Am I a Baller? Basketball Performance Assessment from First-Person Videos
2017 IEEE INTERNATIONAL CONFERENCE ON COMPUTER VISION (ICCV)(2017)
摘要
This paper presents a method to assess a basketball player's performance from his/her first-person video. A key challenge lies in the fact that the evaluation metric is highly subjective and specific to a particular evaluator. We leverage the first-person camera to address this challenge. The spatiotemporal visual semantics provided by a first-person view allows us to reason about the camera wearer's actions while he/she is participating in an unscripted basketball game. Our method takes a player's first-person video and provides a player's performance measure that is specific to an evaluator's preference. To achieve this goal, we first use a convolutional LSTM network to detect atomic basketball events from first-person videos. Our network's ability to zoom-in to the salient regions addresses the issue of a severe camera wearer's head movement in first-person videos. The detected atomic events are then passed through the Gaussian mixtures to construct a highly non-linear visual spatiotemporal basketball assessment feature. Finally, we use this feature to learn a basketball assessment model from pairs of labeled first-person basketball videos, for which a basketball expert indicates, which of the two players is better. We demonstrate that despite not knowing the basketball evaluator's criterion, our model learns to accurately assess the players in real-world games. Furthermore, our model can also discover basketball events that contribute positively and negatively to a player's performance.
更多查看译文
关键词
nonlinear visual spatiotemporal basketball assessment feature,basketball assessment model,labeled first-person basketball videos,basketball expert,basketball performance assessment,basketball player,first-person camera,first-person view,unscripted basketball game,atomic basketball events,spatiotemporal visual semantics,convolutional LSTM network,salient regions,head movement,Gaussian mixtures
AI 理解论文
溯源树
样例
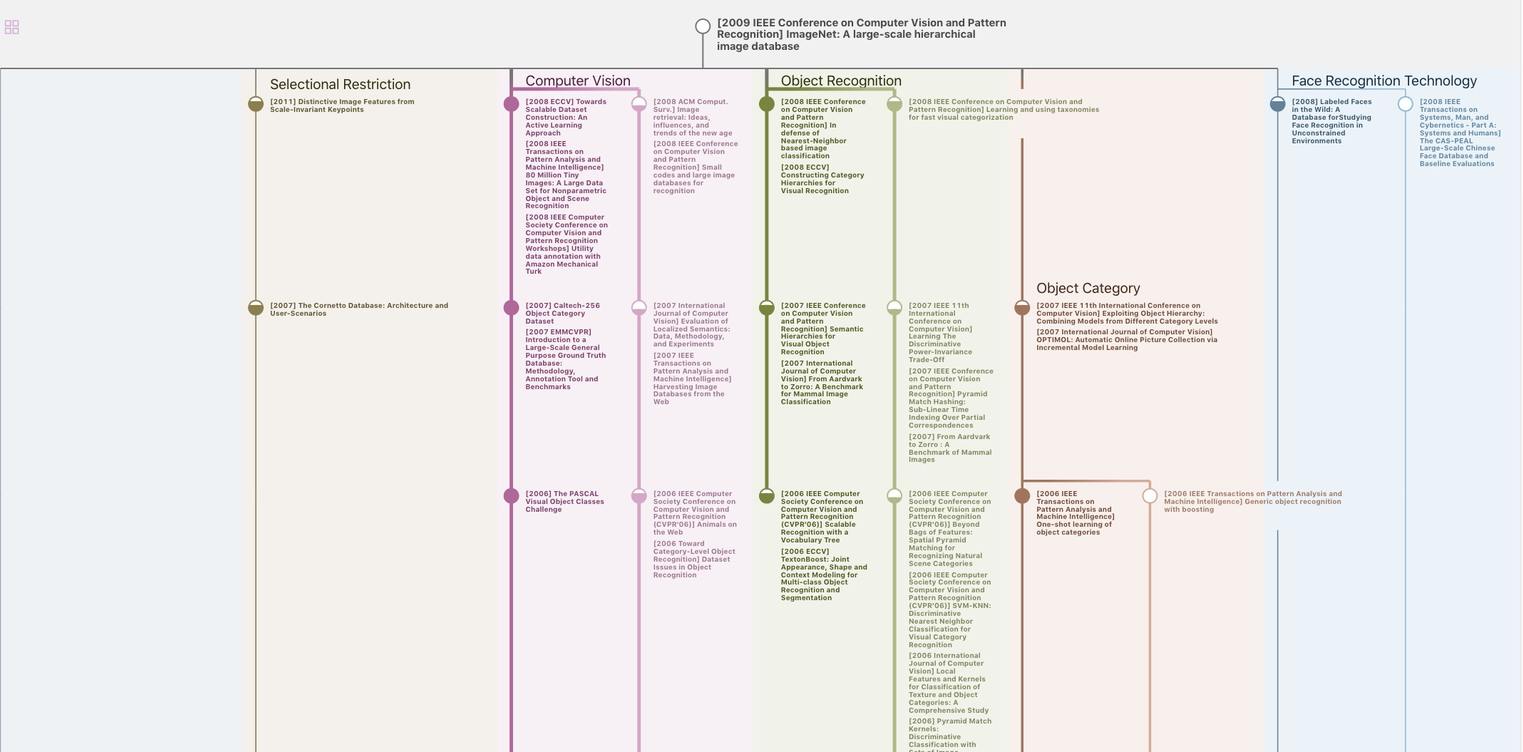
生成溯源树,研究论文发展脉络
Chat Paper
正在生成论文摘要