Stacked Sparse Auto-Encoders (SSAE) Based Electronic Nose for Chinese Liquors Classification.
SENSORS(2017)
摘要
This paper presents a stacked sparse auto-encoder (SSAE) based deep learning method for an electronic nose (e-nose) system to classify different brands of Chinese liquors. It is well known that preprocessing; feature extraction (generation and reduction) are necessary steps in traditional data-processing methods for e-noses. However, these steps are complicated and empirical because there is no uniform rule for choosing appropriate methods from many different options. The main advantage of SSAE is that it can automatically learn features from the original sensor data without the steps of preprocessing and feature extraction; which can greatly simplify data processing procedures for e-noses. To identify different brands of Chinese liquors; an SSAE based multi-layer back propagation neural network (BPNN) is constructed. Seven kinds of strong-flavor Chinese liquors were selected for a self-designed e-nose to test the performance of the proposed method. Experimental results show that the proposed method outperforms the traditional methods.
更多查看译文
关键词
stacked sparse auto-encoders,electronic nose,deep learning,Chinese liquors classification
AI 理解论文
溯源树
样例
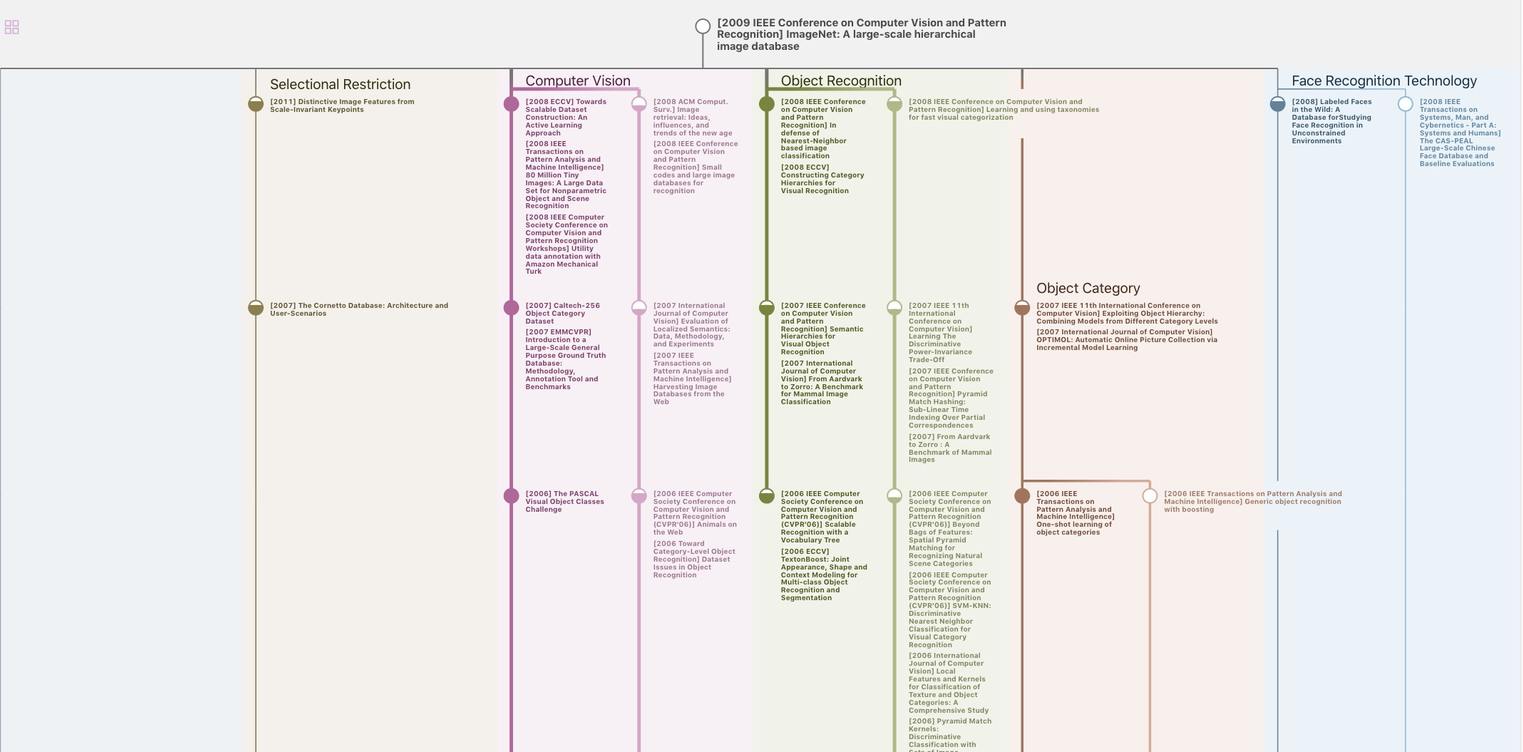
生成溯源树,研究论文发展脉络
Chat Paper
正在生成论文摘要