Spectral Lens: Explainable Diagnostics, Tools And Discoveries In Directed, Weighted Graphs
2017 17TH IEEE INTERNATIONAL CONFERENCE ON DATA MINING (ICDM)(2017)
摘要
How can we quickly explain large-scale directed and weighted graphs? We present Spectral Lens (SL) to analyze a variety of real-world networks with both negative and positive edge weights, like DBLP relationships, email communications and Bitcoin trust votings. SL offers value on three levels: (a) Diagnostics: Spectral Lens combines spectral properties from Singular Value Decomposition to create an SL-Dictionary (SLD) to enhance understanding of any directed, weighted graph. (b) Tools: the SL-Algorithm (SLA) automatically extracts the top groups of nodes with similar connectivity, finds groups of shared connectivity and detects suspicious behaviour in a graph. (c) Discoveries: Experiments on several real-world networks illustrate the effectiveness of SLA. Observations from synthetic and real-world networks reveal relations between spectral and graph properties. We show that SLA is highly scalable and linear on the size of a graph. Analyzing a graph with over 2 million edges takes less than 5 minutes. Overall, SL provides an easy-to-use tool for practitioners to explain a weighted and directed graph quickly, to understand its connectivity and identify regular and anomalous behaviors.
更多查看译文
关键词
Graph Mining,Singular Value Decomposition
AI 理解论文
溯源树
样例
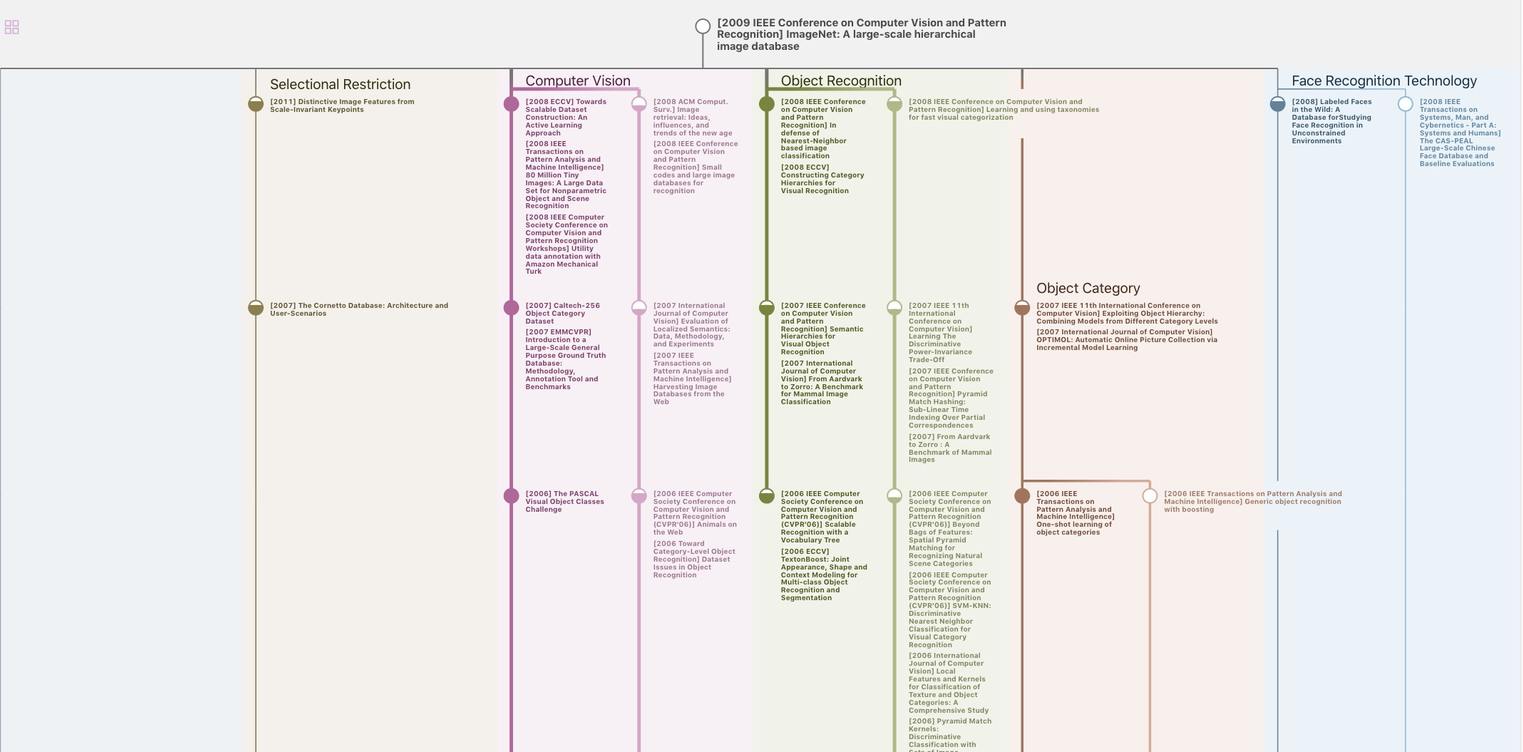
生成溯源树,研究论文发展脉络
Chat Paper
正在生成论文摘要