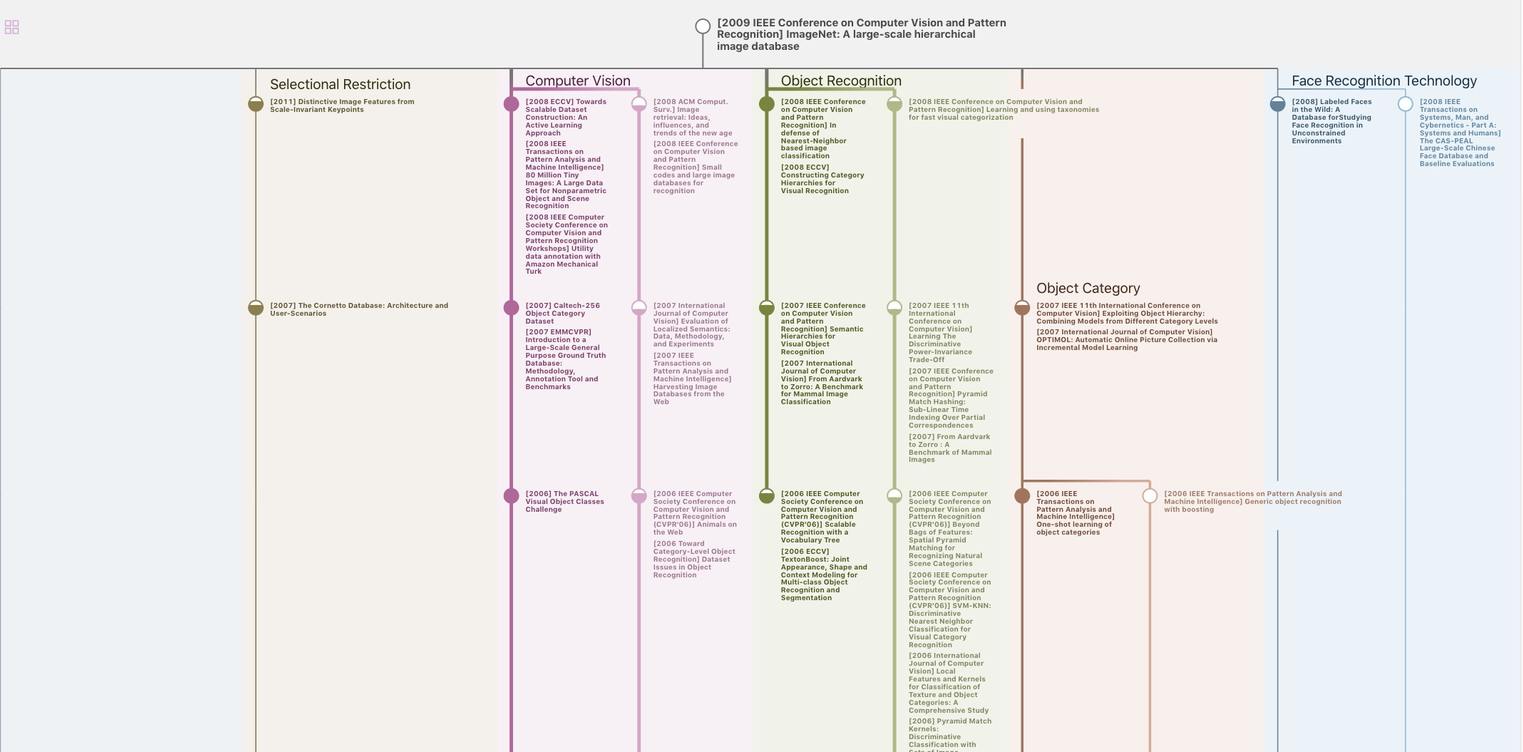
生成溯源树,研究论文发展脉络
Chat Paper
正在生成论文摘要